One of the most effective and well-recognized diagnostic technologies accessible in contemporary medicine is computed tomography (CT). As more individuals choose CT scans, concerns have been raised concerning the quantity of X-Ray radiation that patients are exposed to.
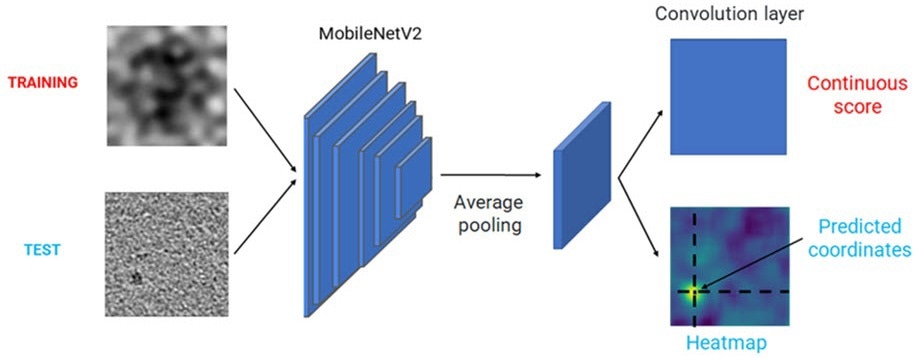
Researchers from Italy developed and trained neural-network-based models to analyze CT scan images. These models accurately matched the assessment of a human professional and can help optimize radiation dose without compromising a patient’s diagnosis. Image Credit: Valeri et al., doi 10.1117/1.JMI.10.S1.S11904
A patient should, ideally, only be exposed to a minimal dose of radiation during treatments or diagnostic procedures to get the desired results.
“As Low As Reasonably Achievable” is also known as the ALARA principle in real-world applications. This approach does, however, lead to a trade-off because the quality of CT images declines when radiation power is reduced. To prevent misdiagnosis, medical staff strive to achieve a balance between a patient’s exposure to X-Rays and acquiring high-quality CT images.
This balance can be reached by employing an optimization technique in which medical professionals, normally radiologists, look at actual tomographic images and try to spot abnormalities like tumors or abnormal tissue. After that, an expert uses statistical techniques to determine the ideal radiation dose and tomographer configuration.
By using reference CT scans of specially created phantoms with inserts of various sizes and contrasts, which reflect standardized abnormalities, this procedure can be generalized. Nevertheless, manual image analysis like these requires considerable time.
Using artificial intelligence (AI), an Italian research team led by Dr. Sandra Doria and members from the University of Florence’s Physics Department, radiologists, and medical physicists from Florence Hospital investigated the viability of automating this procedure.
The researchers developed and trained a “model observer” method based on convolutional neural networks (CNNs), which could assess the standardized abnormalities in CT images just as well as an expert, as was described in the Journal of Medical Imaging (JMI).
The team had to produce enough training and testing data for the model to do this. A total of 1000 CT images with phantoms that resembled human tissue in each were visually inspected by thirty healthcare professionals. This material, appropriately called “phantom,” contains cylinder inserts with various sizes and contrasts.
The observers were tasked with determining if and where the inserted item appeared in each of the images, in addition to indicating their level of confidence. As a consequence, a dataset of 30,000 labeled CT images was produced, precisely representing human interpretation and obtained using various tomographic reconstruction configurations.
Subsequently, the team built UNet and MobileNetV2, two AI models with different architectures. They changed these architectures’ fundamental designs to give them the ability to classify (“Is there an uncommon object in the CT image?”) and localize (“Where is the strange object?”). Using images from the dataset, they trained and evaluated the models.
The study team used statistical analysis to examine several performance indicators to confirm that the model observers could effectively simulate how a person would evaluate the phantom’s CT images.
Our results were very promising, as both trained models performed remarkably well and achieved an absolute percentage error of less than 5 percent. This indicated that the models could identify the object inserted in the phantom with similar accuracy and confidence as a human professional, for almost all reconstruction configurations and abnormalities sizes and contrasts.
Dr. Sandra Doria, European Laboratory for Non-Linear Spectroscopy LENS, University of Florence
The model developed by Doria and her colleagues, in their opinion, has the potential to be a viable technique for automatically evaluating the quality of CT images.
Doria added, “Our CNN-based model observers could greatly simplify the process of optimizing the radiation dose used in CT protocols, thereby minimizing health risks to the patient, and help avoid the time-consuming limitations of medical evaluations.”
Doria expressed confidence in the team’s ability to successfully implement its AI model observers on a wider scale, resulting in the fastest and safest CT assessments yet.
Journal Reference
Valeri, F., et al. (2023) UNet and MobileNet CNN-based model observers for CT protocol optimization: comparative performance evaluation by means of phantom CT images. Journal of Medical Imaging. doi:10.1117/1.JMI.10.S1.S11904.