The COVID-19 pandemic had a massive impact on healthcare systems around the world and helped to highlight their major shortcomings.
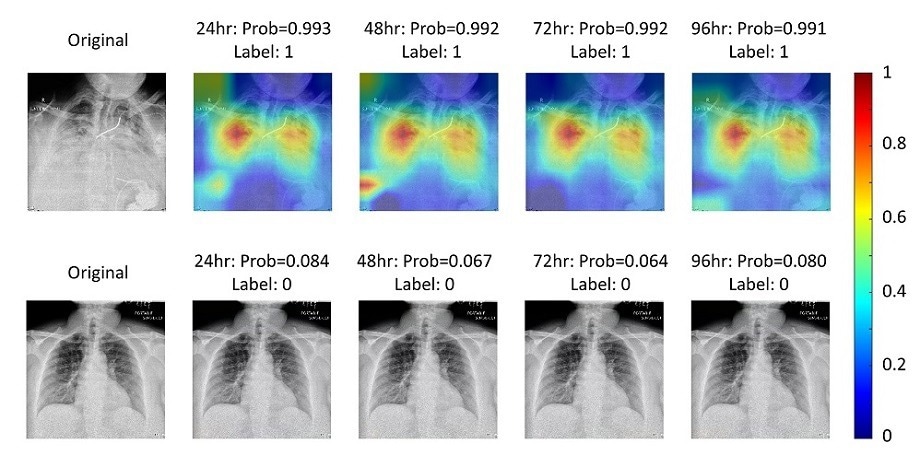
The proposed deep learning model utilizes chest X-Ray radiography images to predict if a patient with COVID-19 will need intensive care within 24, 48, 72, and 96 hours after examination. Image Credit: Li, Drukker, et al., doi 10.1117/1.JMI.10.4.044504
As of June 2023, there have been more than 760 million confirmed cases of COVID-19, with nearly 7 million deaths recorded around the world.
At the time of the major COVID-19 outbreaks, hospitals frequently had their intensive care units (ICU) running at full capacity to offer invasive mechanical ventilation to patients diagnosed with COVID-19. These ICUs often had to work with improper intubation equipment and insufficient staff.
One way to mitigate these issues is to find a way of predicting the prognosis of patients who test positive for COVID-19 in a quick and accurate manner.
Doctors tend to use chest X-Ray radiography (CXR) images to examine patient condition. By examining signs of pneumonia in these images, they can determine whether the patient will need to be admitted to the ICU.
This is a highly beneficial way to streamline the allocation of hospital resources. Despite its major advantages, this process is time-consuming, labor-intensive, and can vary in terms of its diagnosis, which is a major issue, especially during large outbreaks. This is where artificial intelligence (AI) could give a helping hand.
Recently published in the Journal of Medical Imaging, a research group from the Department of Radiology of the University of Chicago has come up with a deep learning-based model that has the potential to forecast if a patient will require intensive care by examining their CXR images.
One of the defining features of the suggested method was the use of a technique known as “transfer learning.”
Constructing deep learning models for medical imaging applications is particularly challenging due to the sheer amount of annotated data that is needed for training. Hence, rather than training a model from scratch with millions of images, one can make use of transfer learning to pass knowledge from a pre-trained model to another model.
The idea is to fine-tune the receiving model utilizing a dedicated dataset so that the “expertise” of the earlier model can be leveraged for a new task.
For instance, with transfer learning, a model trained to detect a particular disease in magnetic resonance images could act as the foundation for another model aimed at detecting a different disease.
Using this strategy, the scientists applied a sequential transfer learning process to develop their final model. They initially fine-tuned a large model, pre-trained on ImageNet with around 1.2 million natural images, utilizing CXR images from a National Institutes of Health dataset to detect around 14 different diseases.
The scientists then refined this model utilizing a dataset from the Radiological Society of North America to detect pneumonia. Ultimately, they fine-tuned it utilizing an in-house dataset comprising around 6,685 CXR images from 3,998 patients with COVID-19.
The AI model that the team has developed has the potential to forecast if a patient with COVID-19 would require intensive care within 24, 48, 72, and 96 hours of the CXR exam with a good degree of accuracy.
In an independent in-house test set (1,672 CXR images from 1,048 patients), the system was able to achieve an area under the receiver operating characteristic curve of 0.78 when predicting a patient's requirements for intensive care 24 hours in advance, and at least 0.76 for 48 or more hours, with patients identified by the model as high risk being almost five times as likely to require intensive care.
Fascinatingly, the performance of the proposed model was equivalent to similar existing models, despite relying just on CXR images rather than a combination of images and clinical data.
Overall, this model could fill a significant gap in clinical practice, as only a handful of tools have been designed to predict the prognosis of patients with COVID-19.
The team's proposed model could thus play a significant role in assisting resource management and clinical decision-making. This, in return, would enhance the quality of care that patients receive.
The scientists are already working on enhancing this model in various different ways. These include training the model with CXR images collected at multiple institutions and integrating comparable clinical variables into the model. This extends the model to include lung opacities caused as a result of related diseases and adds image segmentation and preprocessing steps.
Such measures will hopefully help to improve outcomes for patients with COVID-19 should another pandemic arise.
Journal Reference:
Li, H., et al. (2023) Predicting intensive care need for COVID-19 patients using deep learning on chest radiography. Journal of Medical Imaging. doi.org/10.1117/1.JMI.10.4.044504.
Source: https://spie.org/?SSO=1