Machine learning (ML) and artificial intelligence (AI) can now process the huge amounts of data generated by fields like astronomy, particle physics, life sciences, and social sciences. As such, these technologies can be a crucial enabler for science in general, helping to push the boundaries of discovery even further.
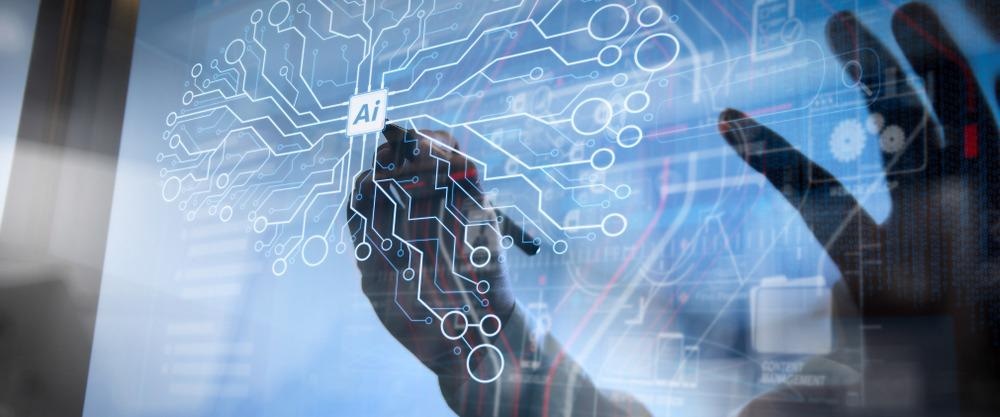
Image Credit: everything possible/Shutterstock.com
Science and Data
Scientists formulate hypotheses, design experiments, and collect data to understand how nature and society work. Their goal is to analyze the data on various natural or social phenomena to understand them better.
Devising techniques or methodologies for working with data better is nothing new. Statistical analysis techniques, the scientific method, experiment design, and research planning are all utilized by scientists trying to organize and analyze their data as well as possible.
With the explosion of computing processing power from the mid-20th century onwards, scientists have increasingly turned to machine thinking to help them with this task.
How Is AI Used in Science?
Now, AI technology is used all over the broad spectrum of scientific endeavors.
Using Genomic Data to Predict Protein Structures
It is essential to know proteins’ shapes so that we can determine the role they play in the body. Scientists use AI to help predict the forms of proteins and how they interact with diseases, in turn improving diagnosis and helping to develop new treatments.
Protein structures can be determined through a defined process, but it is technically challenging and labor-intensive. Researchers are using ML approaches to predict proteins’ three-dimensional structure from DNA sequences. The rich datasets of DNA that have been obtained in recent years through the Human Genome Project made this possible.
The AlphaFold project at DeepMind is working on this problem. It uses a deep neural network AI approach that predicts the distance between pairs of amino acids and the angles needed between their bonds, creating a highly accurate prediction of a protein’s overall structure.
Understanding The Effects of Climate Change
Environmental scientists need to analyze large amounts of data and apply complex systems modeling to understand and forecast climate change effects. Predictions from global climate models inform local, national, and international decision- and policy-making.
Local areas often have a wealth of detailed observations data about local environmental conditions, such as from weather stations. These are not tied into standard global-scale climate modeling, however.
Powerful AI is being used to tie these two vast, discrete data sources together. Doing so can help local areas to plan better for the impending effects of climate change, such as rising sea levels or increasing temperatures.
A University of Cambridge research project is underway to combine these sources and predict changes impacting cotton production in Egypt in coming years. These predictions can be used to create climate change resilience strategies for communities in the area.
Looking for Patterns in Astronomical Data
Astronomy research is another field that generates huge amounts of data, presenting the key challenge of analysis. Finding something of interest among the vastness of empty space – noise in the data – and logging has been the lonely task of the astronomer for centuries.
AI can help.
Kepler is a space observatory launched in 2009 to seek out Earth-sized planets orbiting other stars in the Orion Spur and beyond. However, the data it collects can be distorted by so-called instrumental artifacts. Researchers use an AI system to remove the distortions from the data.
AI has also found new things to study in space. Pulsars, supernovae, and galaxies have been identified and classified by AI systems, which also improved our understanding of stars’ properties.
The difference between AI in science fiction and reality
Video Credit: Interesting Engineering/YouTube.com
Cutting Edge Cases for AI in Science
Researchers are working on new ways to incorporate AI and ML techniques into a wide range of disciplines.
Social historians and curators are building new software that can analyze data from the millions of pages of newspapers and other historical collections in the British Library, UK. Computational linguists and historians are using the software to track social changes that democratizes history by placing the lives of ordinary people in the foreground.
High-resolution imaging data is regularly crawled by AI to carry out spectroscopic analyses, characterizing new materials effectively.
Particle physics experiments, like astronomy, generate huge amounts of physical data. Now, researchers are using AI to attempt to characterize dark matter using this data.
The Future for AI-Enhanced Innovation
AI technology can still be brought to bear on various scientific disciplines, bringing considerable social and economic benefits.
Existing data analysis processes are ripe for the introduction of AI to automate laborious tasks and enhance pattern recognition. It can also refine scientific models, generate hypothetical data to compare with real data and update hypotheses accordingly.
In this way, AI is used to perform the tasks of scientific hypothesis refinement and experimental data collection, freeing scientists to determine research questions and perform sophisticated analyses.
AI also promises to bring unexpected benefits to science, as it can set its own research direction and choose its own questions. This is an emergent area of AI technology that will progress in the coming years.
The main barrier to AI’s advance in science – and anywhere – is computing power. Advanced computing approaches like quantum computing promise to unleash AI’s potential, making an infinitesimally high number of calculations in an infinitesimally small amount of time.
Coupled with advances in computing power, AI will progress as our understanding of algorithms and computing develops.
Both in computing power and algorithmic capability, AI can also speed up its own advancement. By applying AI to these fields – as it is applied throughout the sciences – scientists may invent a self-improving branch of science.
Continue reading: Using Machine Learning and AI with Encrypted Data.
References and Further Reading
The Royal Society and The Alan Turing Institute (2017) The AI revolution in scientific research. Royal Society. [online] Available at: https://royalsociety.org/-/media/policy/projects/ai-and-society/ai-revolution-in-science.pdf?la=en-GB&hash=5240F21B56364A00053538A0BC29FF5F
Disclaimer: The views expressed here are those of the author expressed in their private capacity and do not necessarily represent the views of AZoM.com Limited T/A AZoNetwork the owner and operator of this website. This disclaimer forms part of the Terms and conditions of use of this website.