A new algorithm, based on machine learning, accelerates the measurement of materials libraries by up to four times their previous speed.
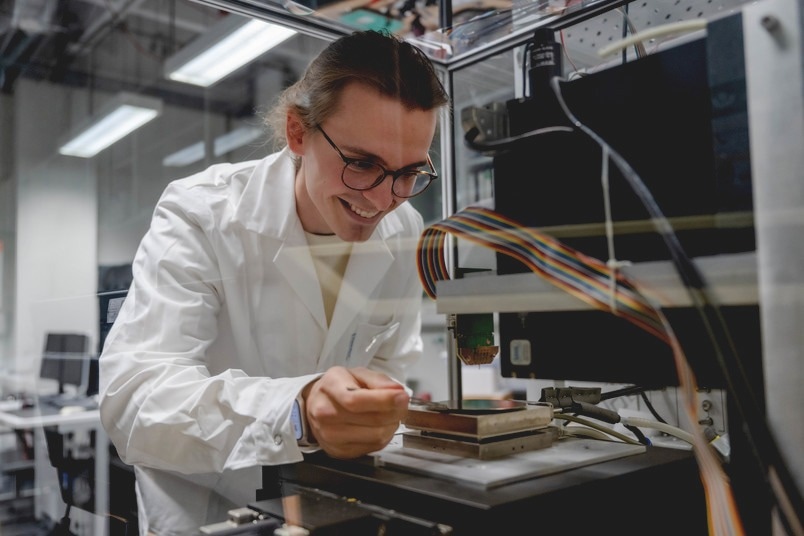
Felix Thelen is writing his doctoral thesis at the Chair of New Materials and Interfaces at Ruhr University. Image Credit: Ruhr University Bochum, Marquard
Researchers are dedicated to discovering materials crucial for future technologies, particularly for the energy transition, such as electrocatalysts. Materials composed of five or more elements are highly valuable due to their versatile properties. With around 50 elements available on the periodic table, the number of potential materials is nearly limitless.
Felix Thelen, from Ruhr University Bochum's Chair of Materials Discovery and Interfaces, led by Professor Alfred Ludwig, has introduced an algorithm that can rapidly evaluate material candidates, achieving a fourfold increase in speed. This advancement is made possible through active learning, a subset of machine learning. The research team reported its findings in the journal Digital Discovery on September 19, 2023.
Days Or Weeks to Measure a Sample
Specific methods are used to produce a range of materials on a single sample and then measure them inevitably. Each minute counts while inspecting them as the days or even weeks can pass before the characterization of a sample is complete. The novel algorithm can be combined with prevailing measuring instruments to increase their efficiency effectively.
The Measuring Instrument Itself Searches for the Next Measurement Area
Through active learning, a measuring instrument is able to independently select the next measurement area on a sample, based on the information already available about the material.
Felix Thelen, Developer, Ruhr University Bochum
The measurement process can be halted at a specific point, and the model generated will subsequently predict the results for the remaining measurement areas.
The research team from Bochum demonstrated the functionality of the algorithm by analyzing ten materials libraries through electrical resistance measurements.
Our work is only just beginning at this point. This is because in materials research there are far more complex measurement methods than resistance measurement, which also need to be optimized.
Felix Thelen, Developer, Ruhr University Bochum
Collaboration with instrument manufacturers is essential to develop solutions that can integrate these active learning algorithms.
Autonome Widerstandsmessung mit Active Learning
Video Credit: Ruhr University Bochum
Journal Reference
Thelen, F., et al. (2023). Speeding up high-throughput characterization of materials libraries by active learning: autonomous electrical resistance measurements. Digital Discovery. doi.org/10.1039/D3DD00125C.