Artificial intelligence is becoming increasingly popular in healthcare and dentistry. Traditional methods have evolved into digital techniques in recent times with the advent of new software that can be employed with a variety of medical instruments.
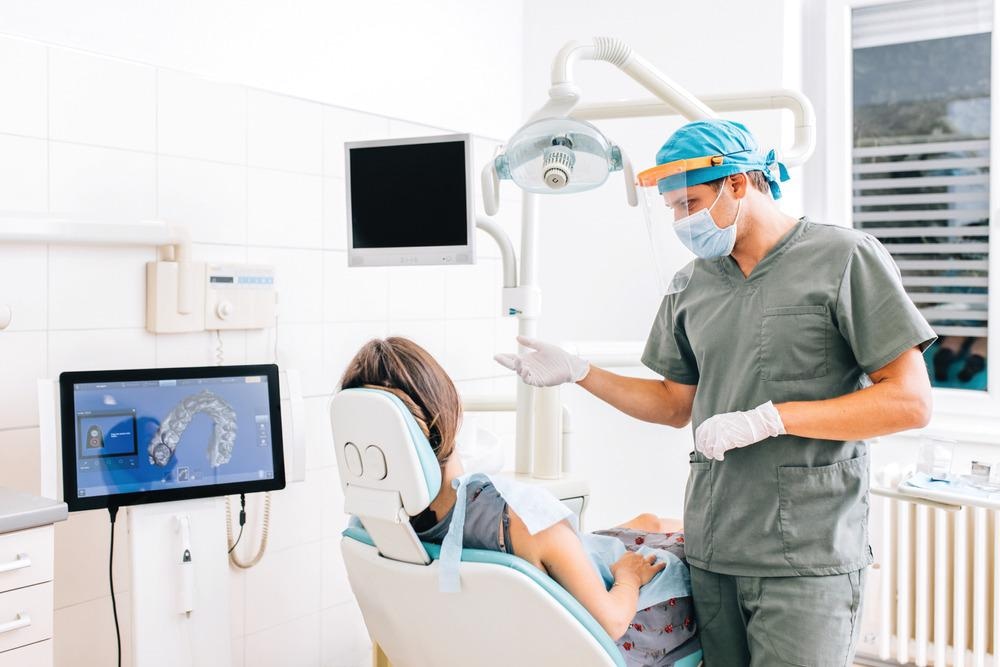
Image Credit: astarot/Shutterstock.com
Artificial intelligence (AI) is gaining traction in public healthcare as more people attempt to diagnose patients using technology that helps them work quickly and effectively, lowering expenses and minimizing medical mistakes.
When AI is applied to the massive amounts of data generated by healthcare facilities, it can provide tremendous benefits and a wide range of contributions, such as predictive, more targeted, and more precise symptom identification, automated use of analysis results, implementation of treatment plans or customized protocols, and enablement of care team coordination.
AI in dentistry can be used for a variety of purposes, ranging from dental emergencies to prosthesis planning. Machines can learn from their mistakes, adapt to different inputs, and perform jobs in a human-like manner, thanks to artificial intelligence.
AI will be able to measure the success of various treatment options linked with specific symptoms and anatomical conditions and enhance the quality of standardization procedures by utilizing enormous volumes of data, like diagnostic results, treatments, and outcomes.
The major goal of this pilot study was to assess the accuracy of a screening tool based on machine learning and artificial intelligence. The secondary goal was to evaluate the tool’s effectiveness in diagnosing various dental disorders and conditions.
Methodology
In this study, 120 panoramic X-rays (OPGs) were chosen at random from the Sapienza University of Rome’s Department of Oral and Maxillofacial Sciences. The OPGs may be jpeg (JPEG) or dicom (DICOM) (DICOM or DCM). Apox was used to create the OPGs, which capture a panoramic X-ray and convert it into clinical insights.
After 1 minute, the software examines the OPGs uploaded and delivered the following results, as shown in Figure 1.
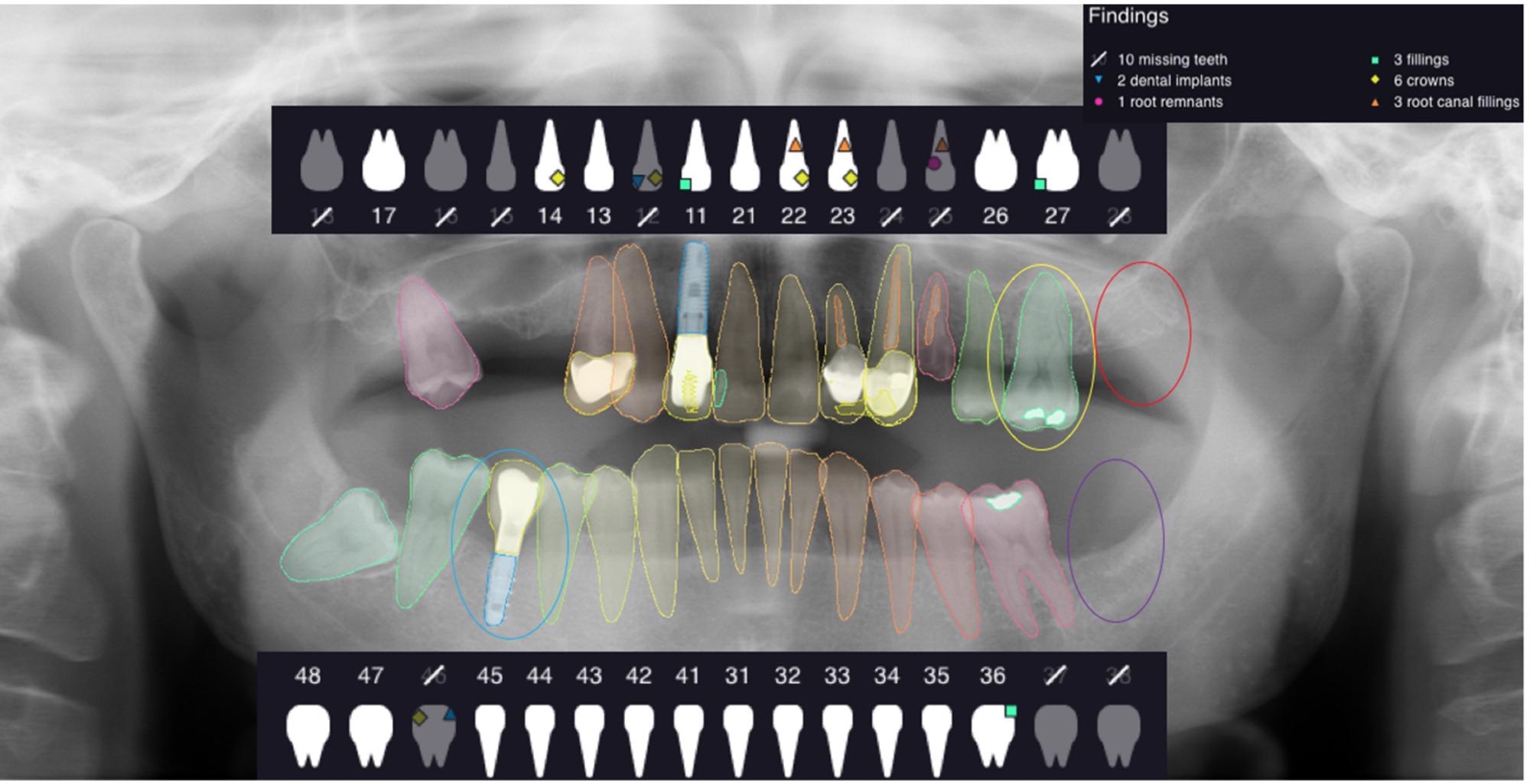
Figure 1. The figure shows an example of analyzed OPG and the outcomes given by the software. Image Credit: De Angelis, et al. 2022
The value of true negative (TN) was assigned when the same analyzed elements were not in the right position; the value of false negative (FN) was delegated when the software did not identify the presence of the analyzed element, and the value of false positive (FP) was delegated when the software showed the presence of one of the elements under analysis that did not even exist (Figures 2–5).
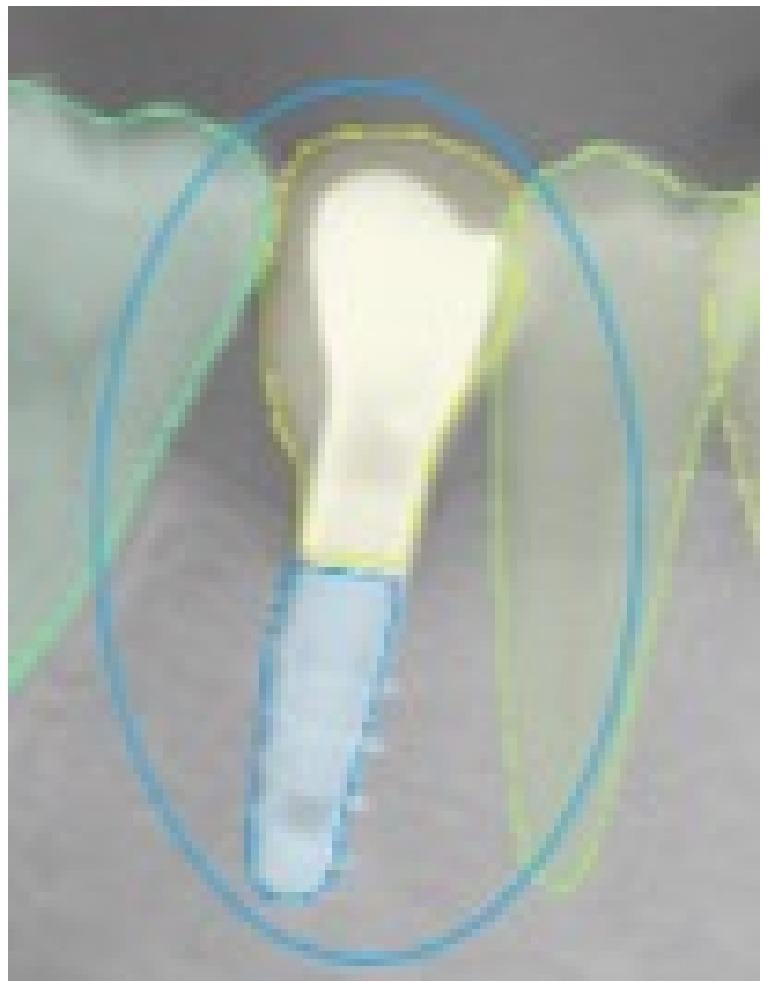
Figure 2. This selected view of Figure 1 shows the correct detection in the right position of an implant. The assigned value was TP. Image Credit: De Angelis, et al. 2022
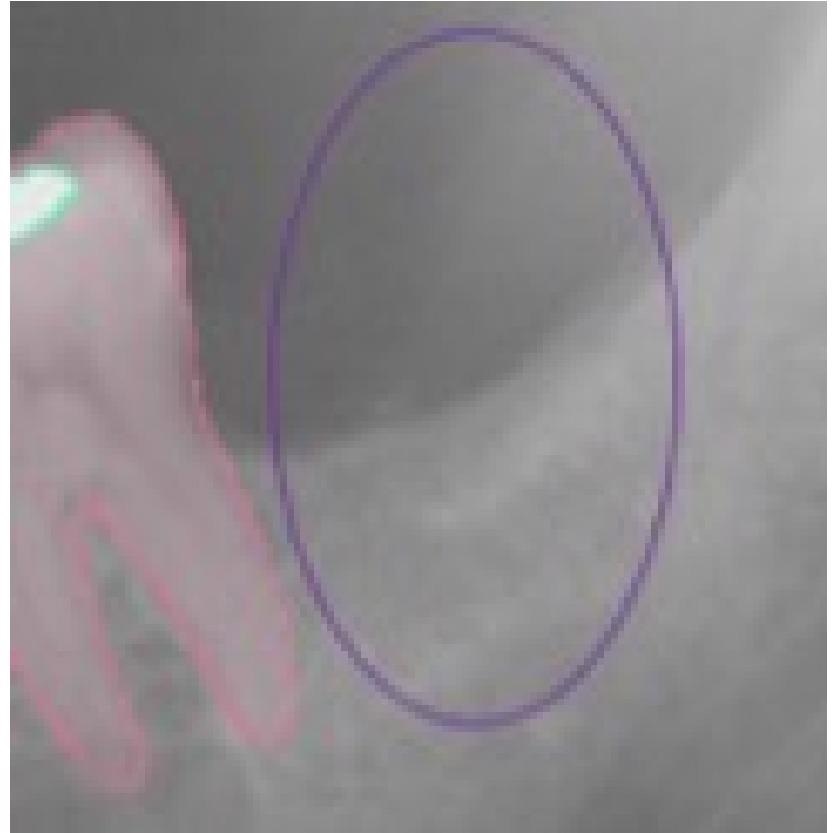
Figure 3. This selected view of Figure 1 shows the correct detection in the right position of an absent tooth in position 3.7. The assigned value was TN. Image Credit: De Angelis, et al. 2022
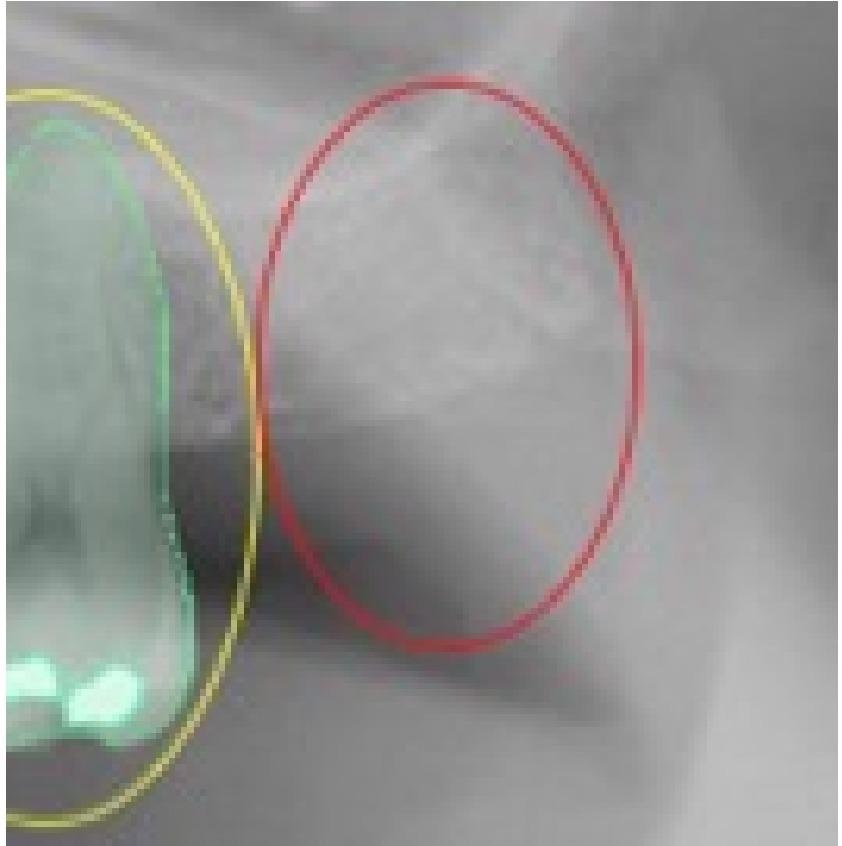
Figure 4. This selected view of Figure 1 shows the incorrect detection in the right position of the tooth 2.7. The assigned value was FP. Image Credit: De Angelis, et al. 2022
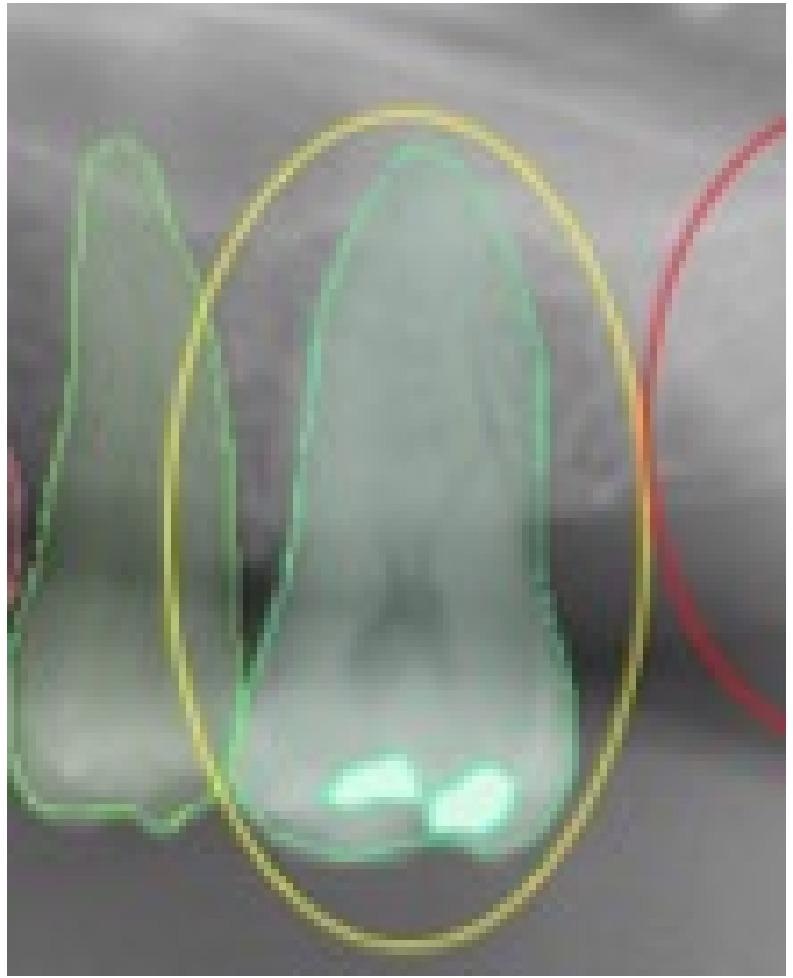
Figure 5. This selected view of Figure 1 shows the missing detection of the tooth filling in position 2.6. The assigned value was FN. Image Credit: De Angelis, et al. 2022
As seen in Figure 6, all values were given in an Excel table.

Figure 6. An example of data collected from an OPG using an Excel table. Image Credit: De Angelis, et al. 2022
The categorical variables were presented as relative and absolute frequencies in a descriptive analysis. Sensitivity, specificity, negative predictive value (NPV), positive predictive value (PPV), negative likelihood ratio (LR–), and positive likelihood ratio (LR+) were used to estimate diagnostic performance.
Results and Discussion
The Cohen’s kappa coefficient was 1.0, indicating that the data collectors were in complete agreement. Table 1 shows the calculated true positive (TP), true negative (TN), false positive (FP), false negative (FN) values for each category (teeth, implants, crowns, fillings, residual roots, and root canal procedures).
Table 1. TP, TN, FP, and FN values calculated for each group (teeth, implants, crowns, fillings, residual roots and root canal treatments). Source: De Angelis, et al. 2022
|
Freq. |
Percent. |
Cum. |
TP |
182 |
9.48 |
9.48 |
TN |
1684 |
87.71 |
97.19 |
FP |
16 |
0.83 |
98.02 |
FN |
38 |
1.98 |
100.00 |
Total |
1920 |
100.00 |
|
Figure 7 shows the area under the Receiver operating characteristic (ROC) curve as 0.94 (AUC) after controlling for the variable group as a covariate.
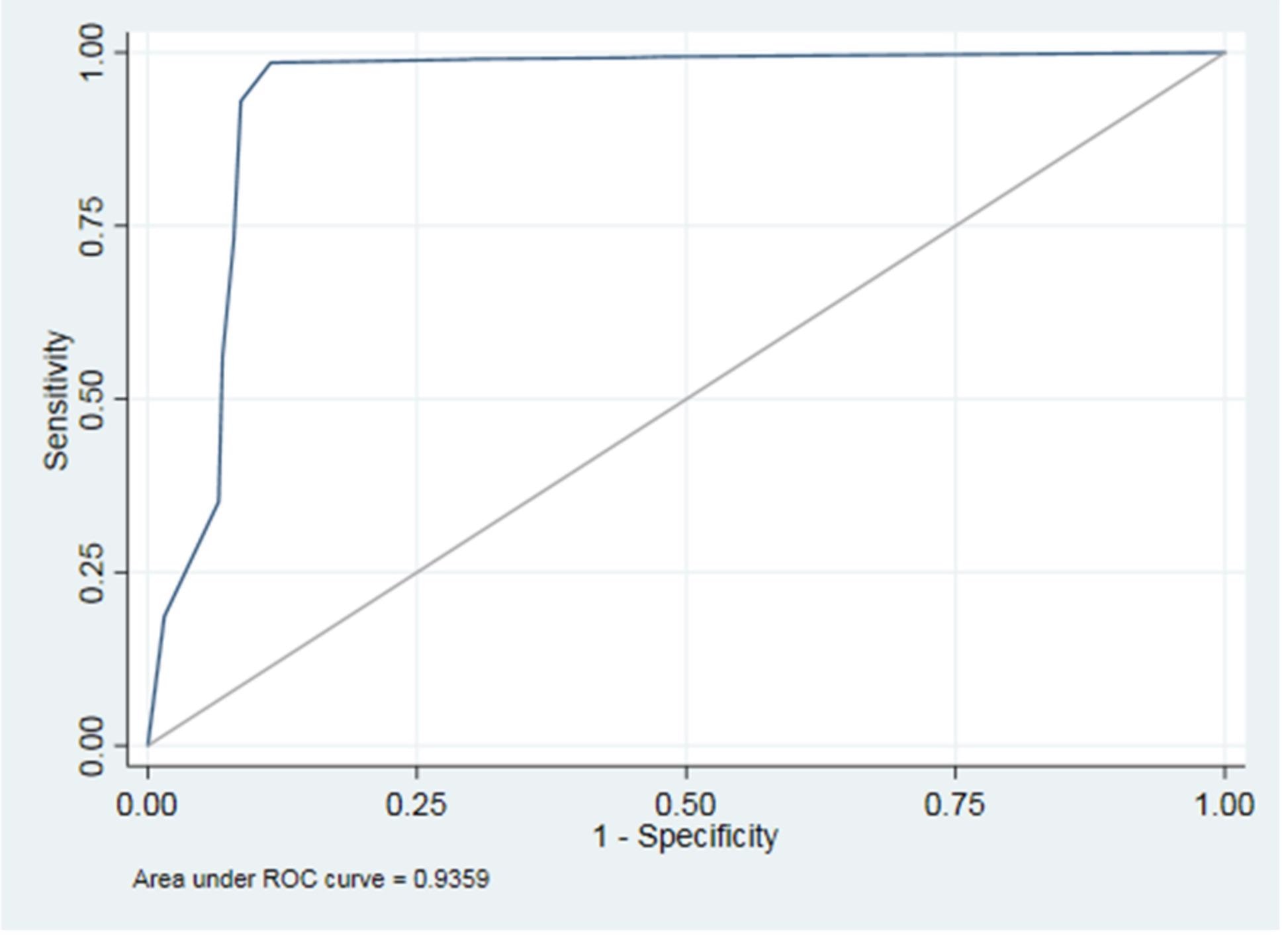
Figure 7. The area under the ROC curve (AUC). Image Credit: De Angelis, et al. 2022
Table 2 shows the AUCs evaluated for the ROC curves of each group variable and Figure 8 depicts the ROC curves for all variables.
Table 2. The AUCs calculated for the ROC curves of each group variable. Source: De Angelis, et al. 2022
Group |
Obs |
ROC |
Std. Err. |
[95% Conf. Interval] |
0 |
1920 |
0.9253 |
0.0070 |
0.91162 0.93901 |
1 |
1919 |
0.9164 |
0.0184 |
0.88027 0.95259 |
2 |
1920 |
0.9600 |
0.0067 |
0.94694 0.97308 |
3 |
1920 |
0.8030 |
0.0520 |
0.70106 0.90500 |
4 |
1919 |
0.8332 |
0.0118 |
0.81005 0.85643 |
5 |
1920 |
0.9089 |
0.0128 |
0.88379 0.93407 |
Figure 8. ROC curves of all variables. Image Credit: De Angelis, et al. 2022
Researchers have been looking for an appropriate and standardized way to diagnose the most prevalent dental diseases by employing two-dimensional (2D) X-rays and considering three-dimensional (3D) images derived from the CT cone beam in recent times.
A review of current literature on artificial intelligence systems in dental and maxillofacial radiology disclosed only in vitro studies, skull studies, and anatomical model studies.
The current study’s objectives were to evaluate the evaluation metrics of a screening tool based on artificial intelligence machine learning and analyze the tool’s precision for diagnosing various dental pathologies or conditions.
Researchers investigated the effectiveness of AI software (Promaton) to evaluate the ability to identify the number of teeth, roots, implants, and fillings by assessing the margin of error. They discovered that while the results are promising, a few errors still exist have to be avoided.
Dentistry is entering a new phase of medicine based on robot-assisted AI. Since AI has not yet been properly implemented in dental research, it appears to be a promising challenge.
With AI, researchers expect to obtain easier treatment protocols with minimal mistakes and boost the efficacy of the healthcare system in the future. Dentists will need to become acquainted with electronic systems, as well as the ability to engage with digital robots and humans.
Conclusion
Artificial intelligence has tremendous potential in dentistry. Artificial intelligence in dentistry is considered very beneficial for analyzing dental X-rays for earlier detection. All of this will result in a one-of-a-kind treatment plan for every patient, from planning to treatment and follow-up.
This software will be able to learn instantaneously and thus enhance its effectiveness over time. As a result, AI assists clinicians in improving the quality of their diagnosis and treatment, saving time, and generating revenue.
To properly utilize the benefits of AI, it is necessary to dose the use of these tools with intellectual ability, objectivity, and practical wisdom, as well as a suitable learning curve.
Journal Reference:
De Angelis, F., Pranno, N., Franchina, A., Di Carlo, S., Brauner, E., Ferri, A., Pellegrino, G., Grecchi, E., Goker, F., Stefanelli, L. V. (2022) Artificial Intelligence: A New Diagnostic Software in Dentistry: A Preliminary Performance Diagnostic Study. International Journal of Environmental Research and Public Health, 19(3), p. 1728. Available Online: https://www.mdpi.com/1660-4601/19/3/1728/htm.
References and Further Reading
- Machoy, M. E., et al. (2020) The ways of using machine learning in dentistry.Advances in Clinical and Experimental Medicine, 29(3), pp. 375–384. doi.org/10.17219/acem/115083.
- Wong, S. H., et al. (2019) Artificial intelligence in radiology: How will we be affected? European Radiology, 29, pp. 141–143. doi.org/10.1007/s00330-018-5644-3.
- McCarthy, J (1989) Artificial Intelligence, Logic and Formalizing Common Sense. In: Philosophical Logic and Artificial Intelligence; Thomason, R H, Edison; Springer: Dordrecht, The Netherlands. doi.org/10.1007/978-94-009-2448-2_6.
- Howard, J (2019) Artificial intelligence: Implications for the future of work. American Journal of Industrial Medicine, 62, pp. 917–926. doi.org/10.1002/ajim.23037.
- Turing, A M (1950) Computing machinery and intelligence. Mind, 49, pp. 433–460. Available online: https://www.csee.umbc.edu/courses/471/papers/turing.pdf (accessed on 23 September 2021).
- Kulkarni, S., et al. (2020) Artificial Intelligence in Medicine: Where Are We Now? AcademicRadiolohy, 27, pp. 62–70. doi.org/10.1016/j.acra.2019.10.001.
- Joda, T., et al. (2020) Recent Trends and Future Direction of DentalResearch in the Digital Era. International Journal of Environmental Research and Public Health, 17(6), p. 1987. doi.org/10.3390/ijerph17061987.
- Thrall, J. H., et al. (2018) Artificial intelligence and machine learning in radiology: Opportunities, challenges, pitfalls, and criteria for success. Journal of the American College of Radiology, 15(3), pp. 504–508. doi.org/10.1016/j.jacr.2017.12.026.
- Hosny, A., et al. (2018) Artificial intelligence in radiology. Nature Reviews Cancer, 18, pp. 500–510. doi.org/10.1038/s41568-018-0016-5.
- Nagi, R., et al. (2020) Clinical applications and performance of intelligent systemsin dental and maxillofacial radiology: A review. Imaging Sciencein Dentistry, 50(2), pp. 81–92. doi.org/10.5624/isd.2020.50.2.81.
- Hiraiwa, T., et al. (2019) A deep-learning artificial intelligencesystem for assessment of root morphology of the mandibular first molar on panoramic radiography. Dentomaxillofacial Radiology, 48(3), p. 20180218. doi.org/10.1259/dmfr.20180218.
- Chen, Y.W., et al. (2020) Artificial intelligence in dentistry: Current applications and future perspectives. Quintessence international, 51(3), pp. 248–257. doi.org/10.3290/j.qi.a43952.
- Currie, G (2019) Intelligent Imaging: Anatomy of Machine Learning and Deep Learning. Journal of Nuclear Medicine Technology, 47(4), pp. 273–281. doi.org/10.2967/jnmt.119.232470.
- Park, W J & Park, J B (2018) History and application of artificial neural networks in dentistry. European Journal of Dentistry, 12(4), pp. 594–601. doi.org/10.4103/ejd.ejd_325_18.
- Vinayahalingam, S., et al. (2019) Automated detection of third molars and mandibular nerve by deeplearning. Scientific Reports, 9, p. 9007. doi.org/10.1038/s41598-019-45487-3.
- Hung, K., et al. (2020) The use and performance of artificial intelligence applicationsin dental and maxillofacial radiology: A systematic review. Dentomaxillofacial Radiology, 49(1), p. 20190107. doi.org/10.1259/dmfr.20190107.
- Miki, Y., et al. (2017) Classification of teeth in cone-beam CT usingdeep convolutional neural network. Computers in Biology and Medicine, 80, pp. 24–29. doi.org/10.1016/j.compbiomed.2016.11.003.
- GhazvinianZanjani, F., et al. (2019) Deep learning approachto semantic segmentation in 3D point cloud intra-oral scans of teeth. In: Proceedings of the 2nd International Conference onMedical Imaging with Deep Learning, CL South Kensington Campus, London, UK, 8–10 July 2019; Volume 102, pp. 557–571. Available online: http://proceedings.mlr.press/v102/ghazvinian-zanjani19a/ghazvinian-zanjani19a.pdf.
- Kim, T., et al. (2020) Tooth segmentation of 3D scan data using generative adversarial networks. Applied Science, 10, 490. doi.org/10.3390/app10020490.
- Bouletreau, P., et al. (2019) Artificial Intelligence: Applications in orthognathic surgery. Journal of Stomatology, Oral and Maxillofacial Surgery, 120(4), pp. 347–354. doi.org/10.1016/j.jormas.2019.06.001.
- Schwendicke, F., et al. (2020) Artificial Intelligence in Dentistry: Chances and Challenges. Journal of Dental Research, 99(7), pp. 769–774. doi.org/10.1177/0022034520915714.
- Shan, T, et al. (2021) Application of Artificial Intelligence in Dentistry. Journal of Dental Research, 100(3), pp. 232–244. doi.org/10.1177/0022034520969115.
- Pethani, F (2021) Promises and perils of artificial intelligence in dentistry. Australian Dental Journal, 66(2), pp. 124–135. doi.org/10.1111/adj.12812.
- Hung, K., et al. (2020) Current Applications, Opportunities, and Limitations of AI for 3D Imagingin Dental Research and Practice. International Journal of Environmental Research and Public Health, 17(12), p. 4424. doi.org/10.3390/ijerph17124424.
- Mupparapu, M., et al. (2018) Artificial intelligence, machine learning, neural networks, and deep learning: Futuristicconcepts for new dental diagnosis. Quintessence International, 49, pp. 687–688. doi.org/10.3290/j.qi.a41107.
- Grischke, J., et al. (2020) Dentronics: Towards robotics and artificial intelligence in dentistry. Dental Materials, 36(6), pp. 765–778. doi.org/10.1016/j.dental.2020.03.021.
- Leite, A. F., et al. (2020) Radiomics and Machine Learning in Oral Healthcare. Proteomics Clinical Application, 14(3), p. e1900040. doi.org/10.1002/prca.201900040.
- Rekow, E D (2020) Digital dentistry: The new state of the art—Is it disruptive or destructive? Dental Materials, 36(1), pp. 9–24. doi.org/10.1016/j.dental.2019.08.103.
- Das, S., et al. (2015) Applications of artificial intelligence in machine learning: Review and prospect. International Journal of Computer Application, 115(9), pp. 31–41. doi.org/10.5120/20182-2402.
- Zhang, Z & Sejdić, E (2019) Radiological images and machine learning: Trends, perspectives, and prospects. Computers in Biology and Medicine, 108, pp. 354–370. doi.org/10.1016/j.compbiomed.2019.02.017.