Reviewed by Emily Henderson, B.Sc.Nov 1 2022
Computers can be taught to generate mixtures from a set of target properties using an inverse mixture-design approach based on machine learning. KAUST-developed technology could be used to identify high-performance transportation fuels with minimal atmospheric carbon dioxide (CO2) emissions.
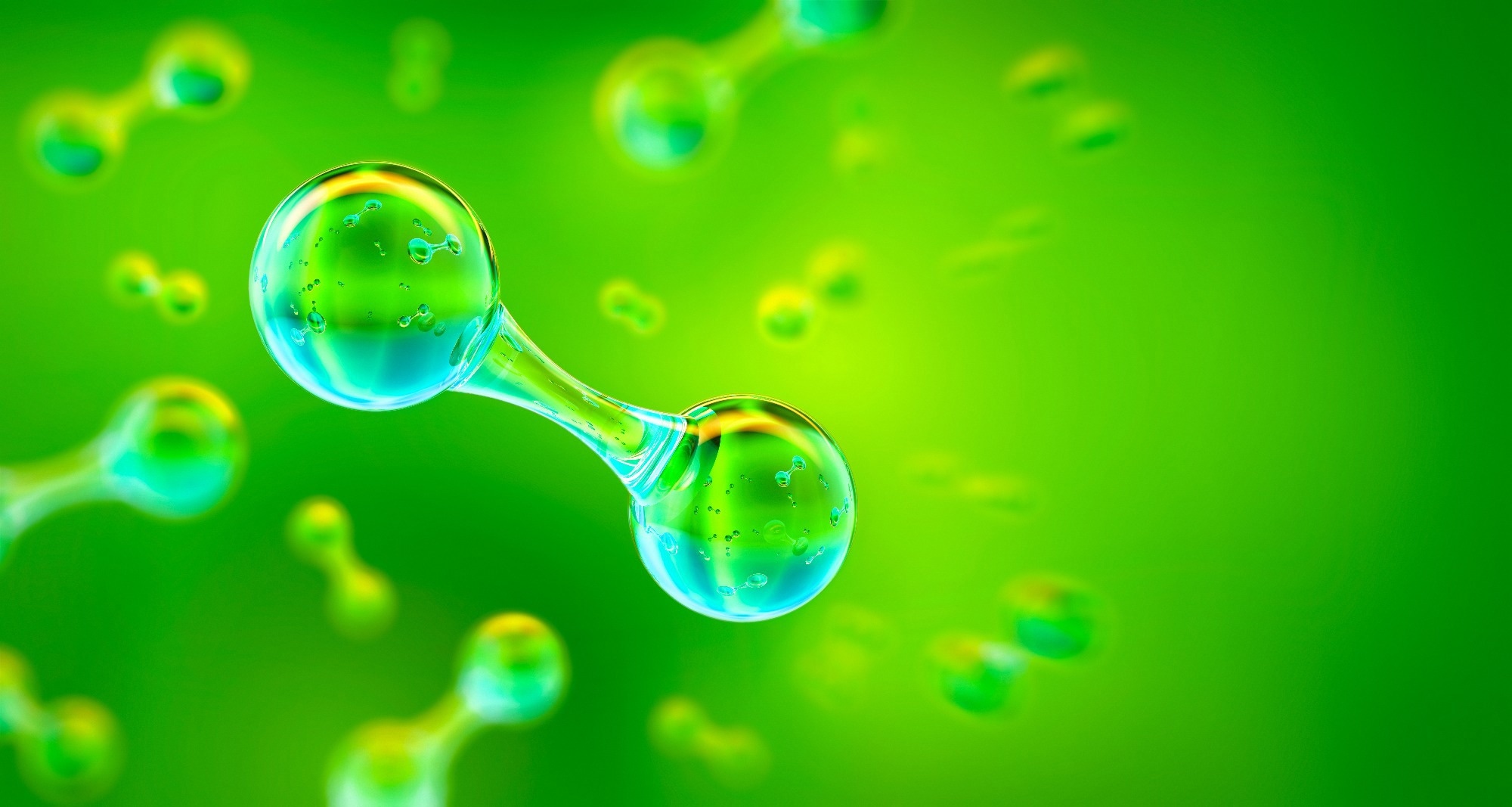
Image Credit: Corona Borealis Studio/Shutterstock
Most of the increase in global temperatures can be attributed to greenhouse gas emissions. The combustion of hydrocarbon fuels, such as gasoline, which power most automotive engines, is a major source of CO2 emissions.
Engineering transportation fuels with higher efficiency and reduced carbon emissions is a viable answer to these environmental problems.
Numerous methods for fuel screening have been developed; however, they are typically only proven on smaller blends or call for additional preprocessing, making these combinations unsuitable for inverse fuel design.
The key bottleneck is screening complex mixtures containing hundreds of components to predict synergistic and antagonistic effects of species on the resultant mixture properties.
Nursulu Kuzhagaliyeva, Study First Author and PhD Student, Clean Combustion Research Center, King Abdullah University of Science and Technology
To effectively screen fuels, Kuzhagaliyeva, Mani Sarathy, and colleagues built a deep learning model that consists of numerous smaller networks dedicated to particular tasks.
“This problem was a good fit for deep learning that allows capturing nonlinear interactions between species,” stated Kuzhagaliyeva.
The researchers used the inverse-design method to identify possible fuels by first defining combustion-related characteristics, such as fuel ignition quality and sooting propensity.
Few experimental data are available to the general public. To train the model, the researchers created a large database utilizing experimental measures from the literature. The database included all kinds of pure compounds, substitute fuel blends, and complex mixtures, like gasoline.
The researchers had to incorporate vector representations into the model as no model could be modified for inverse fuel design, according to Kuzhagaliyeva. They created a mixing operator that directly connects hidden representations of pure compounds and mixes through linear combinations.
This mixing operator was inspired by text processing methods that use hidden vectors to connect words to phrases. They also included search algorithms to find fuel mixes within a chemical space that match the predefined parameters.
The model correctly predicted the fuel ignition quality and the sooting propensity of different molecules and mixes. Additionally, it found several gasoline blends that met the predetermined standards.
The team is now improving model accuracy by adding new properties to the property database, like volatility, viscosity, and pollutant generation. The technique is being improved to create synthetic aviation fuels and e-fuels for gasoline.
Kuzhagaliyeva added,
We are also developing a cloud-based platform to enable others to use the tool.”
Journal Reference:
Kuzhagaliyeva, N., et al. (2022) Artificial intelligence-driven design of fuel mixtures. Communications Chemistry. doi:10.1038/s42004-022-00722-3.