Generative AI techniques, machine learning, and simulations provide researchers with fresh avenues to discover environmentally friendly metal-organic framework materials.
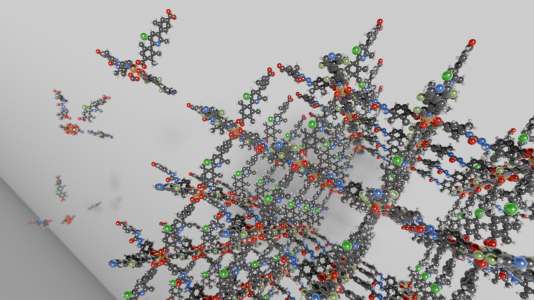
Scientific visualization of the AI-guided assembly of a novel metal organic framework with high carbon dioxide adsorption capacity and synthesizable linkers. Building blocks, predicted by generative AI, are shown on the left, while the final AI-predicted structure is shown on the right. Image Credit: Xiaoli Yan/University of Illinois Chicago and the ALCF Visualization & Data Analytics Team
Reducing greenhouse gas emissions from power stations and other industrial facilities is made possible in large part by carbon capture technology. However, a low-cost, effective material for carbon capture has not yet been discovered.
Metal-organic frameworks, or MOFs, are one possible option. Carbon dioxide can be selectively absorbed by this porous substance.
MOFs consist of three different types of molecular building blocks: organic linkers, inorganic nodes, and organic nodes. They can be positioned and configured in various relative ways. Consequently, scientists have an infinite number of possible MOF configurations to create and experiment with.
Researchers at Argonne National Laboratory, part of the US Department of Energy (DOE), are taking multiple routes to expedite the discovery process. One uses generative artificial intelligence (AI) to conjure up possibilities for building blocks that were not yet understood.
Machine learning is another kind of AI. High-throughput screening of potential materials is a third approach. Finally, there are theory-based simulations that employ the molecular dynamics technique.
Researchers from the University of Illinois at Chicago, the University of Chicago, and the Beckman Institute for Advanced Science and Technology at the University of Illinois Urbana-Champaign (UIUC) are collaborating with Argonne on this project.
It is quite difficult to design MOFs with the best carbon selectivity and capacity. Up until recently, the design of MOFs has been based on laborious computational and experimental work. This may require a lot of money and time.
Using generative AI to explore the MOF design space, the team assembled over 120,000 new MOF candidates piece by piece in less than 30 minutes. These computations were performed on the Argonne Leadership Computing Facility's (ALCF) Polaris supercomputer. An Office of Science user facility run by the DOE is the ALCF.
Using only the most promising choices, they then resorted to time-consuming molecular dynamics simulations on the UIUC Delta supercomputer. Testing them for stability, chemical makeup, and carbon-capture capabilities is the aim. Illinois and its National Center for Supercomputing Applications collaborated to create Delta.
In the end, the team's method might enable scientists to combine only the top MOF candidates.
People have been thinking about MOFs for at least two decades, and the traditional methods have typically involved experimental synthesis and computational modeling with molecular dynamics simulations. But trying to survey the vast MOF landscape in this way is just impractical.
Eliu Huerta, Computational Scientist and Study Lead Author, Argonne National Laboratory
The crew will soon have access to even more sophisticated computing. Researchers may examine billions of MOF candidates at simultaneously, including many that have never even been proposed before, because to the capabilities of the ALCF's Aurora exascale supercomputer.
Furthermore, the group is finding new ways that the many components of a MOF could interact together by drawing chemical inspiration from earlier work on molecular design.
Huerta said, “We wanted to add new flavors to the MOFs that we were designing, and we needed new ingredients for the AI recipe.”
By learning chemistry from experimental datasets in biophysics, physiology, and physical chemistry that have not previously been taken into consideration for MOF design, the team's algorithm can enhance MOFs for carbon capture.
Huerta believes that by exploring outside conventional methods, a transformational MOF material may be possible; this material might be inexpensive, easily produced, and effective at capturing carbon dioxide.
We are now connecting generative AI, high-throughput screening, molecular dynamics and Monte Carlo simulations into a standalone workflow, and this workflow incorporates online learning using past experimental and computational research to accelerate and improve the precision of AI to create new MOFs.
Eliu Huerta, Computational Scientist and Study Lead Author, Argonne National Laboratory
Ian Foster, director of the Data Science and Learning division at Argonne, described the atom-by-atom approach to MOF design made possible by AI as giving scientists a "wider lens" on these kinds of porous structures.
Work is being done so that, for the new AI-assembled MOFs that are being predicted, we incorporate insights from autonomous labs to experimentally validate their ability to be synthesized and capacity to capture carbon, and with the model fine-tuned, our predictions are just going to get better and better.
Ian Foster, Director, Department of Data Science and Learning, Argonne National Laboratory
The study was published in journal Nature Communications Chemistry, which was authored by Hyun Park, Xiaoli Yan, Ruijie Zhu, Eliu Huerta, Santanu Chaudhuri, Donny Copper, Ian Foster, and Emad Tajkhorshid.
UIUC’s Tajkhorshid said, “The study demonstrates the great potential of using AI-based approaches in molecular sciences, and we hope to extend the scope of the approach to problems such as biomolecular simulations and drug design.”
Huerta said, “This work is a testament to the collaboration between graduate students and early-career scientists from different institutions who came together to work on this important AI for science project, the future will stay bright as we continue to inspire and be inspired by talented young scientists.”
The research was funded by DOE’s Office of Science, Office of Advanced Scientific Computing Research, laboratory-directed research and development funds, and the National Science Foundation.
Journal Reference
Park, H., et.al. (2024) A generative artificial intelligence framework based on a molecular diffusion model for the design of metal-organic frameworks for carbon capture. Nature Communications Chemistry. doi.org/10.1038/s42004-023-01090-2.