Metal additive manufacturing is used by many sectors to quickly create parts and components. Additive manufacturing, which entails layer-by-layer assembly of parts using a 3D printer, is a technique used to create orthopedic implants, high-performance automotive pistons, and rocket engine nozzles.
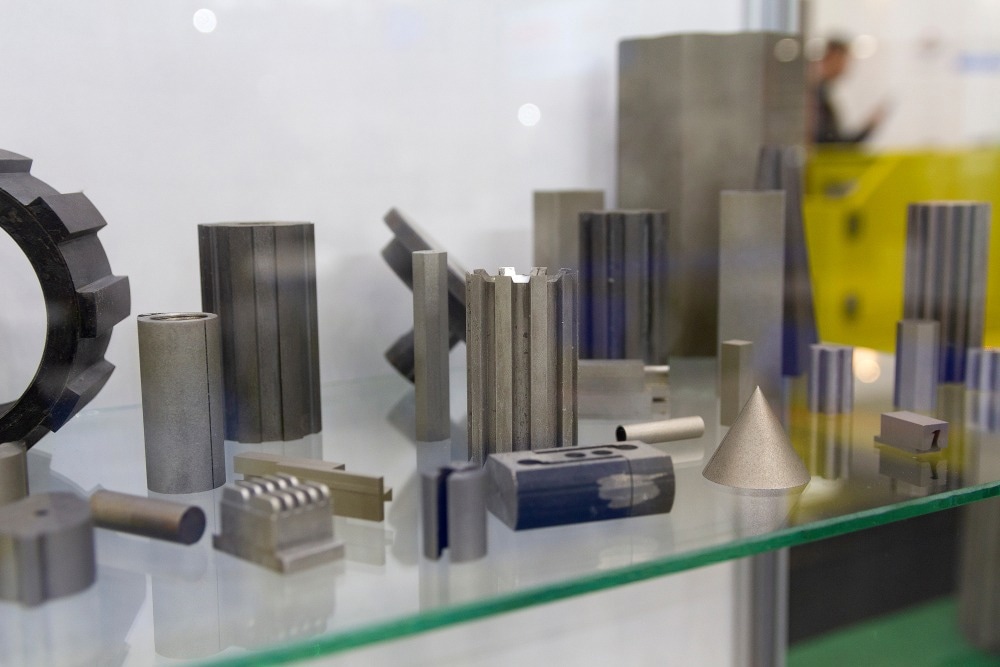
Image Credit: DmyTo/Shutterstock.com
Users are able to construct complex components with speed using additive manufacturing; however, during the building process, structural defects can form. Because of this, additive manufacturing is yet to be widely used.
Scientists from the US Department of Energy’s (DOE) Argonne National Laboratory have created a brand-new technique for identifying and anticipating flaws in 3D printed materials that has the potential to revolutionize additive manufacturing.
A study team led by Argonne and the University of Virginia (UVA) recently published the approach in the journal Science. The scientists were able to identify and anticipate the emergence of pores in 3D-printed metals in real time with almost perfect accuracy using a variety of imaging and machine-learning approaches.
By heating metal powder with a laser and then melting it into the required shape, a method known as laser powder bed fusion was employed to manufacture the metal samples used in the study. Still, this method frequently causes pores to develop, which might impair a part’s performance.
Many additive manufacturing machines feature thermal imaging sensors that keep track of the build process, however, since these sensors only image the surface of the parts being manufactured, they can overlook the development of pores.
Intense X-Ray beams, such as those produced by the Advanced Photon Source (APS), a DOE Office of Science user facility in Argonne, are the sole technique to directly identify pores inside solid, metal parts.
Our X-ray beams are so intense that we can image more than a million frames per second.
Samuel Clark, Assistant Physicist, Argonne National Laboratory
With the aid of these images, the researchers could watch pore formation in action. The researchers found that holes produced within a sample produce different thermal signatures at the surface that thermal cameras can identify.
Finally, using just thermal images, the researchers developed a machine-learning model to forecast the development of pores within 3D metals. They used data from the X-Ray images, which they knew properly depicted the formation of pores, to validate the model. The model was then put to the test in unlabeled samples to see if it could identify heat signals and predict pore generation.
The APS offered the 100% accurate ground truth that allowed us to achieve perfect prediction of pore generation with our model.
Tao Sun, Associate Professor, University of Virginia
There are sensors in many additive manufacturing machines currently on the market, but they are not quite as precise as the technique the researchers found.
Our approach can readily be implemented in commercial systems. With only a thermal camera, the machines should be able to detect when and where pores are generated during the printing process and adjust their parameters accordingly.
Kamel Fezzaa, Physicist, Argonne National Laboratory
For instance, a machine could immediately cease building a part if it discovers a significant flaw early in the manufacturing process. The new method can give users information about potential pore defects in the part, saving time during inspection even if the production process is not stopped.
Sun added, “If you have a log file that tells you these four locations could have defects, then you are just going to check out these four locations instead of looking at the entire part.”
The ultimate objective is to develop a system that not only finds defects but also fixes them while it is being manufactured. In the future, the researchers will develop sensors that can find several kinds of additive manufacturing process defects.
“In the end, we want to develop a comprehensive system that can tell you not only where you possibly have defects, but also what exactly the defect is and how it might be fixed,” Sun concluded.
Journal Reference:
Ren, Z., et al. (2023) Machine learning–aided real-time detection of keyhole pore generation in laser powder bed fusion. Science. doi:10.1126/science.add4667.