Reviewed by Mila PereraOct 26 2022
Artificial intelligence (AI) makes it possible for people worldwide to personally access vital medical screening. Jingtong Hu, an Engineering Researcher at the University of Pittsburgh, is involved in ensuring that the screening is fair and effective, regardless of who is accessing it.
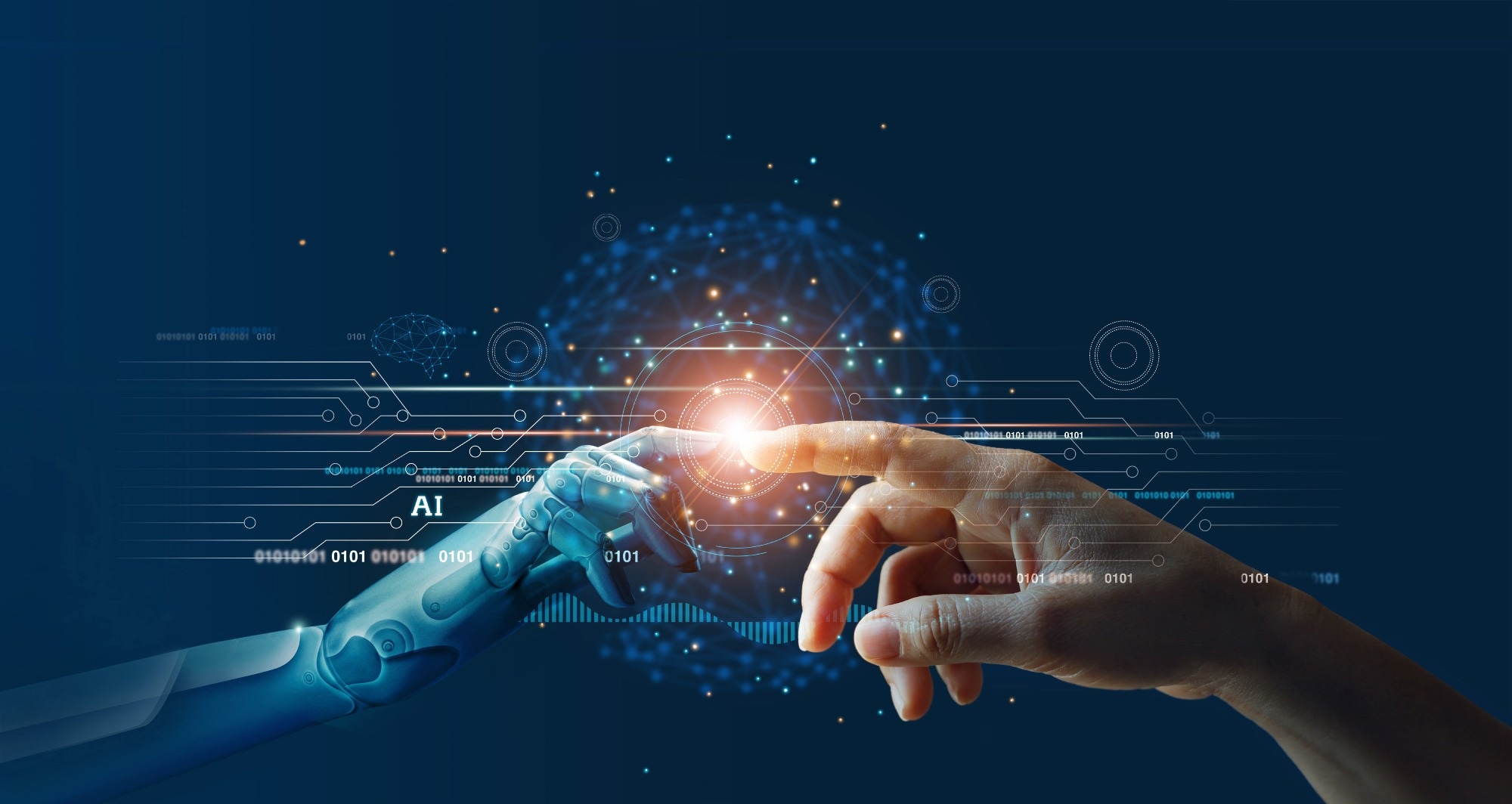
Image Credit: Shutterstock.com/PopTika
AI has been installed across various health applications, such as monitoring vital signs, recognizing emotions, detecting skin or cancer, and other medical imaging and diagnostics.
However, neural networks are just as good as the data set on which they are programmed, and minorities are poorly represented in these datasets, resulting in a principally deceptive form of technological inequality.
Hu and his team at the University of Pittsburgh Swanson School of Engineering are developing a distributed, inclusive data collection and learning framework that depends on smartphone apps, rendering it easy to join while safeguarding user privacy. The National Institutes of Health (NIH) recently awarded Hu $1,744,696 for this study.
“Existing and easily accessible data sets are inherently biased. It’s not always easy for people in marginalized communities to participate in data collection and research, and these communities might also lack medical professionals,” said Hu, Associate Professor and William Kepler Whiteford Faculty Fellow of Electrical and Computer Engineering.
AI could make critical healthcare more accessible for these communities; but without a dataset that accurately reflects the diversity of the population, AI could misdiagnose people that are under-represented during the data collection stage, thereby increasing healthcare disparities.
Jingtong Hu, Associate Professor and William Kepler Whiteford Faculty Fellow, Electrical and Computer Engineering, Swanson School of Engineering, University of Pittsburgh
Hu’s project would help curb these inequalities by creating an on-device learning framework that learns nonstop from the data of new users when using a mobile application. It will make the most of federated learning (FL), which uses numerous devices to collaboratively program a shared model while preserving the data on the devices.
In FL, the models are shared with the Cloud rather than user data, thereby safeguarding user privacy.
By using this method, not only can we improve the global model to be fairer by incorporating more equally represented data, but we can personalize the model for each individual. After all, the most important metrics for each user is the accuracy for him or herself.
Jingtong Hu, Associate Professor and William Kepler Whiteford Faculty Fellow, Electrical and Computer Engineering, Swanson School of Engineering, University of Pittsburgh
“A user could use our app to diagnose their skin condition, for example, to see if a skin issue is skin cancer or just normal eczema. Meanwhile, our algorithm will learn from the new images locally. Patients' images will not be uploaded to the server; they will be analyzed on their own cell phones,” Hu added.
In contrast to present frameworks, this would depend on unsupervised learning with data transmitted from a range of smartphone models and other devices, enabling more people to join in the study. The framework would also have to be mindful of the fairness of diverse machine learning models.
Going forward, the team will build a machine learning framework that will automatically search already present learning models and use the most favorable architectures for varied datasets.
The project, “Achieve Fairness in AI-Assisted Mobile Healthcare Apps through Unsupervised Federated Learning,” is funded for four years in partnership with Dr. Alaina James from the University of Pittsburgh School of Medicine’s Department of Dermatology, Dr. Lei Yang from George Mason University, and Dr. Yiyu Shi from the University of Notre Dame.