Reviewed by Emily Henderson, B.Sc.Nov 1 2022
Most melanoma deaths happen to individuals originally diagnosed with early-stage melanoma and then encountered a recurrence that is often not recognized until it has spread or metastasized.
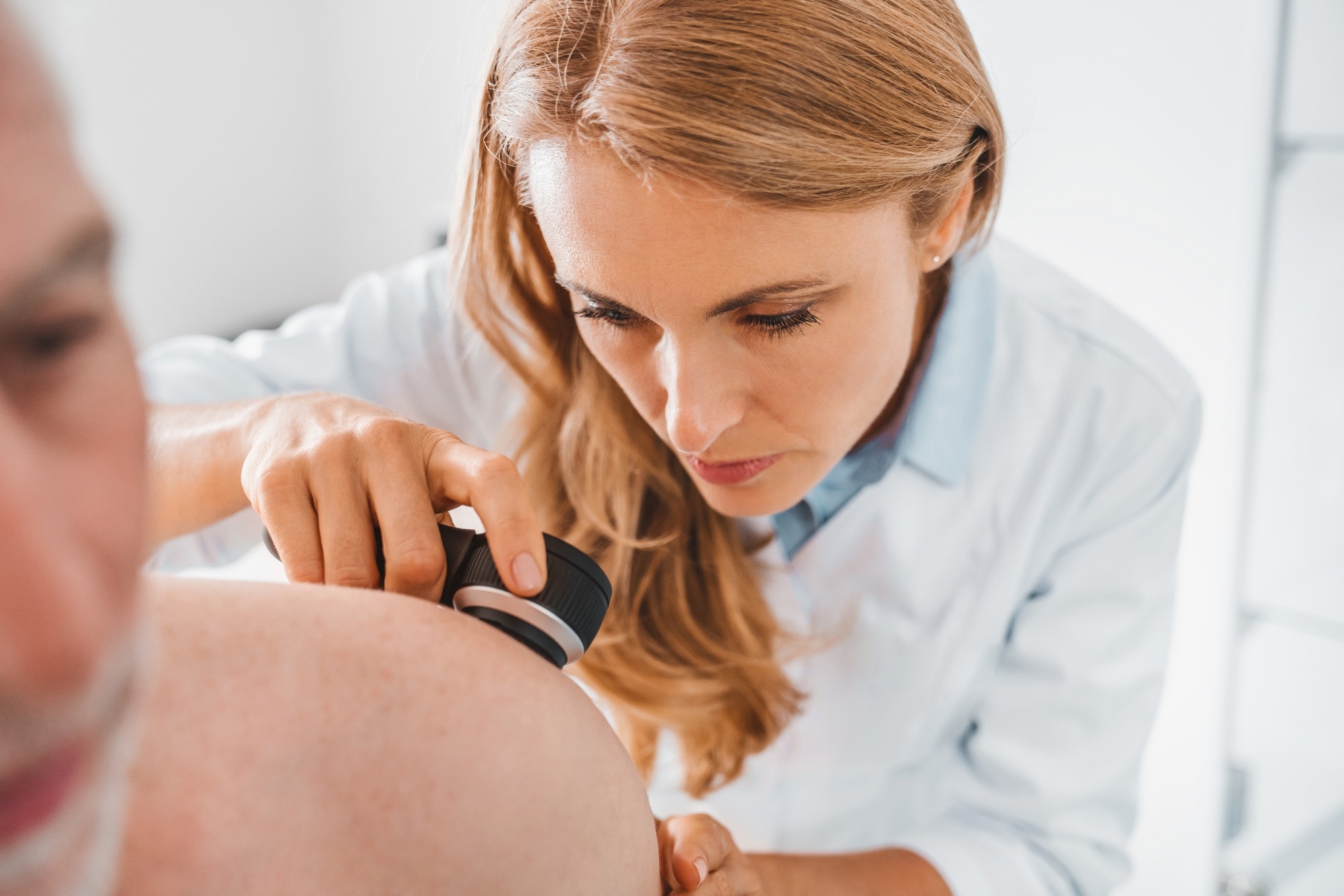
Image Credit: Inside Creative House/Shutterstock
Researchers at Massachusetts General Hospital (MGH) recently devised an artificial intelligence-based method for determining which patients are most likely to have a recurrence and benefit from aggressive treatment. The study was published in the journal npj Precision Oncology.
Most individuals with early-stage melanoma are treated with surgery to eliminate cancerous cells. Still, patients with more advanced cancer are frequently treated with immune checkpoint inhibitors, which efficaciously enhance the immune response against tumor cells but have serious side effects.
There is an urgent need to develop predictive tools to assist in the selection of high-risk patients for whom the benefits of immune checkpoint inhibitors would justify the high rate of morbid and potentially fatal immunologic adverse events observed with this therapeutic class.
Yevgeniy R. Semenov MD, Study Senior Author and Investigator, Department of Dermatology, Massachusetts General Hospital
Semenov says, “Reliable prediction of melanoma recurrence can enable more precise treatment selection for immunotherapy, reduce progression to metastatic disease, and improve melanoma survival while minimizing exposure to treatment toxicities.”
Semenov and his coworkers evaluated the efficacy of machine learning-based algorithms, a branch of artificial intelligence, to aid in this goal by predicting the recurrence of melanoma using information from patient electronic health records.
The researchers gathered 1,720 early-stage melanomas—1,172 from the Mass General Brigham healthcare system (MGB) and 548 from the Dana-Farber Cancer Institute (DFCI)—and retrieved 36 clinical and pathologic features from electronic health records to estimate patients' risk of recurrence using machine learning algorithms.
Algorithms were created and tested using different MGB and DFCI patient sets, and tumor thickness and rate of cancer cell division were found to be the most predictive features.
Our comprehensive risk prediction platform using novel machine learning approaches to determine the risk of early-stage melanoma recurrence reached high levels of classification and time to event prediction accuracy. Our results suggest that machine learning algorithms can extract predictive signals from clinicopathologic features for early-stage melanoma recurrence prediction, which will enable the identification of patients who may benefit from adjuvant immunotherapy.
Yevgeniy R. Semenov MD, Study Senior Author and Investigator, Department of Dermatology, Massachusetts General Hospital
Ahmad Rajeh, Michael R. Collier, Min Seok Choi, Munachimso Amadife, Kimberly Tang, Shijia Zhang, Jordan Phillips, Nora A. Alexander, Yining Hua, Wenxin Chen, Diane, Ho, Stacey Duey, and Genevieve M. Boland also contributed to the study.
The research was funded by the Melanoma Research Alliance, the National Institutes of Health, the Department of Defense, and the Dermatology Foundation.
Journal Reference:
Wan, G., et al. (2022) Prediction of early-stage melanoma recurrence using clinical and histopathologic features. npj Precision Oncology. doi.org/10.1038/s41698-022-00321-4.