For secured autonomous driving, precise real-time prediction of the movement of nearby vehicles or the future trajectory of pedestrians is necessary.
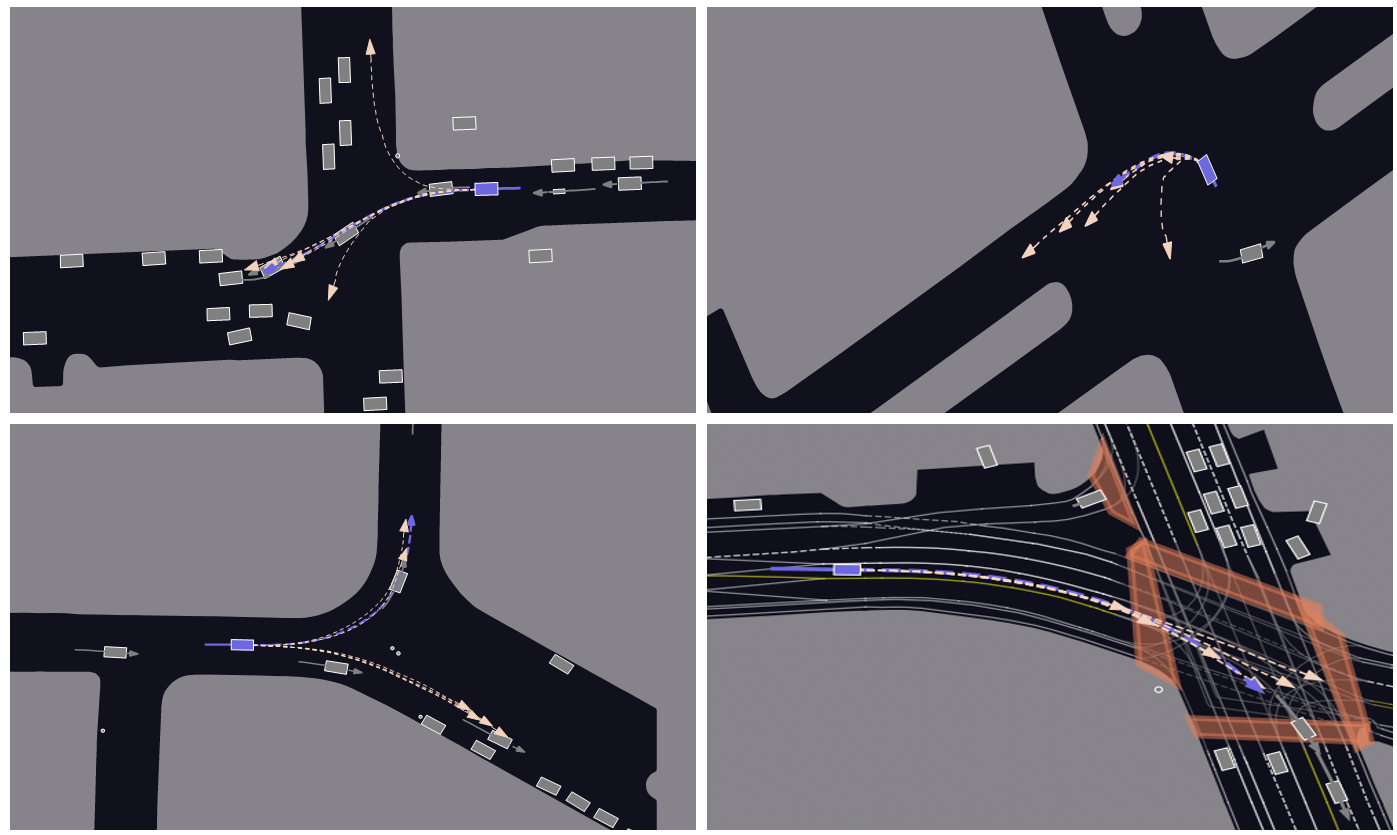
QCNet can capture the intentions of road users, accurately predicting multiple possible movements of surrounding vehicles. Image Credit: Professor Wang’s research group / City University of Hong Kong
Recently, a research group headed by the City University of Hong Kong (CityU) developed a novel AI system that enhances predictive accuracy amid dense traffic and raises computational efficiency by more than 85%, thereby providing great potential for improving the safety of autonomous vehicles.
Wang Jianping, a Professor in the Department of Computer Science (CS) at CityU, who headed the study, described the vital significance of accurate and real-time prediction in autonomous driving, emphasizing that even the least delays and errors could result in catastrophic accidents.
However, present solutions available for behavior prediction frequently fight to properly understand driving scenarios or lack efficiency in their predictions.
Generally, such solutions include re-normalizing and re-encoding the latest positional data of encircling objects and the environment whenever the vehicle and its observation window go ahead, although the latest position data considerably overlaps the preceding data. This results in redundant latency and computations in real-time online predictions.
To defeat these limitations, Professor Wang and her team presented a discovery trajectory prediction model, known as “QCNet,” which could theoretically assist the streaming processing.
It depends on the principle of comparative spacetime for positioning, which provides the prediction model with outstanding properties, like the “roto-translation invariance in the space dimension” and “translation invariance in the time dimension”.
These two properties allow the position information that has been extracted from a driving scenario to be special and fixed, irrespective of the viewer’s space-time coordinate system when the driving scenario has been viewed.
This method enables caching and reusing earlier computed encodings of the coordinates, thereby allowing the prediction model to operate in real-time theoretically.
Also, the team integrated the comparative positions of lanes, road users, and crosswalks into the AI model to capture their interactions and relationships in driving scenarios.
This improved understanding of the rules of the road and the interactions among several road users allows the model to produce collision-free predictions while considering uncertainty in the future behavior of road users.
For the efficacy of QCNet to be assessed, the research group made use of “Argoverse 1” and “Argoverse 2,” two large-scale collections of open-source autonomous driving data and high-definition maps from various US cities.
These datasets are known to be the most difficult benchmarks for behavior prediction, compassing more than 320,000 sequences of data and 250,000 scenarios.
In testing, QCNet illustrated both speed and precision in forecasting road users’ future movements. This could happen with a long-term prediction of up to six seconds. It ranked first among the 333 prediction methods on Argoverse 1 and 44 approaches on Argoverse 2.
Furthermore, QCNet considerably decreased online inference latency from 8 ms to 1 ms and increased the efficiency by more than 85% in the densest traffic scene, including 190 road users and 169 map polygons, like crosswalks and lanes.
By integrating this technology into autonomous driving systems, the autonomous vehicles can effectively understand their surroundings, predict the future behavior of other users more accurately, and make safer and more human-like decisions, paving the way for safe autonomous driving. We plan to apply this technology to more applications in autonomous driving, including traffic simulations and human-like decision-making.
Wang Jianping, Professor, Department of Computer Science, City University of Hong Kong
The study’s first author is Mr Zhou Zikang, a PhD student in Professor Wang’s research group in the Department of CS at CityU. The corresponding author of the study is Professor Wang.
Also contributing to the study were collaborators from the Hon Hai Research Institute, a research center established by Hon Hai Technology Group (Foxconn®), and Carnegie Mellon University in the US. The study outcomes will be combined into Hon Hai Technology Group’s autonomous driving system to improve self-driving safety and real-time prediction efficiency.
The study was financially supported by several funding sources, including Hon Hai Research Institute, the Hong Kong Research Grants Council, and the Shenzhen Science and Technology Innovation Commission.