AZoRobotics speaks with Alice Ruixue Ai from the University of Oslo about her efforts to create an artificial intelligence (AI)-based virtual screening algorithm and a cross-species Alzheimer’s disease (AD) drug verification system. This system could help provide a fast, cost-effective and highly accurate method for the identification of potent mitophagy inducers to maintain brain health.
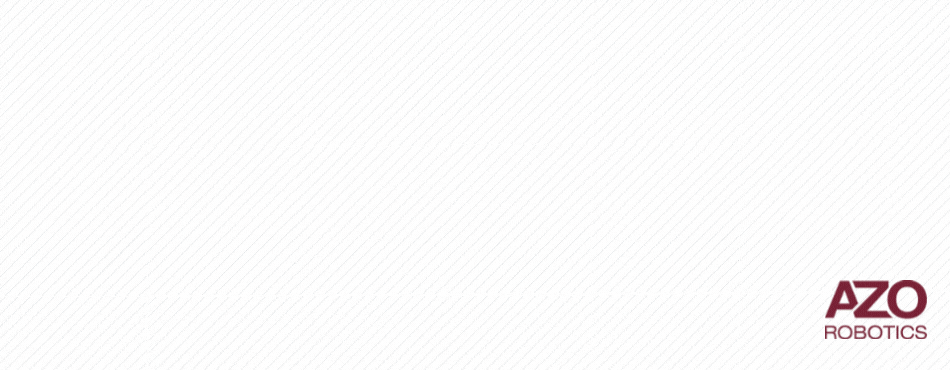
What inspired your research into using artificial intelligence (AI) to develop an Alzheimer’s disease (AD) drug verification platform?
Alzheimer’s Disease (AD) is the most common form of dementia, seen mainly in the elderly. Around 50 million people in the world suffer from dementia, and about 70% of those people have AD, so this is a huge problem for society. It is estimated that managing the health and social costs for people with AD will cost about 2 trillion dollars by the year 2030.
Many people have tried to find a cure for Alzheimer’s, and about 250 drugs have been developed over the past 15 years or so, but almost all of them failed when they were tested. Thus, my team and I were really interested in looking into how we could help these people by looking at the mechanisms that cause AD and then developing drugs to potentially help them.
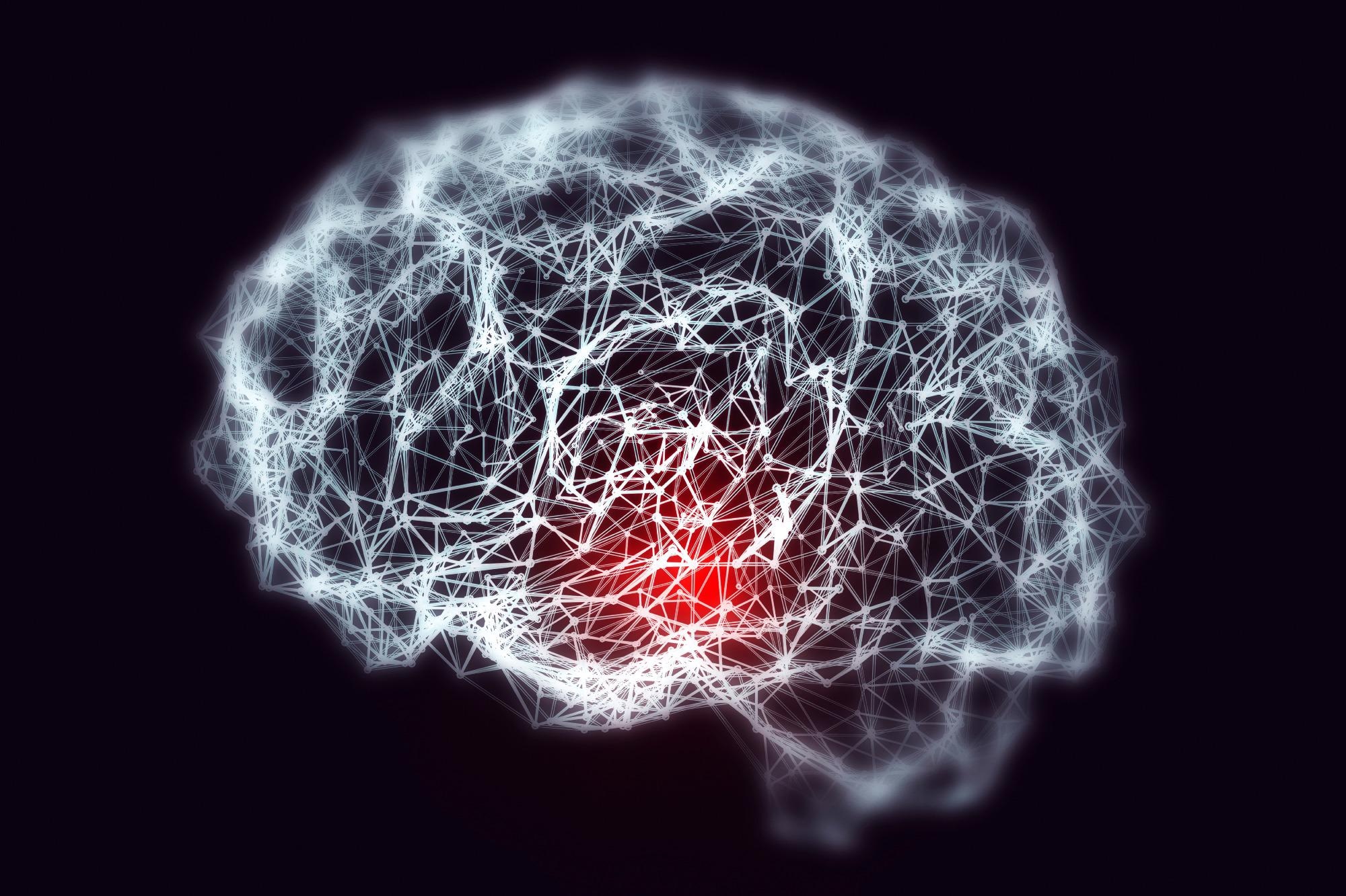
Image Credit: Shutterstock.com/Kateryna Kon
It is a great passion of ours to understand the mechanisms driving the disease because we believe that this will help us someday find a cure for AD.
When new technologies came out that allowed us to look at molecules in three dimensions, we saw huge potential for using them to help us research molecules that might help slow or stop AD. So, we have used a unique cross-discipline platform to do this that involves both 3-dimensional molecule examination and laboratory validation in model species such as worms and mice to find new candidates in a fraction of the time it used to take to do this.
The speed of this platform is really the main key to why it is so exciting. What used to take several years now takes only weeks.
Another exciting thing about our work is that we are looking through a lot of molecules that are already found in nature; we are basically just taking a second look at what nature might have to offer us in terms of compounds. The idea that nature might hold the key to controlling this devastating disease inspires us every day.
Can you give a brief overview of what causes AD and why it can be so damaging to the individual?
AD is a neurodegenerative disease, which means that it causes the connections in your brain to break down, which causes you to lose the ability to respond appropriately to your environment. It is a frightening experience for the patient and their family and ultimately leads to their death. Few individuals, around 1-5% or so, get AD from a genetic mutation(s) that is passed through their family tree. Others may develop it because of a combination of genetic risks (e.g., ApoE4/4), none-genetic factors (e.g., unhealthy diets, metabolic diseases) and environmental factors.
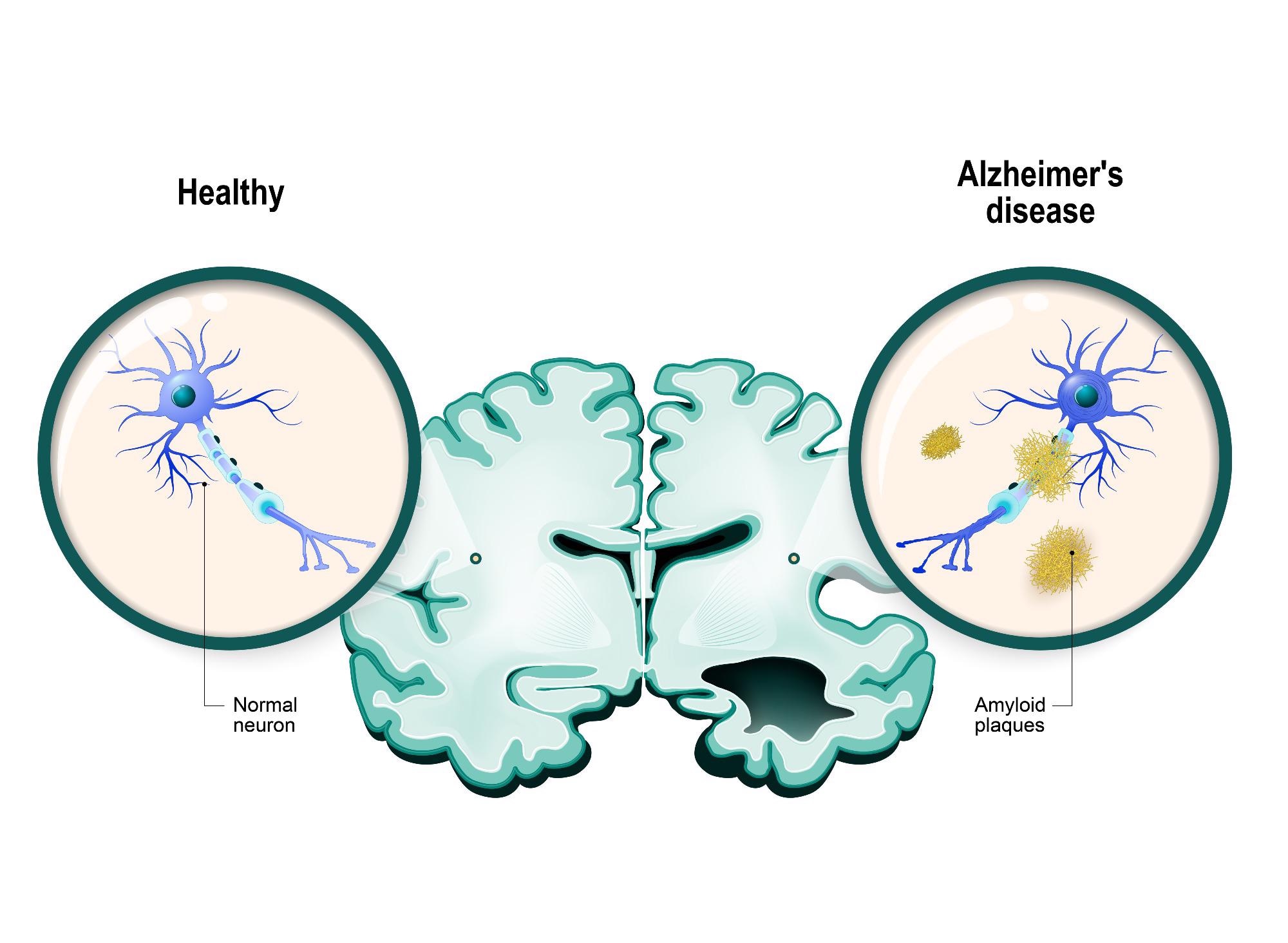
Image Credit: Shutterstock.com/Designua
Right now, we believe that AD develops when changes in the brain triggered by proteins called “amyloids” begin to build up, making the cells “sticky,” which causes the cells to become entangled and stick together. These sticky beds of cells are called “plaques.” When the cells are trapped in these plaques, they are not able to communicate, meaning that they cannot function properly.
Several changes within the cells can cause a range of problems, including inflammation of the surrounding tissues that can cause the neurons to degenerate and die. When the cells begin to die off, the brain begins to shrink, which causes breaks in the network of neurons that connect the various parts of our brain.
This process seems to start in the part of our brain that is responsible for memory, which is why the first symptom that people might notice is that they are more forgetful. As the disease progresses, it devastates more and more of the brain tissue, causing the memory to fail and issues with more and more centers, such as motor or speech function. Eventually, it leads to death, but the progression of the disease is generally a long, difficult and painful process for both the patient and their family.
Recently, we have been examining a process called autophagy, which is a normal cellular process that helps the cell to remove old components or unwanted build-ups of cellular building blocks (molecules). Specifically, it might be that the cells are not able to clear out old or broken mitochondria, which are cellular components that are responsible for producing energy in the neurons.
The removal of those broken or old mitochondria is called “mitophagy,” and it might be important in ensuring that energy levels within the cells are kept high. However, this is not completely understood yet, and we are still working on uncovering whether this process might be responsible for some or all of the problems we see in Alzheimer’s.
Roughly 50 million people worldwide suffer from dementia. Despite the prevalence of the disease, the development of AD drugs has been unsuccessful for decades. Why do you think this is?
There are a few potential reasons that this could be.
First, AD develops in people’s brains, which are not easily accessible for testing – we cannot really take samples of people’s brains while they are still using them since the brain is a very delicate organ and does not heal as muscle or skin would. Because of this, we have to use models of the disease like cultured cells in a petri dish or laboratory animals (like worms and mice) who show symptoms of AD in their pathology.
The problem is that, of course, these models are not always exactly the same. What works in a mouse or a cell in a dish may not work in a more complex system like a living human. In the past, most drugs have been focusing on preventing and/or eliminating the development of the amyloid plaques, but these have unfortunately not translated very well to the much larger human system.
Secondly, there are huge challenges when we are dealing with a disease that is affecting the brains of the patients. It may be that a drug will work well when a patient is diagnosed before the onset of symptoms, but we do not have a lot of data about this because we do not necessarily know who will develop Alzheimer’s and who will not, so we cannot selectively identify patients prior to the onset of their symptoms.
Although much research into this is ongoing, the pools of subjects are still very small, which is also restricting our ability to find the exact biomarkers that point to someone who will definitively develop AD.
Despite having few patient samples to work with, our understanding of the disease process is progressing every day, and this means that new information is coming to light that we might be able to use to develop drugs.
However, even when we find this information, it might only be a small piece of the puzzle. We need to understand all the small steps in order to understand how AD builds up in the way it does, but not all of these are exploitable. For example, a molecule might be found to be involved, but it could also be responsible for other very important cellular processes, and so turning it off would cause the cell to die or become ineffective.
Finally, drug development is a really costly, time-consuming, and uncertain process. It has been estimated that the development of a single new drug costs about $1 -2 billion from start to finish and usually takes between 10 to 20 years.
Even after that 20-year process, only about 12% of drugs that make it to the point of being tested in a clinical trial will actually end up being approved by the US FDA.
What has caused most of the drug developments to fail?
There are quite a few reasons that newly developed drugs can fail. As I said previously, the drugs can be really super effective in pre-clinical models but then fail to work when the drug is tested in humans for the first time.
Due to the difference in size between mice and humans, the effective dose of a drug in a mouse may be toxic when it is scaled up to human weight or cause terrible side effects in unrelated parts of the body. Finally, and possibly most crucially, the treatment might just come too late to make any real difference for the patient.
According to your research, enhancing mitophagy may be a novel strategy for treating AD. What is mitophagy, and why could it be a potential approach for treating AD?
Mitophagy is the selective degradation of mitochondria by autophagy. It is something that happens naturally in cells and is especially important when our bodies have been under stress, such as during sickness or recovery from an injury since this can wear out our mitochondria (the part of our cells that produce all the energy they need to do these jobs).
So when we were looking at the brain cells (neurons) under the microscope, we noticed that there was a build-up of mitochondria in the cells involved in the brain plaques. This led us to wonder whether there might be an impairment in the mitophagy process that could be causing the progression of AD. Therefore, we decided to check what happened in worms and mice when we helped them to improve their mitophagy.
As it turned out, it seemed like when we protected the mitophagy process in neurons in animal models, we were able to prevent them from developing certain biological signs of AD as well as reversing the memory impairment we would have normally seen. This led us to begin looking into the potential for the use of specific mitophagy inducer drugs, which is where the machine learning platform comes in
Machine learning is emerging as a powerful, fast, reliable and cost-effective approach to drug development. How can an AI-assisted model be helpful in anti-AD drug development?
As I said before, the drug development process has traditionally been a long and extremely expensive process. The use of machine learning techniques for developing personal drug strategies is becoming more and more popular because we can use everything known about an individual, including clinical history, transcriptomic, neuro-imaging, and biomarkers data, when we develop algorithms to examine a disease.
Deep learning using unsupervised models may be one approach to stratifying patients to reduce some of the complexity we sometimes see in high-dimensional information like health data, which could help us to identify different therapies for different patients. For example, patients with different subtypes of AD might be able to be identified, and these subtypes could, in turn, show us paths toward the development of strategies for personalized medicine.
For example, using this evidence, physicians may prescribe single-drug or combination drugs to an individual patient to improve treatment effects.
AI could also be a new way to design or discover new drugs for AD. As mentioned before, AD pathology involves a vast array of mechanisms. Exploring the data related to these mechanisms in an efficient, holistic and thorough manner is key to understanding AD. However, this can be a challenge for individual researchers because it is a time- and resource-intensive process.
AI can help make sense of vast amounts of data, predict the efficacy of therapies or even help us design new drugs. AI is able to produce results in a fraction of the time that human researchers need. For example, knowledge graphs, which link genes, diseases and drugs, are built using AI to integrate different data types, including huge chemical and protein pathway databases and even scientific papers.
This approach can highlight the less-obvious links between biological targets and AD. For example, by extracting gene expression data from healthy control and individual patients’ gene expression data, molecular networks can visualize biological processes that change in different disease stages.
For instance, a combination of Bayesian inference, clustering and co-regulation was used to analyze transcriptomic data collected from the brain tissue of individuals with late-onset AD and non-AD controls. A group of microglial-specific genes, coding the TYROP protein and immune-related genes, were found to be highly expressed in late-onset AD patients. After further verifying the function of TYROP in the AD mouse model, researchers found a deficiency of this protein, showing a neuroprotective function. This significantly reduced the time needed to identify a new potential target for drug therapy.
Your team utilized machine learning to provide a potential solution for drug discovery. Can you give an overview of how machine learning aided the discovery of two compounds with anti-AD potential?
Led by my supervisor, Associate Professor Evandro Fei Fang, with collaborators, we have developed a novel ‘Fang-AI’ platform for drug discovery focusing on mitophagy induction. The machine learning model we created uses what is called a chemical “fingerprint” to search through databases of known molecules in order to identify those that might be effective in the treatment of AD.
Specifically, we wanted to see whether we could identify a molecule that could improve the rate of mitophagy in the brain to help cells clear the backlog of spent mitochondria and improve the biological function of neurons in the brain.
We hypothesized that this might help the brain to stop or even reverse the problems seen with the accumulation of plaques. To create the screening technology, we used molecular representation approaches, including Mol2vec, pharmacophore fingerprint and 3D conformers fingerprint to create a sort of digital signature for each potential molecule.
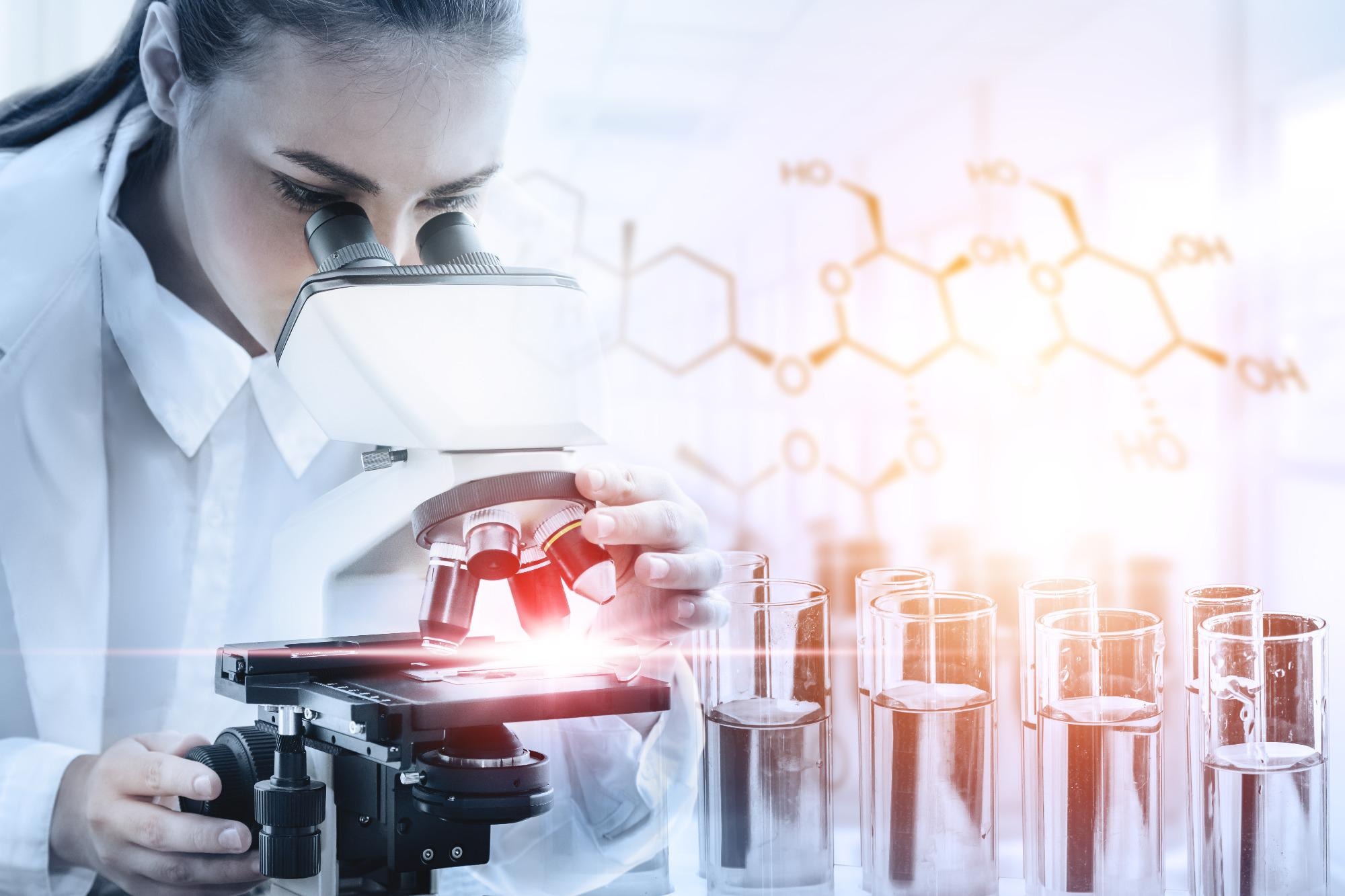
Image Credit: Shutterstock.com/Blue Planet Studio
We started by creating a large pre-training dataset using compounds retrieved from the ChEMBL and ZINC natural products databases that had 19.9 million potential compounds in it after a first pass-through for relevance.
Molecules were represented using a text-based annotation system called SMILES (Simplified, Molecular Input Line System). Representations were created for these compounds using software packages called RDKit and Word2vec. Pharmacore (2D) and conformers (3D) fingerprints, two more ways of translating 3D molecules into strings, were generated, and the resulting dataset was used for training the natural language processing model.
Structural distances between the target molecules (known mitophagy inducers) and each of the compounds in the database were calculated to create a model. We then took the trained model and applied it to a dataset consisting of 3,274 plant-based natural products used in traditional Chinese medicine.
Similarity scores were calculated, and the top compounds were selected. From these 3,274 compounds, 18 scored over a cut-off threshold for similarity and were selected for further study. Although 18 may seem like a small number when compared to the original 3,274 possibilities, this method was able to increase the success rate from 0.01% -10.00% to 44%, with 8 of the 18 compounds showing some promise.
We then took those 18 compounds and tested them in the lab, and from those 18, eight really showed some promise. After testing, we found that three of the compounds showed significant biological effects, while the rest were discounted.
After further testing, two of the compounds were found to have strong, statistically relevant effects on the rate of mitophagy, with the models showing improvements in neural function when compared to those that did not receive treatment with the molecules.
What are the potential benefits of the two compounds with anti-AD properties, and how could they be used to improve memory and cognitive impairments?
Studies in cells and AD laboratory animal models (worms and mice) showed that our lead compounds K and R improved memory and reduced AD pathologies. As the mouse data was generated via ‘oral gavage,’ it can be concluded that these compounds could be given to patients as a ‘pill/capsule’ if they prove to be equally effective in clinical trials.
How successful is your AI-driven virtual screening recommendation?
When you consider what the rate of success was in the past (0.01 – 10.00%), our rate of success of 44% is actually very impressive, and we were able to achieve this with a minimum of expense and time.
Our combinational approach provides a fast, cost-effective and highly accurate method for the identification of potent mitophagy inducers to maintain brain health.
We think that this method will really help with the quick, effective identification of new, potentially helpful drug candidates, as well as assisting with the reduction of ‘false positive’ candidates, which could potentially speed up the discovery of new drugs while keeping research costs lower.
Recently, the scope of AI has moved from sheer theoretical knowledge to real-world applications. What is next for AI in terms of drug development for aging and various diseases?
Even though the quality of clinical technologies has improved in the 21st century, a dramatic increase in lifespan and, correspondingly, an increasingly aged population is expected. Since the elderly population is more susceptible to disease, infection and neurodegenerative diseases (like AD), we foresee an increase in pressure on both society and the healthcare system. Thus, it is timely and necessary to apply AI in clinical decision-making.
In recent years, AI applications have been widely used in precision medicine, including AI diagnosis, prognosis and drug development. AI-aided medical applications have not only supported doctors, researchers and scientists in increasing efficiency and providing decision-making support but have also accelerated the development of the medical and healthcare industry.
With more and more breakthroughs, such as DeepMind’s AlphaFold2 (a program that uses AI to predict how proteins fold), we anticipate the time when AI will begin to revolutionize the drug development and disease diagnosis industry is near.
The next big advances will bring much tighter integration with automation that allows us to move from an augmented drug design platform where the design chemist makes all the decisions to an autonomous drug design platform where the system can autonomously decide which compound to make next.
What impact will such a machine learning approach have on the industry?
As stated previously, the introduction of more machine learning technology will improve and speed up the process of drug discovery.
In terms of what it could really do to the industry, it could prove to be an effective building block, speeding up discovery and thus shortening the pathway from knowledge to the production of a new drug. This could lead to an increase in the number of potential drugs being tested and thus to an increase in the potential therapies on offer for patients.
What are the next steps for your research?
This is really new technology, and so, as with all new tech, we would like to continue to look into the potential to improve the performance of the system to give us more confidence in its efficacy.
Further to this, though, we believe that this technology can be applied to other existing issues, such as looking at the potential to create a “designer molecule” that will lock into the mitophagy machinery, helping it to work more effectively. Since this is just the beginning of our journey, the potential applications are almost endless at this point.
About Alice Ruixue Ai
Alice Ruixue Ai is a student at the University of Oslo (UiO) and the Akershus University Hospital. In Assoc. Prof. Evandro F. Fang’s lab, she is working on the mechanisms of impaired mitophagy in aging and age-predisposed Alzheimer’s disease; with collaborators, she is applying artificial intelligence on screening and designing of drug candidates for aging and Alzheimer’s disease. Before joining the Fang lab, she studied for a master’s degree in Oral Medicine at Sichuan University, China, and published four papers in international peer-reviewed journals in the 3-year master program. For career development, she aims to become a physician-scientist working on healthy oral and brain aging in the next 7-10 years.
Disclaimer: The views expressed here are those of the interviewee and do not necessarily represent the views of AZoM.com Limited (T/A) AZoNetwork, the owner and operator of this website. This disclaimer forms part of the Terms and Conditions of use of this website.