A significant part of economic losses happens due to plant diseases caused by fungi, viruses, and bacteria every year. Hence, the prompt detection of these diseases is essential to control their spread and reduce agricultural damage. However, it represents a significant challenge, particularly in areas of high-scale production.
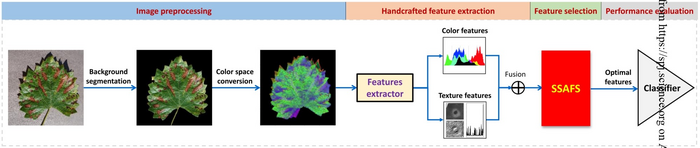
SSAFS uses an “optimal feature subset” of plant images. This subset included only the highest priority features capable of correctly classifying a plant as diseased or healthy and estimating disease severity. Image Credit: Plant Phenomics
Smart agriculture systems make use of camera surveillance fitted with artificial intelligence (AI) models to detect features of plant diseases, which frequently manifest as alterations in leaf morphology and appearance.
But traditional techniques of image classification and pattern recognition help extract features suggestive of diseased plants from a training set. Consecutively, they have low interpretability, implying it is difficult to explain what features were learned.
Furthermore, achieving huge datasets for model training is uninteresting. Handcrafted features, which are chosen based on expert-designed descriptors, feature detectors, and vocabulary, provide a viable solution to this issue. But these often lead to the adoption of unrelated features, which pave the way to reduce the performance of the algorithm.
Luckily, a solution is on the horizon. A group of plant phenomics experts and data scientists from China and Singapore have come up with a swarm intelligence algorithm for feature selection (SSAFS). This enables effective image-based plant disease detection.
The researchers reported the development and validation of this algorithm in their recent study reported in the Plant Phenomics journal. Describing the advantages of introducing SSAFS, Ji stated the following.
SSAFS not only significantly reduces the count of features, but also significantly improves the classification accuracy.
Zhiwei Ji, Study Corresponding Author and Professor, Nanjing Agricultural University The Academy of Science
A combination of two principles was utilized by the study: high-throughput phenomics via which plant traits like disease severity could be examined on a large scale, and computer vision, in which image features representative of a particular condition have been extracted.
With the help of SSAFS and a set of plant images, the scientists determined an “optimal feature subset” of plant diseases. This subset surrounded a list of only the high-priority features that could be successful in verifying a plant as diseased or healthy.
The SSAFS’ effectiveness was tested in six plant phenomics datasets and four UCI datasets. These datasets were utilized to make a comparison of the performance of SSAFS against that of five other identical swarm intelligence algorithms.
In both plant disease detection and severity estimation, the study outcomes have illustrated that SSAFS works. Certainly, it surpassed the present sophisticated algorithms in determining the highly valuable handcrafted image features.
Curiously, the majority of these disease-related features were local—that is, they involved diverse structures or patterns, like edges, points, and patches, which are frequently noted in diseased plants.
On the whole, this algorithm is a useful tool for achieving an optimal combination of handcrafted image features suggestive of plant diseases. Its adoption could considerably enhance plant disease recognition accuracy and thereby decrease the required processing duration.
When asked about the study’s future implications, Professor Ji stated:
One of the crucial contributions of this work to plant phenomics is the definition of handcrafted features and the precision screen of relevant features through a novel computational approach. We propose to combine comprehensive handcrafted and non-handcrafted features of plant images for accurate and efficient detection in the field of phenomics.
Zhiwei Ji, Study Corresponding Author and Professor, Nanjing Agricultural University The Academy of Science
Journal Reference
Xie, X., et al. (2023) A Novel Feature Selection Strategy Based on Salp Swarm Algorithm for Plant Disease Detection. Plant Phenomics. https://doi.org/10.34133/plantphenomics.0039.