Jul 3 2020
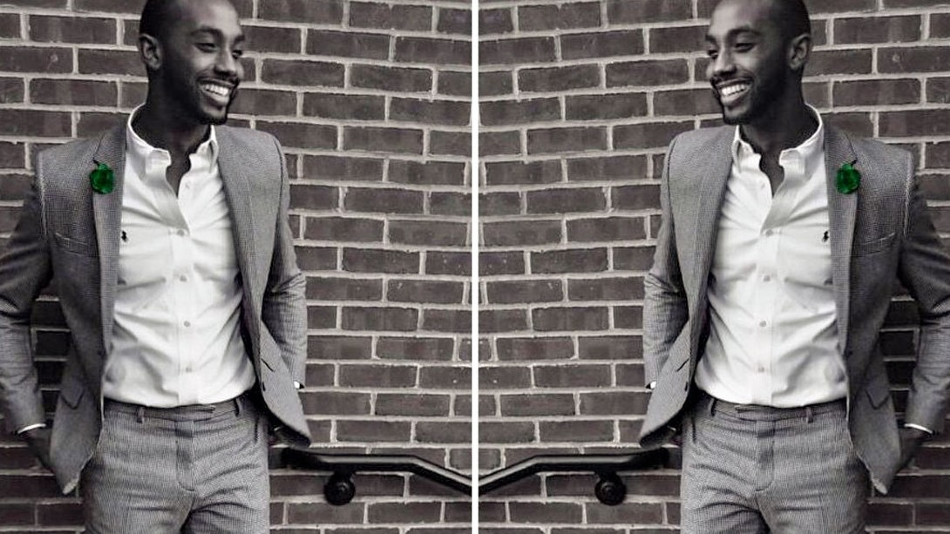
AI learns to pick up on unexpected clues to differentiate original images from their reflections, the researchers found. Image Credit: Cornell University.
When it comes to mirrors, things are not the same. For example, clocks run in counterclockwise directions, text appears backward, right hands become left hands, and vehicles drive on the wrong side of the road.
Fascinated by how images are changed by reflections in both subtle and not-so-subtle ways, researchers from Cornell University employed artificial intelligence (AI) to analyze the difference between original images and their reflections.
The algorithms developed by the researchers learned to detect surprising clues, like the direction of gaze, hair parts, and, amazingly, beards. These findings show promise for identifying faked images and for training machine learning models.
The universe is not symmetrical. If you flip an image, there are differences. I’m intrigued by the discoveries you can make with new ways of gleaning information.
Noah Snavely, Study Senior Author and Associate Professor of Computer Science, Cornell Tech
The study titled “Visual Chirality,” was presented at the 2020 Conference on Computer Vision and Pattern Recognition, conducted virtually from June 14th to 19th, 2020.
Zhiqui Lin is the first author of the study. Abe Davis, an assistant professor of computer science, and Jin Sun, a postdoctoral researcher at Cornell Tech, are the study’s co-authors.
Snavely added that AI can easily differentiate between reflections and original images and this fact is rather surprising. In fact, a rudimentary deep learning algorithm can rapidly learn to categorize a flipped image with a precision of 60% to 90%, based on the types of images used for training the algorithm. Most of the clues picked up by the algorithm cannot be easily noticed by humans.
In this analysis, the researchers devised a technology to produce a heat map indicating the portions of the image that are important to the algorithm. The aim was to find out how these decisions are made by the algorithm.
Not surprisingly, the researchers discovered that text was the most commonly used clue and this text appears different backward in each written language.
To further understand this concept, the researchers eliminated images with text from their set of data and observed that the subsequent set of properties that were focused by the model included phones, faces, shirt collars (buttons tend to be on the left side), and wristwatches—which a majority of the people are likely to carry in their right hands—and also other factors that expose right-handedness.
The team was fascinated by the tendency of the algorithm to focus on faces, which do not appear to be evidently asymmetrical. “In some ways, it left more questions than answers,” added Snavely.
The researchers subsequently performed another study but this time they focused on faces. They discovered that areas such as hair part, beards, and eye gaze—a majority of the people, for reasons unknown to the scientists, gaze to the left in portrait photos—are illuminated by the heat map.
Snavely added that he and his team members are clueless about the kind of information detected by the algorithm in beards, but they assumed that the way individuals shave or comb their faces could expose handedness.
It’s a form of visual discovery. If you can run machine learning at scale on millions and millions of images, maybe you can start to discover new facts about the world.
Noah Snavely, Study Senior Author and Associate Professor of Computer Science, Cornell Tech
While each of these clues may not be reliable at an individual level, the algorithm has the ability to instill more confidence by integrating numerous clues, revealed the findings. The scientists also noted that the algorithm employs low-level signals, arising from the way images are processed by cameras, to make its decisions.
While more research is required, the results may influence the training strategy of the machine learning models. Such models require a large number of images to learn how to categorize and detect images, and this is the reason why computer scientists usually employ reflections of prevalent images to effectively increase their datasets by two-fold.
Analyzing how such reflected images vary from the originals may provide data about the potential biases in machine learning that may lead to incorrect results, added Snavely.
This leads to an open question for the computer vision community, which is, when is it OK to do this flipping to augment your dataset, and when is it not OK? I’m hoping this will get people to think more about these questions and start to develop tools to understand how it’s biasing the algorithm.
Noah Snavely, Study Senior Author and Associate Professor of Computer Science, Cornell Tech
By interpreting how reflection alters an image, AI could be used for identifying images that have been doctored or faked—a problem of greater concern on the internet.
“This is perhaps a new tool or insight that can be used in the universe of image forensics if you want to tell if something is real or not,” concluded Snavely.
The study was partly funded by philanthropists Eric Schmidt, former CEO of Google, and Wendy Schmidt.
Visual Chirality: How Is Our World Different From What We See in the Mirror?
Video Credit: Abe Davis.