May 2 2024Reviewed by Lexie Corner
In a recent paper published in the Proceedings of the AAAI Conference on Artificial Intelligence, an interdisciplinary team from Washington State University developed a new computer model that utilizes advanced artificial intelligence. 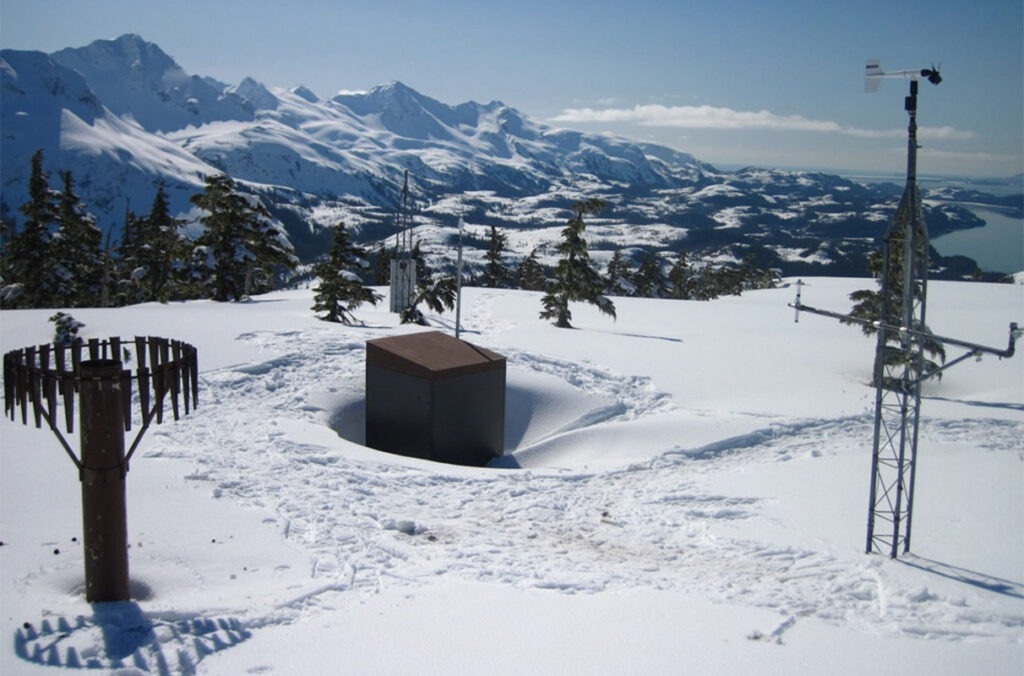
Mt. Eyak SNOTEL site is above the coastal town of Cordova, Alaska. Snow depth is about 10.5 feet, and the density is 45 %. Image Credit: Daniel Fisher of the USDA Natural Resources Conservation Service.
This model accurately predicts snow and water availability across the Western US, surpassing previous models by incorporating both time and space dimensions, which are critical for water resource management decisions.
The information provided by the new model may one day be used to improve the prediction of water availability for farmers and other users.
Researchers demonstrated that their model performed better than other models that use the AI technique known as machine learning by comparing their findings to data from more than 300 snow measuring stations in the Western United States. Prior models collected data at various times from a small number of locations and concentrated on time-related measures.
Krishu Thapa, a graduate student in Computer Science at Washington State University who led the study, emphasized the critical significance of the information for water planners across the Western region, as every water resource is allocated for irrigation, hydropower, drinking water, and environmental preservation.
Every spring, water management organizations across the West decide how best to use water based on the amount of snow that has fallen in the mountains.
This is a problem that’s deeply related to our own way of life continuing in this region in the Western U.S. Snow is definitely key in an area where more than half of the streamflow comes from snow melt. Understanding the dynamics of how that’s formed and, how that changes, and how it varies spatially is really important for all decisions.
Kirti Rajagopalan, Co-Author and Professor, Department of Biological Systems Engineering, Washington State University
Throughout the Western United States, 822 snow measurement stations offer daily data on the potential water availability at each location, a measurement known as the snow-water equivalent (SWE). The stations also offer data on temperature, relative humidity, precipitation, and snow depth.
However, the stations are dispersed, with only one station per 1,500 square miles. Depending on variables like the topography of the area, the SWE can vary significantly, even if it is only a short distance from a station.
Decision makers look at a few stations that are nearby and make a decision based on that, but how the snow melts and how the different topography or the other features are playing a role in between that’s not accounted for, and that can lead to over predicting or under predicting water supplies. Using these machine learning models, we are trying to predict it in a better way.
Bhupinderjeet Singh, Study Co-Author and Graduate Student, Biological Systems Engineering, Washington State University
To predict the daily SWE for any location - regardless of the presence of a station - the researchers created a modeling framework for SWE prediction and modified it to gather data in space and time. Previous machine learning models were limited to a single temporal variable, utilizing data from a single location over a period of days to predict outcomes for additional days.
Thapa said, “Using our new technique, we’re using both and spatial and temporal models to make decisions, and we are using the additional information to make the actual prediction for the SWE value. With our work, we’re trying to transform that physically sparse network of stations to dense points from which we can predict the value of SWE from those points that don’t have any stations.”
Rajagopalan noted that although this work is not currently utilized for direct decision-making, it represents a stride towards enhancing future forecasting and refining the inputs for stream flow prediction models.
The researchers aim to expand the model to achieve spatial completeness and ultimately transform it into a practical forecasting tool. This project was carried out under the auspices of the AI Institute for Transforming Workforce and Decision Support (AgAID Institute) and received support from the USDA’s National Institute of Food and Agriculture.
Journal Reference:
Thapa, K. K., et al. (2024) Attention-Based Models for Snow-Water Equivalent Prediction. Proceedings of the AAAI Conference on Artificial Intelligence. doi.org/10.1609/aaai.v38i21.30337
Source: https://wsu.edu/