Machine learning and Artificial intelligence and (ML/AI) technologies constantly find new application across several different disciplines.
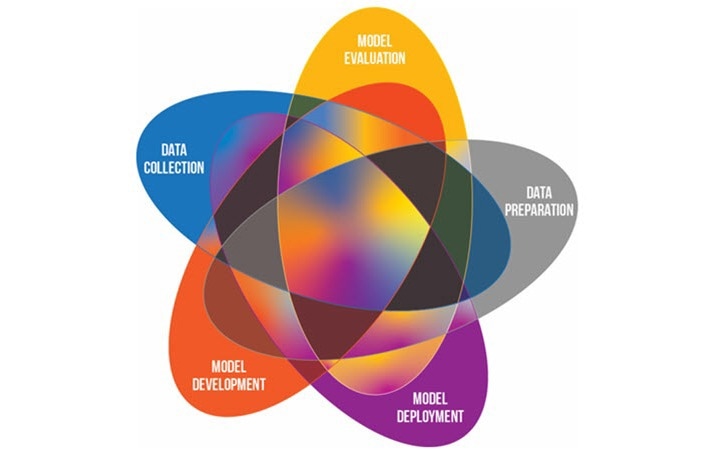
In recent years, artificial intelligence (AI) has been recognized as a powerful tool in the field of medical imaging. However, these models can be subject to several biases, leading to inequities in how they benefit both doctors and patients. Understanding these biases and how to mitigate them is the first step toward a fair and trustworthy AI. Image Credit: MIDRC, midrc.org/bias-awareness-tool.
Medicine is no exception, with AI/ML being utilized for evaluating the prognosis, diagnosis, risk assessment, and treatment response of several diseases. In particular, AI/ML models are finding increasing applications in the analysis of medical images.
This includes computed tomography, X-Ray, and magnetic resonance images.
An essential part of successfully implementing AI/ML models in medical imaging is making sure that they are designed, trained, and used as they were designed. In reality, however, it is exceptionally hard to develop AI/ML models that work well for all members of a population and can be generalized to all circumstances.
Similar to humans, AI or ML models can be biased and may result in differential treatment of medically identical cases.
It is important to address these biases and ensure fairness, equity, and trust in AI/ML for medical imaging. This necessitates the sources of biases that exist in medical imaging AI/ML and developing strategies to be identified in order to mitigate them.
Failing to do so can result in differential benefits for patients, alleviating the inequities associated with healthcare access.
As stated in the Journal of Medical Imaging (JMI), a team of experts from the Medical Imaging and Data Resource Center (MIDRC), such as medical physicists, statisticians, physicians, AI/ML researchers, and scientists from regulatory bodies, helped to address this concern.
In this extensive report, the researchers determine nearly 29 sources of potential bias that could occur together with the five critical steps to develop and implement medical imaging AI/ML from data collection, data preparation and annotation, model development, model evaluation, and model deployment, with many identified biases potentially occurring in more than one step.
Bias mitigation strategies have been discussed, and data is also made available on the MIDRC website.
One of the primary sources of bias comes under data collection. For instance, sourcing images from a single hospital or from a single type of scanner could lead to biased data collection.
Also, data collection bias could emerge as a result of differences in how particular social groups have been treated, both during research and within the healthcare system as a whole. Furthermore, data could be outdated as medical knowledge and practices develop. This initiates temporal bias in AI or ML models trained on such data.
The other sources of bias available come under data annotation and preparation and are linked closely to data collection. In this step, biases could be initiated depending on how the data has been labeled before being fed to the AI/ML model for training. Such biases might source from the personal biases of the annotators or from oversights concerning how the data itself is presented to the users that have been tasked with labeling.
Biases can also arise during model development based on how the AI/ML model itself is being reasoned and created. Inherited bias is one example that occurs when the output of a biased AI/ML model is used to train another model.
Biases caused by unequal representation of the target population or originating from historical circumstances, including societal and institutional biases that lead to discriminatory practices, are other examples.
Model evaluation could also be a possible source of bias. Testing the performance of a model, for example, could initiate biases either by using earlier biased datasets for benchmarking or using unsuitable statistical models.
Finally, bias can also creep in during the deployment of the AI/ML model in a real-life setting, mainly from the system’s users. For instance, biases can arise when a model is not used for the intended categories of configurations or images or when a user becomes over-reliant on automation.
Besides determining and completely describing such sources of potential bias, the team recommends possible methods for their mitigation and best practices for medical imaging AI/ML models to be implemented.
Hence, the article offers information to clinicians, scientists, and the general public based on the restrictions of AI/ML in medical imaging as well as a roadmap for their redressal in the next future. This, in return, could streamline a more equitable and just deployment of medical imaging AI or ML models in the future.
Journal Reference:
Drukker, K., et al. (2023) Toward fairness in artificial intelligence for medical image analysis: identification and mitigation of potential biases in the roadmap from data collection to model deployment. Journal of Medical Imaging. doi.org/10.1117/1.JMI.10.6.061104.