Jun 11 2020
Although feedforward mechanisms employed to manipulate machines are considerably more precise compared to feedback mechanisms, they could be computationally difficult at the same time.
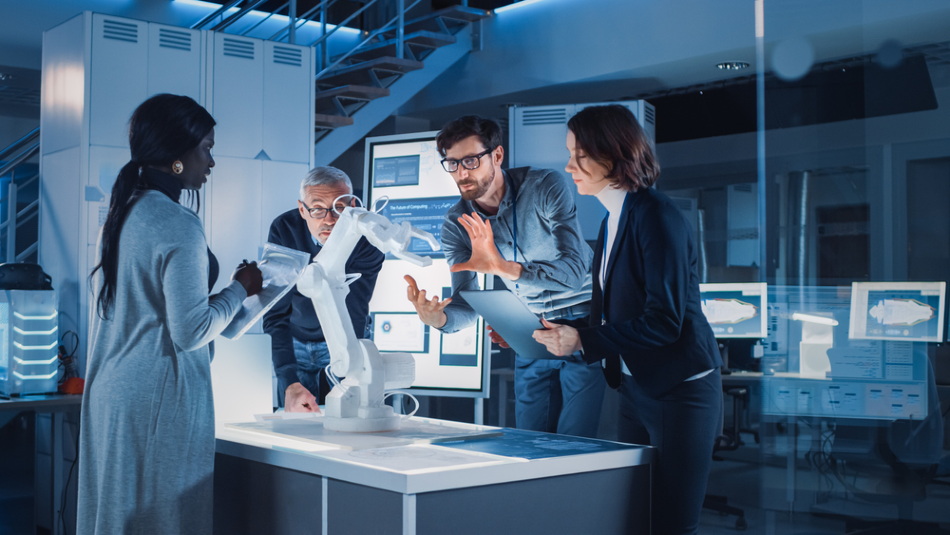
Image Credit: Gorodenkoff/Shutterstock.com
A new technique has enhanced traditional methods and would soon be tried on machine vision and industrial robots.
A majority of the people will be familiar with “feedback,” where a machine like thermostat adjusts itself after noting an error in performance. For instance, as soon as the temperature exceeds a preset point, the heating system of the building turns off.
However, feedback systems can be crude control mechanisms: in case the upper-temperature limit is set very high, then room temperature can still start to feel unpleasant just before the heating is turned off.
By contrast, if the desired temperature range is set very narrow—probably just a few degrees away—then the heating system will continuously be turned on and off as it goes in and out of range, thereby increasing wear and tear.
In general, people are not much aware of “feedforward” control systems, but they manage to overcome the shortcomings of feedback mechanisms by quantifying variations in the entire system in which a specified machine operates and making the machine to consider such changes before they can have an adverse effect.
For example, a baseball player attempting to catch a ball is engaged in feedfoward control. As she tracks a ball’s trajectory, she adjusts her movement in anticipation of where it will land.
Dailin Zhang, Engineer, Huazhong University of Science and Technology
Consequently, feedforward is highly precise compared to feedback. A thermostat working on feedforward control may sense the opening of a door and thus turn on the heating system before the temperature within the home can go down.
However, there are disadvantages in the system: Feedforwards need better knowledge, or “modeling,” of the system in which they are functioning. If the thermostat tracks the door to check if it is open while missing the windows, it implies that it has a bad “model” of the house, and it will get too cold.
The brains of humans and other animals frequently practice feedforward control since they are very good at whole-system modeling. However, in the case of machines, this modeling is computationally difficult.
But scientists from the Huazhong University of Science and Technology and the University of California at Berkeley have designed a new feedforward technique that enhances traditional feedforward methods.
In general, the parameters fed into such a technique cannot be changed (for instance, the probability of a window or door opening). However, the new method acquires these parameters from a doubtful surrounding to design an updated model of the system in real-time through a set of measures.
The method was tested on a simulation of a motor, and the team identified that the method certainly realized enhanced performance compared to the conventional feedforward compensation.
Each one in the team is an expert in machine vision and enhancing the calibration of industrial robots. As a next step, the researchers intend to trial their method on real-world challenges.
Journal Reference:
Zhang, D., et al. (2020) A Variable-Parameter-Model-Based Feedforward Compensation Method for Tracking Control. IEEE/CAA Journal of Automatica Sinica. doi.org/10.1109/JAS.2020.1003135.