Surface inspection is crucial to ensure product quality. In recent decades, several advances have been made in accurate determination of product surfaces and quality. This article will explore how the emerging field of machine learning (ML) could advance surface inspection and metrology.
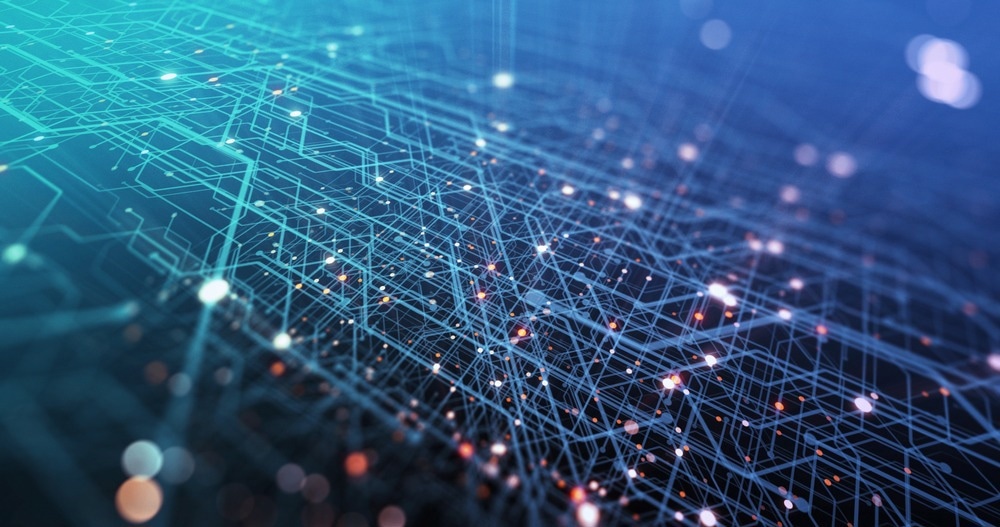
Image Credit: Quardia/Shutterstock.com
Issues with Conventional Manual Surface Inspection
Surface inspection is a key stage in manufacturing to identify any surface defects which may impact the quality and performance of the final product. Historically, human inspectors have assessed surface quality by eye, but this is a laborious and time-consuming process that often misses miniscule surface defects.
Although skilled inspectors are able to conduct metrology assessment with high efficiency and accuracy, errors persist. Research has indicated that visual inspection errors range between twenty and thirty percent, either due to human error or space constraint.
There are two types of visual inspection error: missing a defect or producing a false positive. Of these two error types, misses occur more frequently. Missing an extant error can lead to quality loss, whereas incorrectly identifying false positives leads to increased production cost, time, and overall reductions in yield.
Manual inspection can produce errors due to a number of limitations, such as the untrustworthiness of vision, the inability of the human eye to make precise measurements on a small scale, and operator costs. To overcome this, new and more accurate methods are needed.
Automated Surface Inspection and Surface Metrology
Due to the limitations of manual surface inspection, automated surface inspection methods have become more commonplace over the past several decades. Both contact and non-contact methods have been developed to improve this vital part of the manufacturing process.
Contact methods include using diamond-tipped control devices to detect micro- and nano-scale imperfections. X-rays, ultrasound, and gamma rays are some of the non-contact methods commonly used in automated surface inspection.
At the core of automated systems are technologies such as sensors, cameras, and computer software. Images are processed digitally by computers. Inspection data can be compared to pre-existing reference images or target values to determine if a piece is faulty. Lasers can also be used to build a 3D image for testing.
Surface metrology is a well-established field of visual surface inspection which uses both contact and non-contact methods to detect minute defects. Profilometers are used in contact metrology, with non-contact methods including digital holography, interferometry, confocal microscopy, electron microscopy, and non-contact profilometers.
The desired measurement scale determines the appropriate choice of method and equipment used in surface metrology. Additionally, the probe used can be a physical filter which can limit measurement accuracy, especially if the stylus tip is too large to reach the bottom of a defect trough.
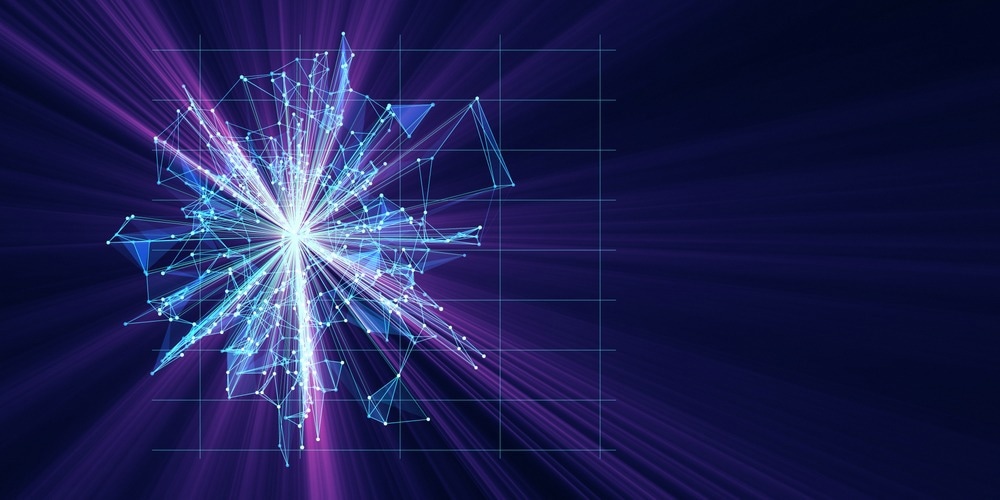
Image Credit: Anatolii Stoiko/Shutterstock.com
Utilizing Machine Learning to Accelerate Surface Inspection
Real surface geometry is a complex microenvironment which can produce vast amounts of data points. Moreover, accurate and fast surface inspection and metrology requires high-throughput methods and data analysis.
AI-based methods have seen accelerated adoption across multiple industries and research areas in the past decade as technologies evolve and mature and make their way out of the laboratory into the real world.
Machine learning is fast becoming an essential tool in automated surface inspection for many different products, especially for more complex inspections. It is highly effective at learning complex defects, building a vast library of data from previous inspections which it can learn from and improve future inspections.
Machine learning differs from traditional machine vision as it can conceptualize and generalize the appearance of a component more effectively. It can handle variations that would otherwise require human knowledge to fully conceptualize such as changes in shading, distortion, light levels, and texture.
Production and process constraints can produce parts with varying sizes, shapes, brightness, and contrast, which traditional machine vision struggles to identify, but machine learning can amply handle. Due to these key benefits, machine learning is being increasingly deployed in multiple industries for surface metrology.
Incorporating machine learning into smart sensors is a fast-growing element in product assembly line inspection to complement conventional inspection techniques, detecting defects which would otherwise be missed, affecting final product quality, aesthetics, and performance.
Research in 2016 into convolutional neural networks (CNNs) for this vital stage of product manufacture revealed several benefits. A single network can be applied to different types of surfaces for defect detection and, moreover, the proposed machine learning method provides time, cost, and energy saving advantages.
Research such as this has demonstrated the many advantages of machine learning-based surface inspection and metrology over conventional manual inspection and machine vision techniques.
The Future of Automated Surface Inspection and Metrology
AI, machine learning, deep learning, and advanced neural networks have been largely touted as the future of many industrial sectors, conferring huge benefits over manual and current automated methods.
Manufacturing requires the accurate and rapid determination of multiple complex surface defects to ensure consistent product quality. While offering a brief overview, this article has explored how machine learning will benefit surface inspection in the coming years, significantly improving product quality.
References and Further Reading
Park, J.K et al. (2016) Machine learning-based imaging system for surface defect inspection. Int. J. of Precis. Eng. and Manuf.-Green Tech., 3, pp. 303–310 https://link.springer.com/article/10.1007/s40684-016-0039-x
Gao, W et al. (2019) On-machine and in-process surface metrology for precision manufacturing. CIRP Annals 68(2) pp. 843-866 https://doi.org/10.1016/j.cirp.2019.05.005
Industrial Vision (website) Surface Inspection [Online] industrialvision.co.uk. Available at: https://www.industrialvision.co.uk/applications/surface-inspection
Disclaimer: The views expressed here are those of the author expressed in their private capacity and do not necessarily represent the views of AZoM.com Limited T/A AZoNetwork the owner and operator of this website. This disclaimer forms part of the Terms and conditions of use of this website.