A new method has been developed that could drastically improve imaging capacity for industrial autonomous vehicles and robots. The so-called “Parallel Structured Light” method developed by Photoneo – a Slovakia-based developer of smart automation products – could pave the way for more and more accurate autonomous industrial operations in the future.
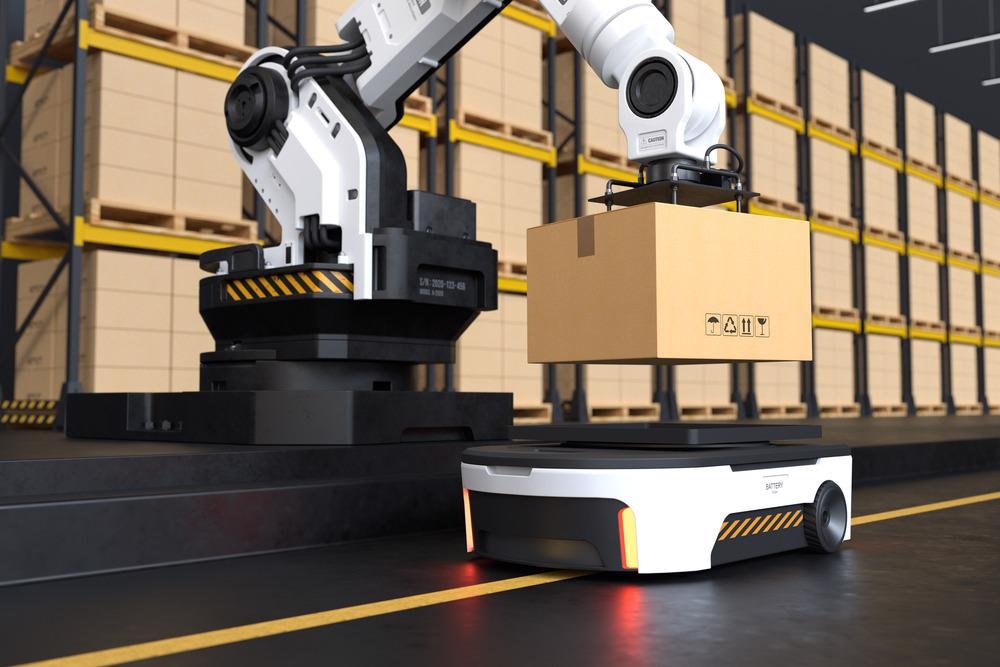
Image Credit: Es sarawuth/Shutterstock.com
Autonomous Vehicles in Industry
Autonomous vehicles are probably some way away from acceptance in the consumer market. But the manufacturing sector, often a leader in adopting new technology, is already making use of autonomous or semi-autonomous mobility to maximize output.
Approximately 10% of manufacturers in the U.S.A. use some kind of autonomous vehicle in their value chains. This is mostly made up of so-called “free range” autonomous robots (ones that are not fixed in place). Self-driving forklifts and cranes operate in many manufacturing and logistics facilities, with some even using autonomous drones.
The result – and driver – of this growing adoption of autonomous mobility is the potential for integrating with Industrial Internet-of-Things (IIoT) to create large digital twins of manufacturing processes that can be algorithmically optimized and automatically adjusted.
In surveys, early adopters of automation typically cite cost savings as the primary reason for investing in the technology. Paradoxically, most manufacturers who are not using automation technology cite cost as their main barrier to adopting it.
This paradox is mirrored often when new technology arrives in an industry or activity. Early adopters recognize the long-term benefits of initial investment and tend to reap rewards for that investment on the market.
Although late adopters bear less risk for unrealized technological potential, they also lose out on any first-mover advantage they could have had.
Seeing in Three Dimensions Still a Challenge for Automated Mobility
Machine vision is arguably the key enabling technology for moving robots, self-driving vehicles, and any technology that automates mobility. But, despite great progress in this area in the last few years, three-dimensional (3D) sensing technologies still struggle to record and reconstruct objects in motion.
Typically, manufacturers have offered a trade-off between image quality and speed. In other words, today’s 3D sensing can either have a high temporal resolution or high spatial resolution – not both.
Time-of-Flight (ToF) systems, which are based on area sensing, are good at capturing dynamic events happening quickly. But the 3D data they output is of relatively low quality.
Structure light systems like LiDAR, for example, provide good accuracy and resolution. But, as they need to send multiple frames of coded structured light patterns to work, too much movement in the scene can easily distort the 3D data output.
Using Parallel Structured Light to Overcome 3D Sensing Challenges
Developers of new technology, parallel structured light, claim to have achieved the previously unattainable goal of 3D imaging with high resolution on dynamic scenes. The method also uses a proprietary mosaic shutter type of CMOS image sensor.
Unlike structured light systems like LiDAR, parallel structured light “freezes” the scene during data acquisition to capture multiple virtual images of the scene from one sensor pulse.
This works by sending multiple light patterns simultaneously and sensing their reflections on the surrounding environment with the mosaic shutter. Super-pixel blocks in the sensor are further divided into subpixels.
This results in creating multiple structured light images in parallel rather than sequential capture used in conventional structured light methods. This parallel structured light is decoded directly in the sensor, enabling high-quality scanning that does not produce motion artifacts.
New Imaging Camera for Autonomous Mobility in Industry
The new parallel structured light technique for 3D image sensing is being implemented in a 3D camera also produced by Photoneo, the MotionCam-3D. The company claims that this camera has the highest resolution and accuracy for any area snapshot 3D camera used to capture moving objects.
Industry recognized this claim, awarding the camera many top awards such as inVision Top Innovations 2021, IERA Award 2020, Vision Systems Design 2019 Innovators Platinum, and Vision Top Innovations 2019.
The camera is capable of capturing objects moving up to 144 km/h and reconstructing them in high-quality 3D models with a resolution up to 2 Mpx and high edge detail.
It was designed for industrial environments and can withstand dust, water, and temperature variations. It has IP65 durability rating and thermal calibration. It is also resistant to vibrations and works in demanding light conditions.
Machine Vision for Autonomous Vehicles in Industry
With increased temporal and spatial resolution for machine vision, autonomous robots in industry can now see dynamic scenes and interact with them. This is essential for autonomous mobility, as moving makes all scenes dynamic.
Applications are wide and varied. Manufacturing and logistics could use better machine vision to automate bin picking, object handling, sorting, quality control, metrology, and a host of other processes in their industries.
While the parallel structured light technique was developed for industrial applications, improving machine vision will also help bring more autonomous vehicles and robots to the general public in the future.
Continue reading: Industrial Innovations with AI and Blockchain Technology.
References and Further Reading
Hoey, B. (2020). How will autonomous vehicles change manufacturing? Flexis. Available at: https://blog.flexis.com/autonomous-vehicles-manufacturing.
Photoneo (2021). Machine vision revolutionized: The 3D sensing in motion. Robotics and Automation News. Available at: https://roboticsandautomationnews.com/2021/04/19/machine-vision-revolutionized-the-3d-sensing-in-motion/42460/.
Pilkington, B. (2021). Industrial Automation: Challenges to Overcome. AZO Robotics. Available at: https://www.azorobotics.com/Article.aspx?ArticleID=425.
Disclaimer: The views expressed here are those of the author expressed in their private capacity and do not necessarily represent the views of AZoM.com Limited T/A AZoNetwork the owner and operator of this website. This disclaimer forms part of the Terms and conditions of use of this website.