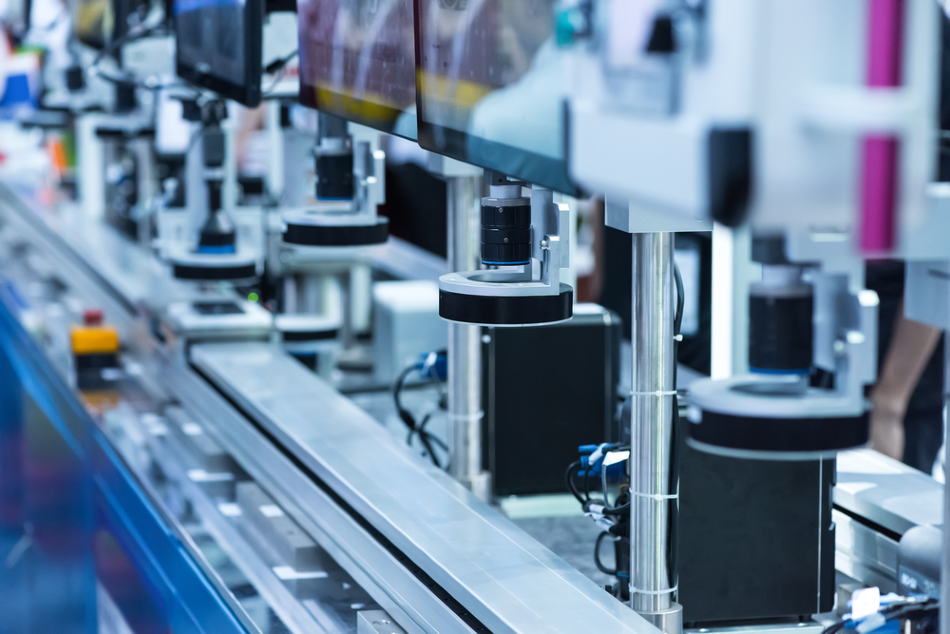
Image Credit: asharkyu/Shutterstock.com
The industrial automation sector is growing rapidly, and much of this growth is attributed to the increasing demand for industrial robots with AI capabilities to do jobs such as pick and place, part transfer, packing and semiconductor manufacturing.
In 2018, the market was valued at a huge $157.04 billion and was predicted to grow rapidly at a CAGR of 8.4% until 2026, bringing it to a value of $296.7 billion.
To meet the requirements of an expanding customer base, the industry will be focusing on developing its capabilities to extend offerings and benefits to customers.
Material Analysis Will Be Developed For Future Industrial Automation Systems
Automation will develop in three levels. The first will be at the hardware and software level where capabilities such as natural language conversations, self-programmable PLC, autonomous flexibility, and predictive maintenance will be the focus of imminent developments.
The second level will be developing attributes such as real-time processing and expandable storage in the CPU/memory of the computers managing the automation systems.
The third level will be improving the output and visualization capabilities of these systems, such as simulative and immersive technologies, real-time work instructions, improved co-ordination, high-resolution machine vision, and material analysis. This article will focus on the development of one of these capabilities, in particular, that of material analysis.
Electron Diffraction is Vital to Developing Material Analysis
Electron diffraction is essential in developing material analysis capabilities in next-generation industrial automation equipment.
The wave nature of electrons is described by electron diffraction. It is a technique that investigates materials and indeed any matter by investigating the interference pattern caused by firing electrons at a sample. Scientists describe this effect as the wave-particle duality, the laws of which state that electrons can be regarded as waves.
Electron diffraction has a number of uses in hard science, mostly in solid-state physics, as well as in chemistry for the purpose of studying a material’s chemical structure. Uncovering the chemical structure of a sample can be useful in identifying it. This can be vital in automation, allowing machines to visually inspect and recognize items without human assistance, as well as playing an essential role in quality control.
The main method of carrying out electron diffraction experiments is with a transmission electron microscope (TEM), although a scanning electron microscope can also be used. The process involved in using either of these instruments involves using an electrostatic potential to accelerate electrons until they gain the desired level of energy. Their wavelength is determined prior to them interacting with the sample under investigation.
The electrons are then passed through the sample, where they are scattered, before moving through an electromagnetic objective lens, which has the effect of focusing the scattered electrons that originate from one particular point and move into a specific point on the image pane.
Electrons scattered in the same direction, converging onto a single point, is the objective lens' back focal plane and where the diffraction pattern is formed.
Material identification by electron diffraction has been around since the 1950s. It can be used to assist in robotic industrial automation by coupling it with artificial intelligence, enabling robots to recognize the items it is handling. This may be crucial to certain roles, such as putting together parts in car assembly applications.
The technique can also be used to obtain data on crystalline structures, stress, contamination, internal fractures, and more, helping robots to automatically spot faults, preventing them from continuing their journey to the consumer.
Future Advancements in Industrial Automation
Adding this capability to automation processes would enhance them by giving them the means to quality test products without the need for human workers to remove samples from the production line and engage in lengthy testing processes.
Electron diffraction can establish the short range order of amorphous solids, identify imperfections known as vacancies, and study the geometry of gaseous molecules. It can also help identify stress and internal fractures that may compromise the function of the quality of the product. Automatically spotting these features can enable them to be automatically removed from the production line.
Click here for more information on service robotics and automation.
As discussed, electron diffraction is an essential tool in material identification, which may provide useful in combination with artificial intelligence to guide robots in more complex automation processes.
The challenge moving forward is to establish reliable ways to incorporate electron diffraction methods into industrial automation robotics. The future of industrial automation will likely see these advancements being made to enhance the output and visualization capabilities in the field.
These will not be the only advancements in the field. We can also expect major advancements in hardware and software as well as computer CPU/memory to emerge in the coming years.
References and Further Reading
Bendersky, L. and Gayle, F., 2001. Electron diffraction using transmission electron microscopy. Journal of Research of the National Institute of Standards and Technology, 106(6), p.997. https://www.ncbi.nlm.nih.gov/pmc/articles/PMC4865294/
Deraz, N., 2010. Size and crystallinity-dependent magnetic properties of copper ferrite nano-particles. Journal of Alloys and Compounds, 501(2), pp.317-325. https://www.sciencedirect.com/science/article/abs/pii/S0925838810009126
Industrial Automation Market Will Rise at a CAGR of 8.4%; Increasing Demand for AI-Based Industrial Robots Will Aid Growth, Says Fortune Business Insights. Available at: https://www.prnewswire.com/in/news-releases/industrial-automation-market-will-rise-at-a-cagr-of-8-4-increasing-demand-for-ai-based-industrial-robots-will-aid-growth-says-fortune-business-insights-866899795.html
Matthews, H. and Wilman, H., 1955. Identification of materials by electron diffraction in the electron microscope. British Journal of Applied Physics, 6(8), pp.277-280. https://iopscience.iop.org/article/10.1088/0508-3443/6/8/303
The Future of Automation – A Primer. Available at: www.google.com
Disclaimer: The views expressed here are those of the author expressed in their private capacity and do not necessarily represent the views of AZoM.com Limited T/A AZoNetwork the owner and operator of this website. This disclaimer forms part of the Terms and conditions of use of this website.