AZoRobotics speaks with Alexander Wong from the University of Waterloo about a new technology that could potentially help reduce malnutrition and improve overall health in long-term care homes by automatically tracking and recording how much food residents consume.
What inspired your research into creating a fully automatic imaging system for quantifying food intake?
My former Ph.D. student Kaylen Pfisterer, now a scientific associate at the University Health Network (Centre for Global eHealth Innovation), has a strong background in nutrition and part of her Ph.D. thesis was motivated by the significant gap in long-term care in reliably monitoring nutritional intake of older adults, which can greatly affect their quality of care and the residents quality of life.
I was very excited by her vision and given our lab’s expertise in artificial intelligence and imaging system design, creating a fully automatic imaging system for quantifying food intake utilizing this expertise was the natural next step.
Such a system can be game-changing by taking the guesswork and time-consuming labor out of the equation, thus providing not only greatly improved assessment of nutritional intake but also relieve caretakers from this task so that they can spend more time helping the older adults in long-term care.
Malnutrition is a multidomain problem affecting 54% of older adults in long-term care (LTC). Why is it so important to monitor nutritional intake in LTC?
Monitoring nutritional intake in LTC is very important because it is critical for maintaining the overall health and well-being of older adults, given the importance of nutrition to both physical and mental health. So knowing their nutritional intake allows dietitians and personal support workers to use trends over time to better understand the health status of residents and provide a higher quality of care.
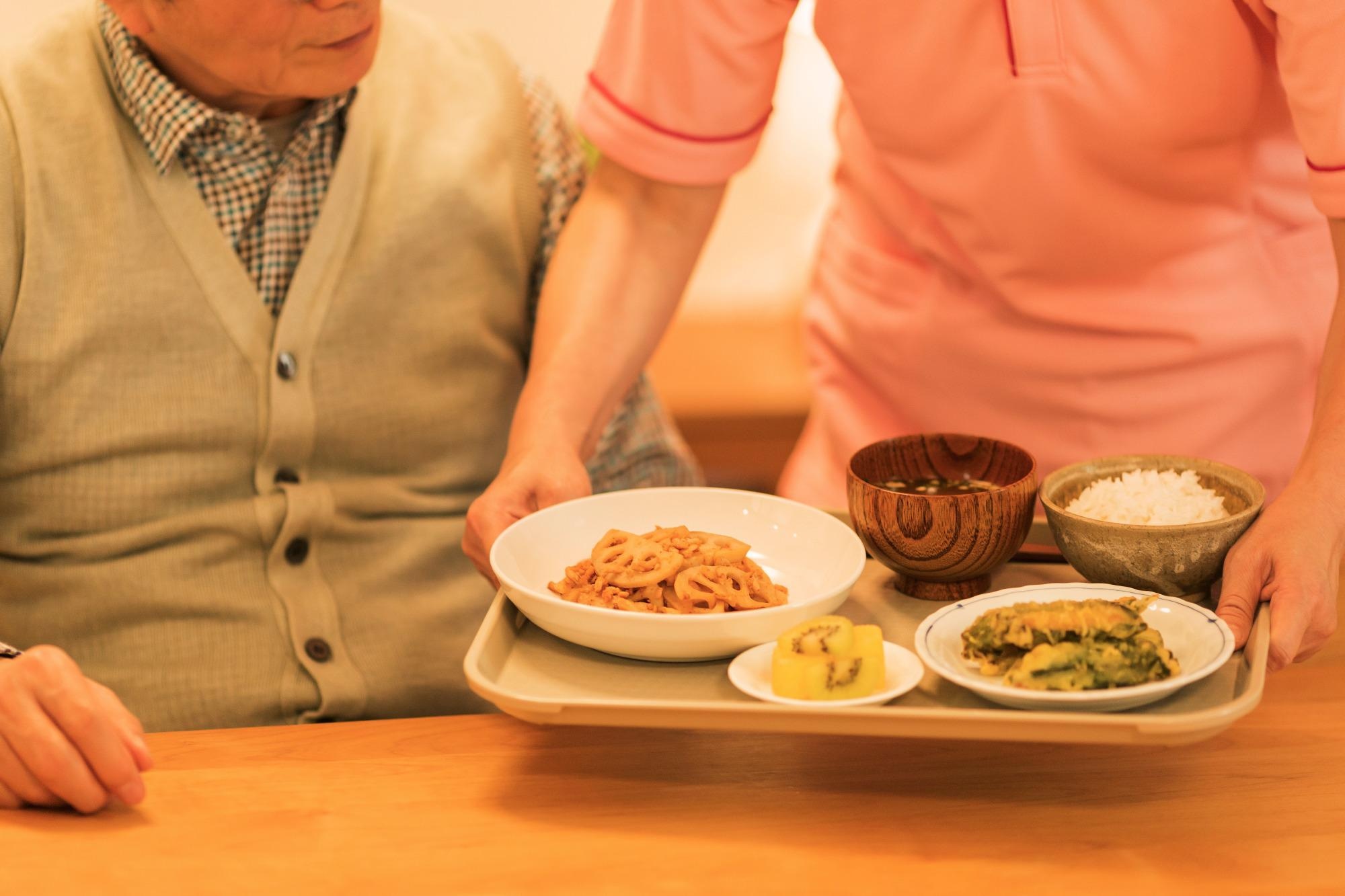
Image Credit: Shutterstock.com/ sukiyaki
Nutrition affects many facets of well-being – for example, if you are protein deficient, especially with age, it is harder to maintain muscle mass which leads to muscle weakening and increased risk for falls. Many nutritional deficiencies are challenging to measure directly and it is hard to know if it is because people are not eating enough or if there were metabolic changes to make it more difficult for them to extract the nutrients.
Measuring food intake, or monitoring nutritional status provides insight into what is going on and is the first step to prevention. Once we know how much and which nutrients are at risk, we can then develop more tailored nutritional interventions to support optimal health and wellbeing.
What are the limitations associated with conventional nutritional intake monitoring in LTC?
The conventional method of nutritional intake monitoring in LTC is based on manual human assessment, which is highly subjective and past studies have shown that there can be a 50 percent or more error rate. It also varies a lot between people who are conducting the assessment, making it subjective and the tools used are ambiguous.
For example, when a “meal” is assessed – is it all the items provided (including the soup appetizer and dessert), or is it just the main plate that gets assessed? If a resident prefers smaller portions, is the assessment done relative to their plate, or the standard size of a plate? There are a lot of moving parts and it is difficult to keep things consistent on the fly.
Can you give an overview of how your deep convolutional encoder-decoder food network works? What does it specifically analyze?
The deep convolutional encoder-decoder food network works by learning from a collection of camera and depth-sensing data of plates with different mixes and types of foods that are typically found in meals at LTC homes.
The food network then learns the relationship between the food types and the output sensing data and is then able to take in a set of input camera and depth-sensing data of a resident’s plate after their meal. The food network then automatically localizes the different types of food left on the plate as well as determines the remaining food volume relative to reference portions to provide food intake reporting information.
In other words, it takes a scan of the plate to see how much food is there, then automatically determines what food was on the plate, and then using the LTC home’s unique recipes, provides a nutrient-level food intake report.
Studies show that manually recording estimates of food consumption results in an error rate of 50% or more. How does your method compare to more traditional forms of manually assessing food intake? What advantages does it offer?
Our method is accurate to within 5 percent, which is significantly better than manual estimates and gives a much finer grain, reliable profile on the consumption patterns of a resident. Our method also looks at what food groups were consumed. Current manual assessment methods take a theoretical average across all foods offered, they are not based on what a resident actually selects. They are also put into 25% buckets so it is a very coarse-grained estimate that is difficult to interpret and the values are not considered trustworthy.
Our approach gives insight at the mL level for bulk food intake, but it also assesses which foods were consumed generating a report at the nutrient level for each resident.
How did you assess the efficacy of your smart system, and what were the results?
We collected a good dataset of different plates with different types and mixtures of food found at an LTC home, and also annotated the data with “ground-truth” so that we can use it to assess the efficacy of the system. The results are very promising with the system achieving accuracy to within 5 percent on the dataset. Our bulk food intake estimates are within 0.5 mL of ground-truth weighed food estimates, with specific nutrient intake estimation in excellent agreement with the weighed food method.
A weighed food method is the gold standard but it is not practical because every single item needs to be weighed before and after a meal – it takes way too long. With our approach, yielding values are essentially equivalent, but only one image is required, so, it will hopefully open the door for how we can re-think nutrition monitoring can be done in LTC.
Did you come across any challenges during your research, and if so, how did you overcome them?
Translational research or getting the research from inception through to the final stage into the hands of users is really challenging. There is a lot that is needed to go from a working prototype, especially when what is being proposed may have policy implications or change workflow.
To minimize these barriers, we co-designed the technology directly with dietitians, personal support workers and directors of food services. We also leveraged Kaylen’s previous experience of conducting meal-time observations and she had interviews to understand the workflow of the current environment.
By doing this, it meant that we could understand the complexities around mealtime and food intake tracking on a larger scale. It allowed us to probe the challenge from a holistic perspective. While we still need to validate this system in the real world, by having co-designed the AFINI-T technology, we have a good understanding of what challenges and limitations are left to overcome.
What impact will such a novel system have on LTC?
The vision is that using AFINI-T would allow for a more holistic assessment of health, and would give more accurate data-driven insights into the role of nutrition in each resident’s chronic disease management, flagging prioritized referrals for a better quality of care to support improved (or maintained) quality of life. This could mean determining how to make the most loved foods more nutrient-packed or translate to earlier detection of malnutrition risk and easier evaluation of nutritional interventions.
We want AFINI-T to elegantly work in the background and to free up time for personal support workers so they can spend more time doing meaningful activities with residents.
Mandated food and fluid intake tracking of at-risk residents is difficult to accurately assess. AFINI-T takes the guesswork out to get more reliable and accurate intake measurements. In future versions, we want to build in learned resident preferences which could also address the challenges around resident-directed care with high staff turnover.
Our user study suggested that the technology can give confidence to team members who are less familiar with residents in a given neighborhood.
It is not meant to undermine resident choices and preferences, but instead, provide a tool to further enhance communication while providing more accurate and reliable data.
More accurately tracking intake could also help reduce food waste, inform menu planning and provide insights into opportunities for enhanced recipes.
What are the next steps for your research?
The next steps involve working with LTCs and dietitians to further explore the best ways in which to capture and visualize the plethora of information being captured and collected so that they can easily understand and make use of it and integrate it to monitor and assess the health status of residents, and identify early warning signs, as well as leverage it for different applications such as monitoring infection control.
About Prof. Alexander Wong
Alexander Wong, P.Eng., is currently the Canada Research Chair in Artificial Intelligence and Medical Imaging, a Member of the College of the Royal Society of Canada, co-director of the Vision and Image Processing Research Group, and a professor in the Department of Systems Design Engineering at the University of Waterloo. Dr. Wong is a world-recognized expert in operational artificial intelligence who has published over 600 scientific articles and is the recipient of ~60 research awards for his research contributions, industrial contributions, and engineering teaching excellence. Dr. Wong holds over 38 patents and patent applications for his technological innovations, with his patented technologies, are reaching world markets through industry integration and deployment by multinationals around the world as well as through clinical implementation around the world. Dr. Wong has given over 90 keynote and invited talks, and his research has appeared in over 400 news articles, TV interviews, and radio interviews at top media outlets around the world.
Disclaimer: The views expressed here are those of the interviewee and do not necessarily represent the views of AZoM.com Limited (T/A) AZoNetwork, the owner and operator of this website. This disclaimer forms part of the Terms and Conditions of use of this website.