The Olympic Games in Paris are currently underway, with thousands of athletes competing for medals. In some cases, questions may arise regarding the fairness of certain medal wins, including concerns about potential doping.
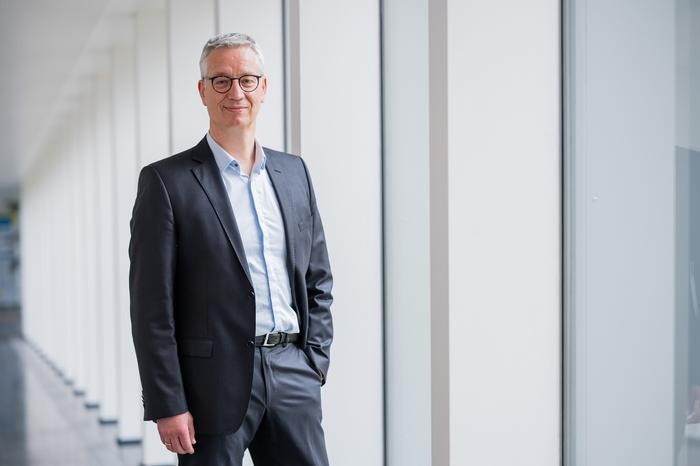
Wolfgang Maaß, Professor of Business Informatics at Saarland University. Image Credit: Oliver Dietze
Software developed by a team led by Wolfgang Maaß, a professor of business informatics at Saarland University, could play a crucial role in addressing these concerns in future competitions.
Presented at the International Joint Conference on AI (3–9 August 2024 in South Korea), this software requires only a small number of data points to predict with unprecedented accuracy which athletes have definitively not used performance-enhancing drugs, thereby identifying cases that warrant further scrutiny.
Detecting doped athletes is often compared to searching for a needle in a haystack or tilting at windmills. With thousands of athletes competing in major sporting events such as the Olympic Games, World Championships, or professional leagues like football, laboratories can take weeks to analyze urine samples to determine whether any competitors have used performance-enhancing drugs.
At the moment, the samples are all analyzed manually.
Wolfgang Maaß, Professor, Information Systems for the Service Industry, Saarland University
Given the sheer number of athletes at major events like the Olympic Games—around 10,500 in Paris—and the time-consuming nature of current testing methods, it may be possible for cheaters to be slipping through the cracks. With only a fraction of urine samples being analyzed in laboratories, it is possible that some athletes are evading detection. As highlighted by the doping scandal at the 2014 Winter Olympics in Sochi, some athletes who cheat attempt to swap their urine samples with 'clean' ones from someone else.
Until now, DNA analysis has been the only reliable method to determine whether samples have been swapped. However, Wolfgang Maaß points out that DNA analysis is both expensive and time-consuming, making it impractical to test every single sample. In search of a simpler and more feasible solution, Maaß, along with colleagues from the DFKI (German Research Center for Artificial Intelligence), the German Sport University Cologne, and the World Anti-Doping Agency (WADA), decided to combine their expertise.
“But that is both expensive and time-consuming,” explained Wolfgang Maaß.
It is just not feasible to analyze each and every sample's DNA. Maaß chose to combine his knowledge with that of his colleagues at the World Anti-Doping Agency (WADA), German Sport University Cologne, and the German Research Center for Artificial Intelligence (DFKI) to find a more straightforward and practical answer.
“This problem is practically crying out for machine analysis,” said Maaß.
To solve the problem, they created software that analyzes urine sample data quickly and affordably using artificial intelligence.
Doping tests measure the concentrations and ratios of various steroids, which are then checked for plausibility.
Wolfgang Maaß, Professor, Information Systems for the Service Industry, Saarland University
This process creates a unique biochemical profile that the Saarbrücken AI software uses to detect any anomalies with high accuracy.
The machine-learning program requires just three urine samples from each athlete, collected at different times throughout their career. Since each athlete's natural steroid profile can vary significantly, the program learns the typical concentration levels of specific substances unique to that individual. For every sample, seven key characteristics, including steroid concentrations and their ratios, are analyzed in the biochemical lab. The software then identifies any deviations from the established pattern, much like a child solving a spot-the-difference puzzle.
“If you compare the three or more ‘pictures’ with the measurement data from the individual urine samples, the software will find the ones where everything matches,” said Wolfgang Maaß, explaining in simple terms how the computer program works.
This results in a residual number of samples where the 'pictures' don't align, indicating that inconsistencies have been detected.
The small number of remaining cases can then be examined in more detail by biochemists in the laboratory using DNA analysis. If an athlete has taken a performance-enhancing substance and that substance can be detected in urine, then our software can help to identify that athlete with a high degree of certainty.
Wolfgang Maaß, Professor, Information Systems for the Service Industry, Saarland University
Instead of directly targeting doping offenders, the software is designed to identify clean athletes with 99 percent confidence, minimizing the risk of wrongly accusing innocent individuals. Although this approach may allow a small number of doping offenders to slip through undetected, it ensures that cases where athletes have used banned substances to enhance their performance—whether to go higher, farther, or faster—are identified with a high degree of certainty.
“Anyone who has doped can almost certainly be found among these remaining cases, which can then be investigated in more detail using DNA testing,” explained Wolfgang Maaß.
Thanks to this innovative and highly accurate method, finding that elusive needle in the haystack has become significantly easier.
Journal Reference:
Rahman, R. M., et al. (2024) SACNN: Self Attention-based Convolutional Neural Network for Fraudulent Behaviour Detection in Sports. doi.org/10.24963/ijcai.2024/665.