A research team has effectively created technology that, through the use of the mutual information loss function during image conversion, can decrease structural distortion in images.
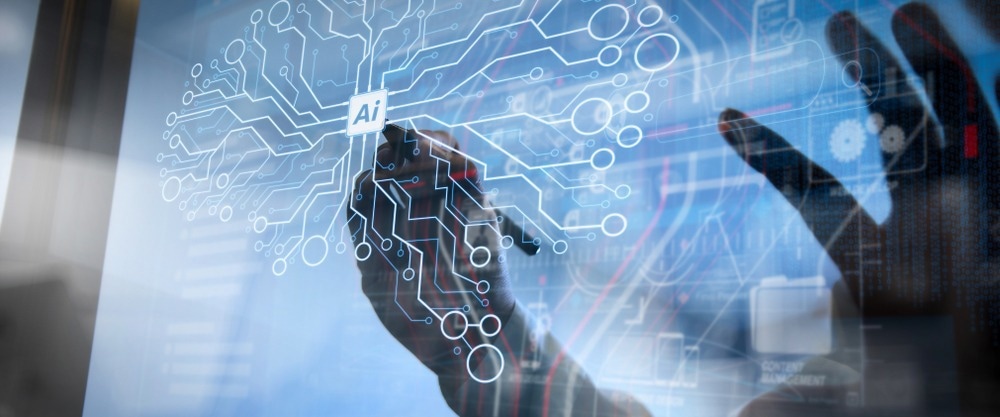
Image Credit: everything possible/Shutterstock.com
The Department of Robotics and Mechatronics Engineering at Daegu Gyeongbuk Institute of Science and Technology (DGIST; President Young Kuk) has a faculty member named Sang-hyun Park who specializes in medical image domain-adaptive artificial intelligence (AI) model technology.
This technique makes it possible to analyze medical images accurately without sacrificing performance, even whether they come from various scanners or environments. Reducing the amount of time and money required to create labels for each change in an image’s attributes and generate fresh training data, is anticipated to have a significant positive impact on the medical industry.
When an image other than the format used for training is fed into the model, medical AI performance frequently plummets. For example, if MRI data from Company A’s scanner is fed into a model that was developed using MRI data from Company B's scanner, performance can suffer.
Furthermore, a dataset created by converting CT images to MRI images can be developed to perform a more accurate analysis in situations when multimodality images, such as those from computed tomography (CT) and magnetic resonance imaging (MRI), are difficult to acquire.
While some recent studies have proposed ways to modify image styles, most of them concentrate on transforming the styles of common images, which frequently results in structural distortion during image conversion. However, organs, blood vessels, and lesions should not show any structural distortion in medical imaging utilized for proper medical diagnosis.
Hence, equipment that reduces structural deformation and prevents performance degradation is needed to provide reliable interpretation of medical images. In this regard, the research team led by Professor Park has effectively created technology that, through the use of the mutual information loss function during image conversion, can decrease structural distortion in images.
Based on the discriminator loss function, this method creates realistic images by extracting texture information from images in a new domain and structural information from medical images.
It also makes use of the mutual information loss function and texture co-occurrence loss function to produce images with less structural distortions while preserving the texture information of the new domain. If a deep learning model is built using these generated images, this technology can be used to generate images in a new domain and enable domain adaptation.
Using this method, Professor Park’s study team generated opposite images with distinct modalities for fundus images, prostate MRIs, heart CT scans, and MRI regions that were acquired from different centers. This enabled domain adaptability.
The approach outperforms conventional domain adaptation and picture conversion techniques, as demonstrated by the findings, which generated images with diverse modalities while keeping structure.
In this study, we have successfully developed technology that can significantly save the time and cost required to train a new artificial intelligence model whenever the domain changes in the medical field. This technology is expected to greatly contribute to the development of diagnostic software applicable across various healthcare sites.
Sang-hyun Park, Professor, Department of Robotics and Mechatronics Engineering, Daegu Gyeongbuk Institute of Science and Technology
The National Research Foundation of Korea’s New Researcher Support Project and the Ministry of Trade, Industry, and Energy’s Field Demand-Based Medical Device Advanced Technology Development Project provided funding for this study. The study’s results were published in Pattern Recognition, a prestigious journal in image analysis, and won accolades for excellence.
Journal Reference:
Kang, M., et al. (2023) Structure-preserving image translation for multi-source medical image domain adaptation. Pattern Recognition. doi:10.1016/j.patcog.2023.109840