AI is actively reshaping how research gets done.
What started with symbolic logic has grown into something far more powerful, thanks to advances in machine learning and deep learning. Machines can now learn from data, adapt, and handle complex tasks that once needed human intuition.
For research institutions, this shift opens up some exciting possibilities: faster discovery, smoother operations, and room for entirely new kinds of innovation.1
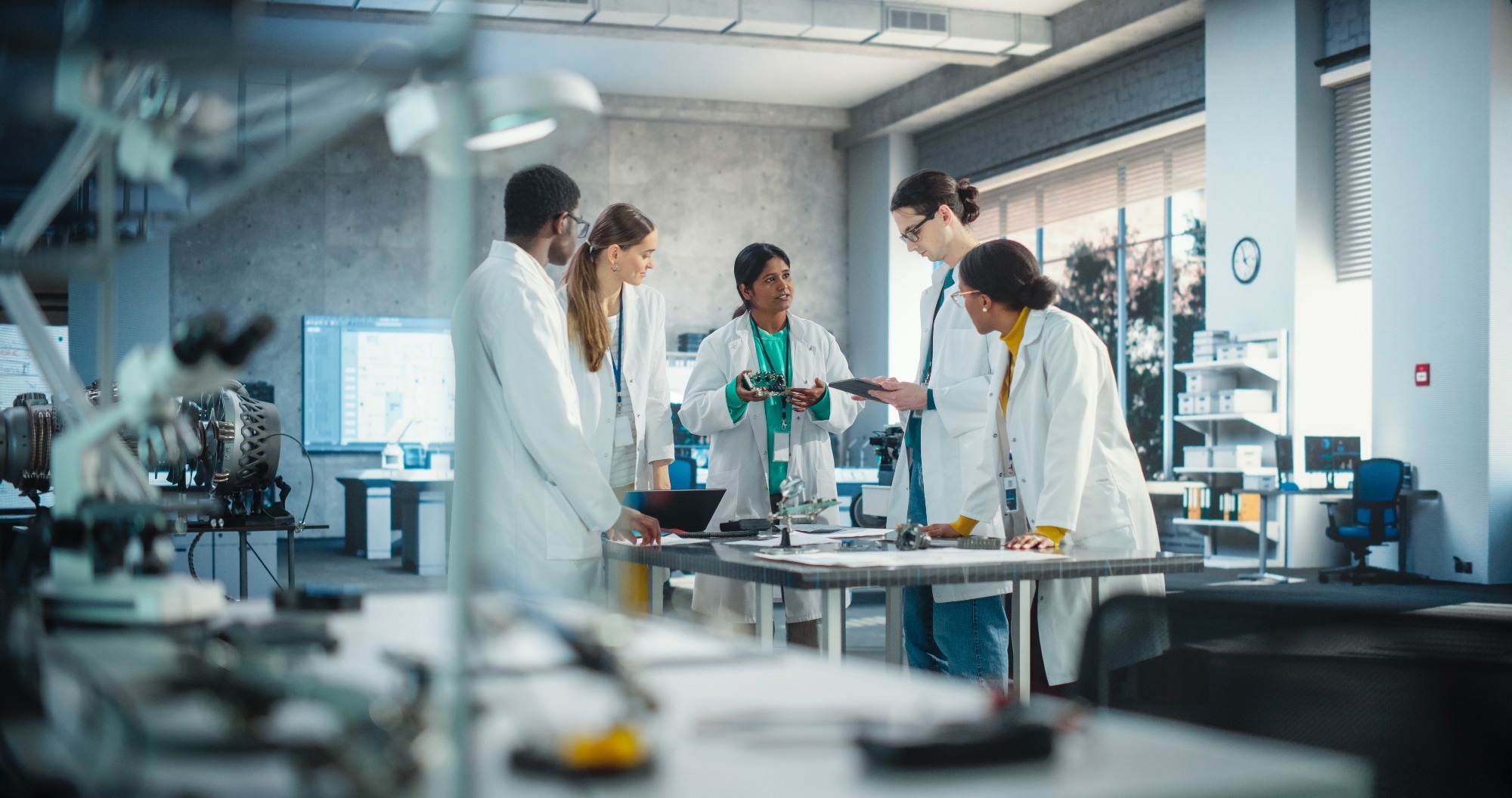
Image Credit: Gorodenkoff/Shutterstock.com
If you're part of a university, public research center, or non-profit R&D group and you’re thinking about how to bring AI into your lab infrastructure, this guide is for you. We’ll walk through what it takes to plan, fund, and launch an AI-powered lab—and how doing so can help your institution boost its research game, draw in top-tier talent, and stay competitive.
Download your PDF copy now!
Why AI Labs Matter for Institutions
Let’s start with the “why.” AI labs are quickly moving from a “nice to have” to a “must have” for research institutions. They pull together the right tools, talent, and data in one place, making it easier to run experiments, collaborate, and get reliable results at scale. With access to high-performance computing, shared datasets, and smart tools, researchers can work more efficiently and with greater consistency.
A good example of this in action is New York University’s collaboration with Facebook AI Research. By integrating AI into their workflows, they were able to significantly cut down MRI processing times. That kind of leap forward isn’t just impressive; it’s quite literally transforming the pace of discovery.
AI labs also help institutions stand out. As AI spreads across disciplines, having a dedicated lab sends a signal that this is a place where innovation happens. It helps with funding, recruiting, and industry partnerships. Just look at the University of Rhode Island’s AI Lab. Funded through a mix of grants and institutional support, it’s become a hub for emerging research and collaboration.
And then there’s the interdisciplinary magic. Modern problems—like climate modeling or genomics—don’t fit neatly into one department. They need engineers, philosophers, data scientists, and more, all working together. URI’s AI Lab, which sits inside its main library, is designed exactly for that. It brings together people from different fields and lets them tackle big questions using shared tools and data. That kind of collaboration is hard to pull off without dedicated AI infrastructure.1,2
How to Know if You're Ready
Before you dive in, it’s important to figure out if your institution is truly ready to build and support an AI lab. Start by taking stock of what you already have; your infrastructure, your data, and your people. A lot of organizations underestimate how demanding AI projects can be. Basic IT systems and patchy datasets won’t cut it. You need powerful computing. Think GPUs or access to scalable cloud services, as well as high-quality, well-organized data.
Ask yourself: Is your data stored in standardized, accessible formats? Can your IT systems handle AI workloads? Do you have staff who know how to build, train, and manage AI models? Many smaller institutions, including SMEs, encounter roadblocks here, such as legacy systems, scattered data, or a shortage of AI-literate staff.
Next, look at where AI can actually make a difference. Not every process benefits from automation. Your best bets are the repetitive, data-heavy tasks where AI can either save time or improve accuracy. But success isn’t just about picking the right use case, it’s about making sure the project fits your broader goals.
This is where frameworks like TOEH (Technology-Organization-Environment-Human) come in handy. They help you assess your readiness from multiple angles, including strategic alignment, organizational structure, and ethics. Taking a structured approach here helps you spot risks early, like data privacy concerns or workforce gaps, so you can prioritize the most impactful projects and move forward with confidence.3,4
What Goes Into an AI Lab?
An effective AI lab isn’t just a room full of computers; it’s a carefully built environment that supports both automation and human decision-making.
Let’s talk automation first. AI-enabled robots can take over repetitive lab tasks like handling samples, recording measurements, and logging data. These aren’t just expensive industrial robots either. Affordable robotic arms with machine vision and adaptable grippers can now handle precise workflows, reducing error and making research more scalable and reproducible.
Then there’s the AI software side. Tools powered by reinforcement learning, multi-task learning, or even physics-informed models help researchers interpret data, run simulations, and design experiments. Virtual labs (VLs) that pair domain-specific digital twins with general-purpose AI are especially powerful—they let researchers test hypotheses digitally before running physical experiments.
Managing all this data is no small feat, either. AI labs rely on modern data platforms (MDPs) that can handle a wide range of structured and unstructured data. These platforms need to be secure, scalable, and built with FAIR principles in mind, so that data stays findable, accessible, interoperable, and reusable. A good MDP doesn’t just store data; it helps people share insights, collaborate across disciplines, and generate new knowledge in real time.
And of course, the human side matters. The best AI labs support intuitive, transparent interaction between people and machines. Large language models (LLMs) can act as conversational assistants, turning plain language into structured commands. But they don’t replace researchers. Humans still need to guide decisions, catch errors, and validate outcomes. Features like workflow playback and task tracking can make it easier to stay in control while letting AI do the heavy lifting.5,6,7
How to Make it Happen
Launching an AI lab is a big lift. It involves new tech, new ways of working, and sometimes, a whole new mindset. A structured rollout helps keep things on track.
One proven approach is to use a mixed-methods design. Start with a literature review to understand what’s worked (and what hasn’t) in other labs, then talk to your stakeholders—faculty, IT, administrators—to get real-world insights. From there, break the process down into phases: planning, implementation, and long-term maintenance.
Twelve core concepts usually come into play here: leadership, buy-in, change management, engagement, workflow, finances, HR, legal, training, data, monitoring, and ethics.
Leadership sets the tone. You need champions who can articulate a clear vision and rally support. Change management smooths the path by addressing concerns and making sure people know what’s coming. Cross-functional engagement ensures you’re building a lab that meets real needs, not just what looks good on paper.
Workflow integration is where a lot of projects hit snags. The key is to make AI support existing processes, not disrupt them. Legal and financial questions should be handled early, and you’ll want ongoing training programs to keep skills fresh. Data governance is crucial for long-term trust and usability. And don’t overlook ethics—bias, transparency, and responsible use need to be baked into your strategy from the start.
Support systems like helpdesks and governance committees can make a big difference in day-to-day operations. They provide structure and continuity as the lab grows and evolves.8,9
The Roadblocks You'll Likely Hit
Of course, it’s not all smooth sailing. Budget and procurement are two of the biggest hurdles. AI-ready hardware, like GPU clusters or NVIDIA DGX stations, isn’t cheap. Even once you have the funds, long lead times for equipment (or facility upgrades like air-conditioning to handle heat loads) can slow everything down.
Approval processes can be just as frustrating. You might need buy-in from multiple decision-makers—hospital CEOs, university deans, grant managers—each with different priorities. Advocating for the long-term research benefits of your lab is key to moving things forward.
Cultural resistance is another big one. Some clinicians or IT staff may be wary of AI, worried about job loss, workflow changes, or reliability. The most successful labs don’t ignore this. They invest in transparency, holding monthly check-ins, offering hands-on demos, and building a shared understanding of what the AI is (and isn’t) doing. But even with buy-in, surprises happen. A Ph.D. student leaving mid-project, for instance, can throw a wrench in your timeline. Resilient team structures and solid documentation help reduce that risk.
Data access and security might be the biggest wildcard. Getting institutional approval to use patient data, even for retrospective studies, can take months of back-and-forth with IRBs and IT departments. One lab described intense resistance from hospital IT, which lacked the infrastructure to meet research needs. During the pandemic, remote work added another layer of complexity, with VPNs and access protocols slowing progress even further.10
What You Get in Return
Despite all the effort and roadblocks, AI labs deliver real value. They speed up research, encourage cross-disciplinary collaboration, and improve the reproducibility of results. With the right tools in place—like foundation models, automated documentation, and multi-cloud computing—researchers can get to insights faster and more reliably.
You can already see this in tools like IBM RXN (for chemical synthesis), GT4SD (for generative science), and HyperTaste (for sensing and analysis). These platforms are helping researchers form hypotheses, run experiments, and publish results more efficiently, all while pushing the boundaries of what’s possible in their fields.11
Want to Learn More?
If this article has taken your interest, then why not check out some of the topics below?
Download your PDF copy now!
References and Further Reading
- Kim, B. (2019). AI and Creating the First Multidisciplinary AI Lab. Technical Services Department Faculty Publications.
https://digitalcommons.uri.edu/lib_ts_pubs/115/
- Hoffman, S. G. (2016). Managing Ambiguities at the Edge of Knowledge. Science, Technology, & Human Values, 42(4), 703–740. DOI: 10.1177/0162243916687038
https://journals.sagepub.com/doi/abs/10.1177/0162243916687038
- Saqib Naheed, Pinto, R., & Fabiana Pirola. (2025). A Preliminary multidimensional AI readiness assessment model for SME’s. Procedia Computer Science, 253, 774–783. DOI:10.1016/j.procs.2025.01.139
https://www.sciencedirect.com/science/article/pii/S1877050925001474
- Jöhnk, J., Weißert, M., & Wyrtki, K. (2020). Ready or Not, AI Comes— An Interview Study of Organizational AI Readiness Factors. Business & Information Systems Engineering, 63(1), 5–20. DOI:10.1007/s12599-020-00676-7
https://link.springer.com/article/10.1007/s12599-020-00676-7#Abs1
- Conrad, S., Auth, P., Masselter, T. and Speck, T. (2025), Lowering the Entrance Hurdle for Lab Automation: An Artificial Intelligence-Supported, Interactive Robotic Arm for Automated, Repeated Testing Procedures. Adv. Intell. Syst. 2401086. DOI:10.1002/aisy.202401086
https://advanced.onlinelibrary.wiley.com/doi/full/10.1002/aisy.202401086
- Klami A, Damoulas T, Engkvist O, Rinke P, Kaski S. Virtual laboratories: transforming research with AI. Data-Centric Engineering. 2024. DOI:10.1017/dce.2024.15
https://www.cambridge.org/core/journals/data-centric-engineering/article/virtual-laboratories-transforming-research-with-ai/F7F2E796AE8A3E9FFF345F6C10CA6992
- Ortega-Calvo, A. S., Morcillo-Jimenez, R., Fernandez-Basso, C., Gutiérrez-Batista, K., Vila, M., & Martin-Bautista, M. J. (2023). AIMDP: An Artificial Intelligence Modern Data Platform. Use case for Spanish national health service data silo. Future Generation Computer Systems, 143, 248–264. DOI:10.1016/j.future.2023.02.002
https://www.sciencedirect.com/science/article/abs/pii/S0167739X23000432
- Nair, M., Svedberg, P., Larsson, I., & Nygren, J. M. (2024). A comprehensive overview of barriers and strategies for AI implementation in healthcare: Mixed-method design. PLoS ONE, 19(8), e0305949–e0305949. DOI:10.1371/journal.pone.0305949
https://journals.plos.org/plosone/article?id=10.1371/journal.pone.0305949#sec048
- Reim, W., Åström, J., & Eriksson, O. (2020). Implementation of Artificial Intelligence (AI): A Roadmap for Business Model Innovation. AI, 1(2), 180–191. DOI:10.3390/ai1020011
https://www.mdpi.com/2673-2688/1/2/11
- Hergan, K. (2022). “Challenges implementing and running an AI-Lab: Experience and Literature Review.” Biomedical Journal of Scientific & Technical Research, 45(4). DOI:10.26717/bjstr.2022.45.007222
https://biomedres.us/fulltexts/BJSTR.MS.ID.007222.php
- AI for Scientific Discovery (2021). IBM Research. Available at:
https://research.ibm.com/projects/ai-for-scientific-discovery (Accessed on 13th May 2025)
Disclaimer: The views expressed here are those of the author expressed in their private capacity and do not necessarily represent the views of AZoM.com Limited T/A AZoNetwork the owner and operator of this website. This disclaimer forms part of the Terms and conditions of use of this website.