Feb 1 2021
The term 'coronary artery calcification' refers to the accumulation of calcified plaque in the arterial walls of the heart. This condition is a crucial predictor of unfavorable cardiovascular events, including heart attacks.
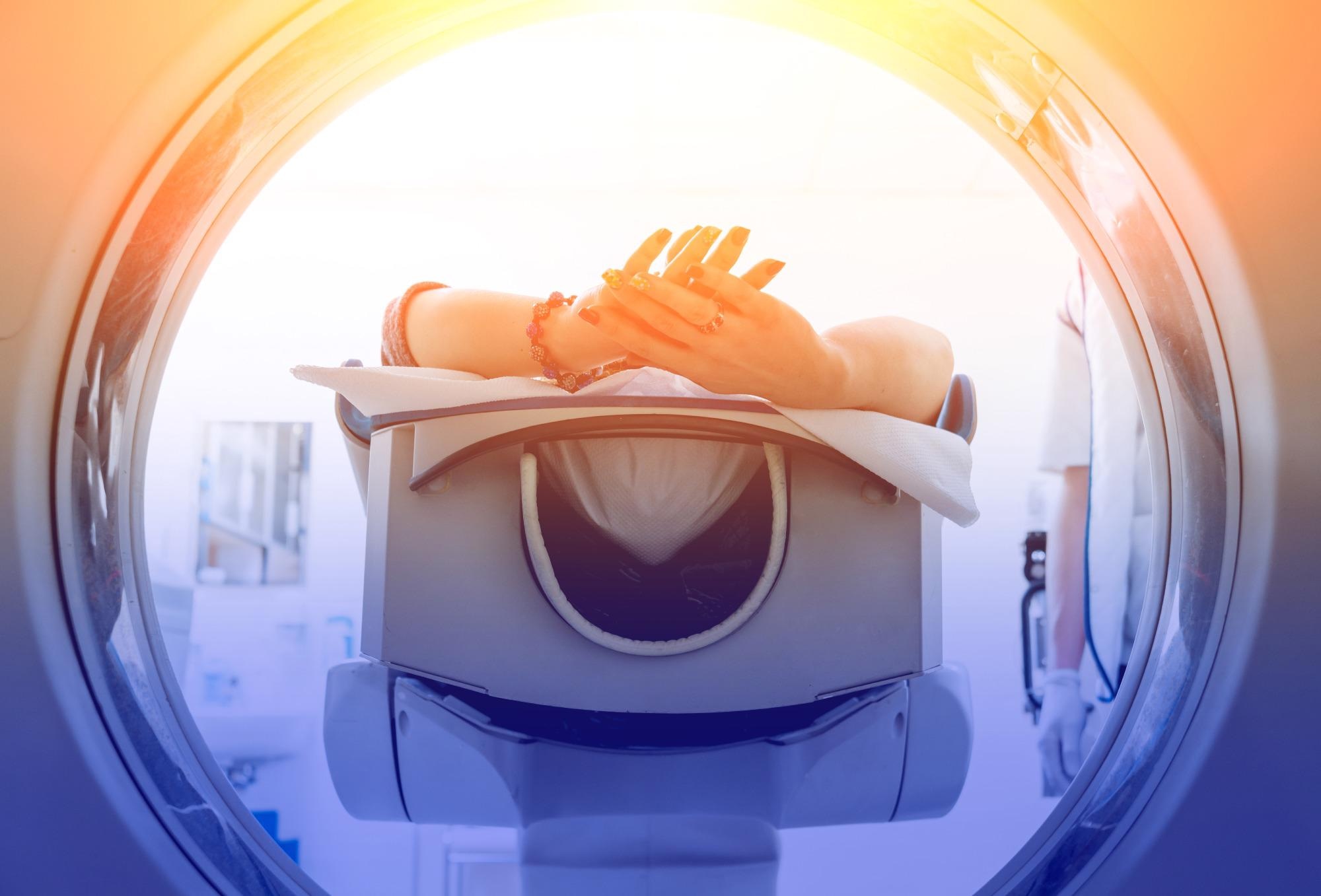
Image Credit: Roman Zaiets/shutterstock
While computed tomography (CT) scans can help detect coronary calcium, measuring the amount of plaque needs time, dedicated equipment, and radiological know-how. While chest CT scans are quite common in practice, calcium score CTs are not.
Now, researchers from Brigham and Women’s Hospital’s Artificial Intelligence in Medicine (AIM) Program and the Cardiovascular Imaging Research Center (CIRC) at Massachusetts General Hospital have collaborated to design and assess a deep learning system that may help change this scenario.
The deep learning system automatically quantifies coronary artery calcium from CT scans to help patients and physicians make more informed decisions about the prevention of cardiovascular events.
By using data from over 20,000 people, the researchers validated the deep learning system with potential results. The team’s findings were recently published in the Nature Communications journal.
Coronary artery calcium information could be available for almost every patient who gets a chest CT scan, but it isn’t quantified simply because it takes too much time to do this for every patient. We’ve developed an algorithm that can identify high-risk individuals in an automated manner.
Hugo Aerts, PhD, Study Corresponding Author and Director, Artificial Intelligence in Medicine Program, Brigham and Harvard Medical School
In association with collaborators, Roman Zeleznik, MSc, the lead author of the study and a data scientist in the Artificial Intelligence in Medicine Program (AIM), designed the deep learning system illustrated in the article to precisely and automatically estimate adverse cardiovascular events by scoring coronary calcium.
At present, the deep learning system is only used for research purposes, but Zeleznik and the co-authors of the study have made it a freely available and open source for anyone to utilize.
In theory, the deep learning system does a lot of what a human would do to quantify calcium. Our paper shows that it may be possible to do this in an automated fashion.
Roman Zeleznik, MSc, Study Lead Author and Data Scientist, Artificial Intelligence in Medicine Program, Brigham and Harvard Medical School
The researchers started by training the deep learning system on information obtained from a long-term asymptomatic community cohort study called the Framingham Heart Study (FHS).
Dedicated calcium scoring CT scans were performed on FHS participants, and these scans were physically scored by expert human readers and applied to train the deep learning system.
The team subsequently used the new deep learning system on three more study cohorts, which comprised patients with acute chest pain who had cardiac CT [the Rule Out Myocardial Infarction utilizing Computer Assisted Tomography trial (ROMICAT-II)]; patients with stable chest pain who had cardiac CT [(the Prospective Multicenter Imaging Study for Evaluation of Chest Pain (PROMISE)]; heavy smokers who had lung cancer screening CT [National Lung Screening Trial (NLST)].
On the whole, the researchers verified the deep learning system in more than 20,000 people.
Udo Hoffmann, MD, director of CIRC at Massachusetts General Hospital and the principal investigator of CT imaging in the FHS, ROMICAT and PROMISE, highlighted that one of the special aspects of the new analysis is the inclusion of three high-quality image and outcome trials, funded by the National Heart, Lung and Blood Institute—that reinforced the generalizability of these outcomes to clinical settings.
The automated calcium scores obtained from the deep learning system corresponded well with the physical calcium scores obtained from human experts. Besides, the automated scores separately predicted which individuals are likely to have a major adverse cardiovascular event, such as a heart attack.
The coronary artery calcium score has a crucial role to play in present guidelines for which individuals must take a statin to avoid heart attacks.
This is an opportunity for us to get additional value from these chest CTs using AI. The coronary artery calcium score can help patients and physicians make informed, personalized decisions about whether to take a statin. From a clinical perspective, our long-term goal is to implement this deep learning system in electronic health records, to automatically identify the patients at high risk.
Michael Lu, MD, MPH, Study Co-Author and Director of Artificial Intelligence, Cardiovascular Imaging Research Center, Massachusetts General Hospital
The study was funded by the National Institutes of Health (NIH-USA U24CA194354, NIHUSA U01CA190234, NIH-USA U01CA209414, NIH-USA R35CA22052, 5R01-HL109711, NIH/NHLBI 5K24HL113128, NIH/NHLBI 5T32HL076136, NIH/NHLBI 5U01HL123339) and the European Union—European Research Council (866504).
It was also supported by the German Research Foundation (1438/1-1, 6405/2-1), American Heart Association Institute for Precision Cardiovascular Medicine (18UNPG34030172), Fulbright Visiting Researcher Grant (E0583118), and a Rosztoczy Foundation Grant.
Journal Reference:
Zeleznik, R., et al. (2021) Deep convolutional neural networks to predict cardiovascular risk from computed tomography. Nature Communications. doi.org/10.1038/s41467-021-20966-2.
Source: https://www.brighamandwomens.org/