AZoRobotics speaks with Luis R. Soenksen, entrepreneur and medical device expert, currently acting as MIT's first Venture Builder in Artificial Intelligence and Healthcare. Luis was part of a team that has developed a system that uses neural networks to spot 'ugly duckling' pre-cancerous lesions on a patient's skin, helping to detect melanoma more efficiently.
Can you give our readers a summary of your recent research? What were the main aims of the research?
Early detection of suspicious pigmented lesions (SPLs) can save lives; however, the current capacity of medical systems to provide comprehensive skin screenings at scale is still lacking.
Searching for the simplest and most clinically relevant way to solve this issue, our group decided to formalize and automate the quantification of the sign commonly known as the "ugly duckling" criteria. Our research suggests that systems leveraging computer vision and deep neural networks quantifying such signs can be trained to achieve comparable accuracy to expert dermatologists.
We believe our research can open a new chapter in delivering more efficient dermatological screenings in primary care settings to drive adequate referrals.
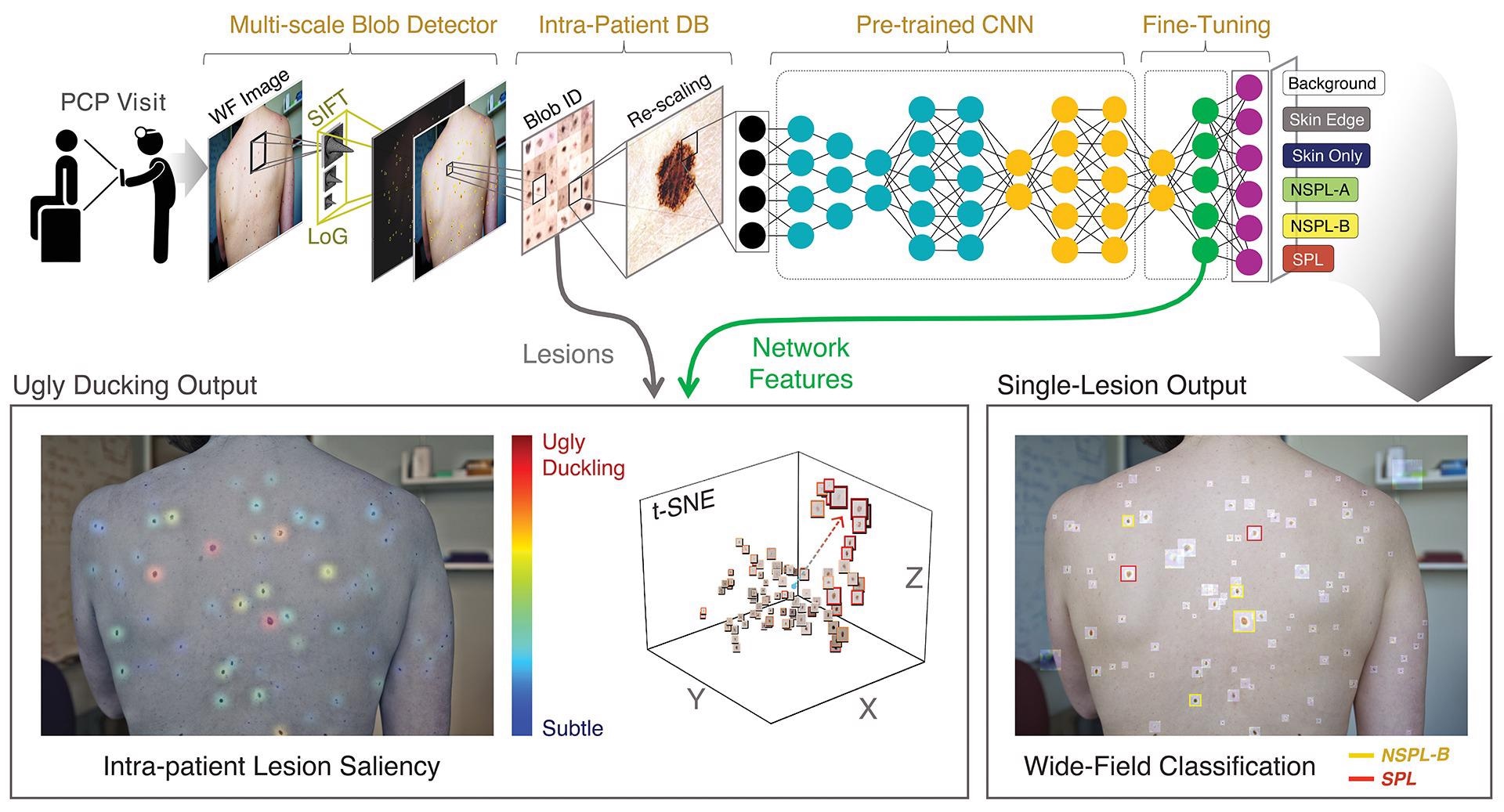
You are described as MIT's first Venture Builder in Artificial Intelligence and Healthcare. Can you give us more insight into your role?
I'm a medical device expert currently acting as MIT's first Venture Builder in Artificial Intelligence and Healthcare within MIT's Jameel Clinic and the MIT Innovation Initiative.
This exciting role at MIT has tasked me with finding, developing and furthering promising technologies on artificial intelligence and healthcare across all MIT to launch ventures that can hopefully be commercially successful and positively impact the world.
Can you give our readers more information on the deep convolutional neural networks (DCNNs) used as part of this research?
Deep Convolutional Neural Networks (DCNNs) are mathematical models that resemble networks (at least conceptually) for information processing capabilities of the human brain, which are currently trained and used to predict or classify (among many things) images.
Artificial Neural Networks are referred to as "deep" when they are stacked in many layers to learn complex patterns and abstractions in the provided data. DCNN can be made to classify images to name what they detect or to extract features out of those images.
In our research, we use the classification and feature extraction capabilities of these types of models, trained on our datasets, to cluster pigmented lesion images according to suspiciousness.
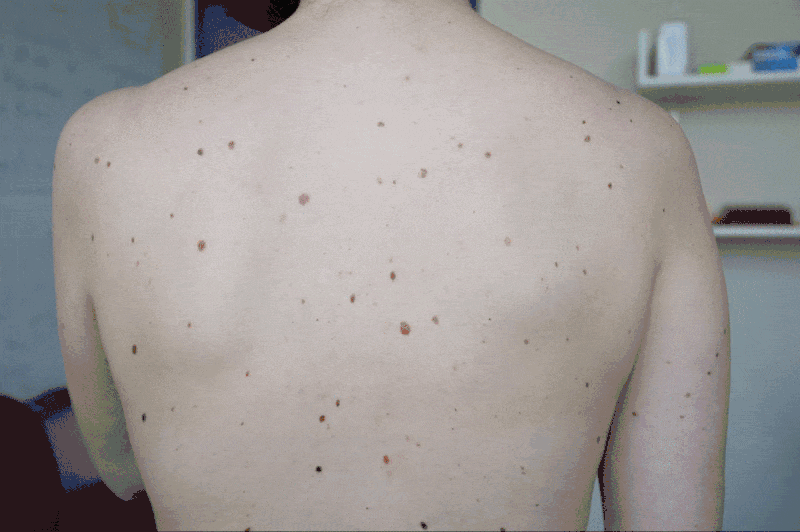
In what ways does the system identify suspicious pigmented lesions (SPLs) from non-cancerous lesions?
Suspiciousness is not a metric of malignancy. Rather, it is a metric of how likely it is that such lesion should be inspected by a dermatologist to handle it in the best possible way. A suspicious lesion may be cancerous but may also be a lesion that may become cancerous in 6 months and would benefit from long-term monitoring. In this regard, a system to identify SPLs is incredibly useful for cancer screening but also for effective referral of high-risk patients even before cancer appears.
What are some of the benefits of the early identification of suspicious pigmented lesions (SPLs)?
SPL detection is incredibly useful for cancer screening, which can save lives, but also for effective referral of high-risk patients even before cancer appears to effectively utilize finite healthcare capacity and resources in constrained healthcare systems.
What makes finding SPLs so difficult?
Until the creation of our system, finding SPLs was considered an "art" that required expert intuition and a clinical eye. Our research suggests that this identification can be standardized and can also be interpreted as a sort of "saliency" metric, which can be mathematically defined as outliers in the complex feature space of all lesions from the same patient.
What's next for the team?
We are hoping to find commercial partners to advance the utilization of this system and conduct a controlled, randomized prospective trial to demonstrate the value of utilizing these tools for melanoma screening.
Where can readers find more information?
The journal detailing the research can be found here.
About Luis R. Soenksen, Ph.D.
Luis R. Soenksen is a serial entrepreneur and medical device expert currently acting as MIT's first Venture Builder in Artificial Intelligence and Healthcare. He is leading the development and launch of multiple cutting-edge AI ventures with faculty and students across MIT. Luis holds a Ph.D. in Mechanical Engineering from MIT and a bachelor's degree in biomedical engineering from the Monterrey Institute of Technology, and a Master's of Science in Bioengineering from Johns Hopkins University.
His training includes medical device design, development and manufacturing, as well as clinical testing and the deployment of healthcare ventures. In research, Luis has made significant contributions to the fields of bioengineering, bio-design education, tissue engineering, synthetic biology and artificial intelligence.
Luis's work has been published in several high impact journals such as Science and Nature, research that has also led to several patented biomedical technologies and the co-founding of 4 start-up companies internationally.
Disclaimer: The views expressed here are those of the interviewee and do not necessarily represent the views of AZoM.com Limited (T/A) AZoNetwork, the owner and operator of this website. This disclaimer forms part of the Terms and Conditions of use of this website.