How can an iPhone predict the next word to be written in a message? A transformer, which is at the heart of many AI applications, is the technology underlying this mechanism.
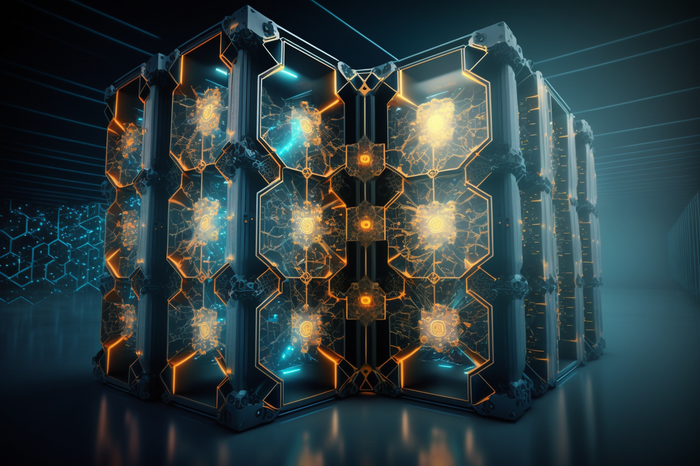
A computer server transformed by MOFs. Image Credit: Kevin Jablonka (EPFL)
EPFL and KAIST researchers have developed a new transformer for Metal-Organic Frameworks (MOFs), a kind of porous crystalline material. Chemists can create millions of distinct substances with possible uses in energy storage and gas separation by mixing organic linkers with metal nodes.
The “MOFtransformer” is intended to be the ChatGPT for MOF researchers. Its design is built on Google Brain, an AI that can understand natural language and is at the heart of popular language models such as GPT-3, ChatGPT’s precursor.
The main idea behind these models is that they have been pre-trained on a significant volume of the text so that when we start typing on an iPhone, for example, models like this “know” and autocomplete the most likely next word.
We wanted to explore this idea for MOFs, but instead of giving a word suggestion, we wanted to have it suggest a property. We pre-trained the MOFTransformer with a million hypothetical MOFs to learn their essential characteristics, which we represented as a sentence. The model was then trained to complete these sentences to give the MOF's correct characteristics.
Berend Smit, Professor, École polytechnique fédérale de Lausanne
The MOFTransformer was then adjusted for activities involving hydrogen storage, such as determining hydrogen’s storage capacity, its diffusion coefficient, and the MOF’s band gap (an “energy barrier” that determines how easily electrons can move through a material).
The method demonstrated that the MOFTransformer could provide results with significantly less data compared to conventional machine-learning techniques, which need a lot more data.
Smit added, “Because of the pre-training, the MOFTtransformer knows already many of the general properties of MOFs; and because of this knowledge, we need less data to train for another property.”
Furthermore, the same model could be utilized for all attributes, whereas in conventional machine learning, a new model for each application is required.
The MOFTransformer is a game changer in MOF studies, delivering faster answers with less data and a more comprehensive understanding of the material. The MOFTransformer, the researchers hope, will lead the way for the creation of new MOFs with outstanding properties for hydrogen storage and other uses.
Journal Reference
Kang, Y., et al. (2023) A multi-modal pre-training transformer for universal transfer learning in metal–organic frameworks. Nature Machine Intelligence. doi:10.1038/s42256-023-00628-2.