Various studies performed displayed that AI systems do not improve radiologists’ diagnostic performance in spite of the exceptional advancements in artificial intelligence (AI).
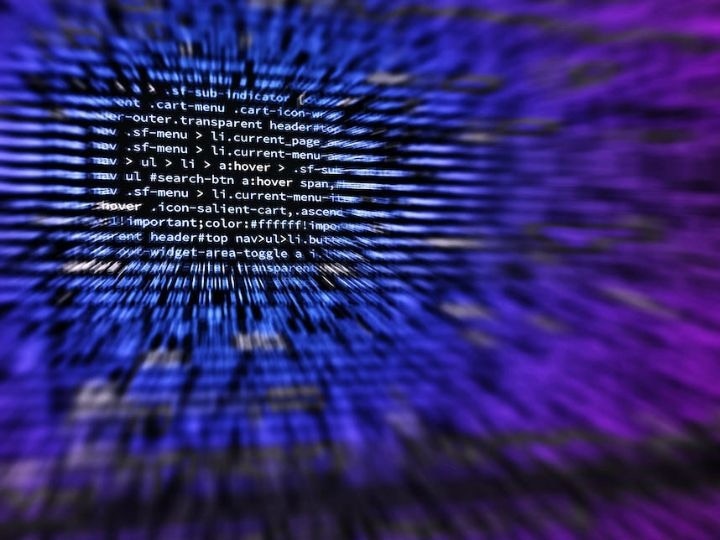
The new computational framework uses a unique combination of eye-gaze tracking, intention reverse engineering, and reinforcement learning to decide when and how an AI system should interact with radiologists. Image Credit: University of Houston.
Indeed, diagnostic errors add up to 40,000 to 80,000 deaths per year in US hospitals. This lapse identifies an urgent need to develop next-generation computer-aided diagnosis algorithms that are highly interactive.
That is just what Hien Van Nguyen, the University of Houston associate professor of electrical and computer engineering, is making with a new $933,812 grant from the National Cancer Institute. He will concentrate on lung cancer diagnostics.
Current AI systems focus on improving stand-alone performances while neglecting team interaction with radiologists. This project aims to develop a computational framework for AI to collaborate with human radiologists on medical diagnosis tasks.
Hien Van Nguyen, Associate Professor, Electrical and Computer Engineering, University of Houston
That framework makes use of a special combination of eye-gaze tracking, reinforcement learning, and intention reverse engineering to determine when and how an AI system must communicate with radiologists.
Van Nguyen is developing a user-friendly and negligibly interfering interface for radiologist-AI interaction to optimize time efficiency and reduce the amount of distraction in clinical work.
The project calculates the methods for two clinically significant applications: pulmonary embolism and lung nodule detection. Pulmonary embolism is the third most common cause of cardiovascular death, and lung cancer is known to be the second most common cancer.
Studying how AI can help radiologists reduce these diseases’ diagnostic errors will have significant clinical impacts. This project will significantly advance the knowledge of the field by addressing important, but largely under-explored questions.
Hien Van Nguyen, Associate Professor, Electrical and Computer Engineering, University of Houston
The questions consist of when and how AI systems must interact with radiologists and how to model radiologists’ visual scanning process.
Our approaches are creative and original because they represent a substantive departure from the existing algorithms. Instead of continuously providing AI predictions, our system uses a gaze-assisted reinforcement learning agent to determine the optimal time and type of information to present to radiologists.
Hien Van Nguyen, Associate Professor, Electrical and Computer Engineering, University of Houston
Nguyen added, “Our project will advance the strategies for designing user interfaces for doctor-AI interaction by combining gaze-sensing and novel AI methodologies.”
Source: https://uh.edu/