Sep 23 2012
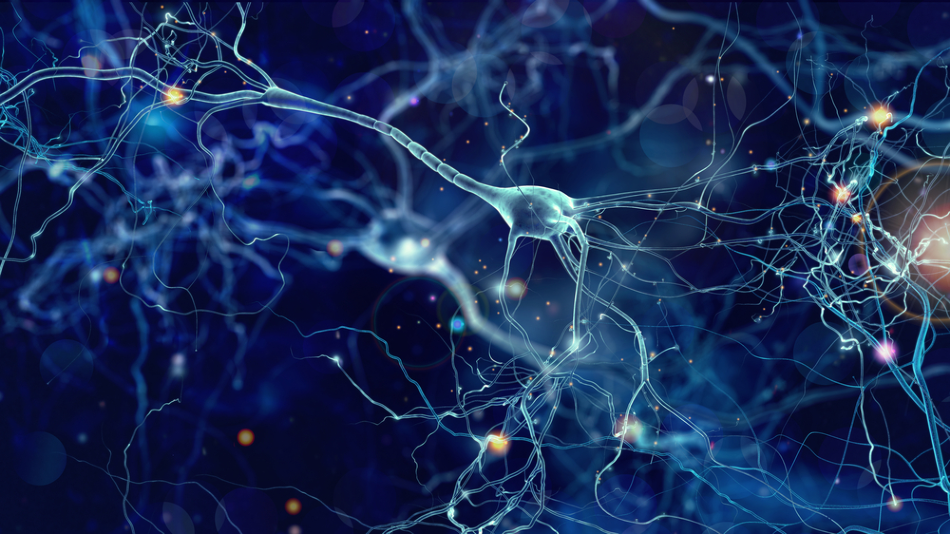
Image Credit: whitehoune/Shutterstock.com
Genetic algorithms are used to train several neural networks that encourage the ability of robots to explore their environment. This method is interesting to explore due to the difficulties faced in employing programming-specific strategies under diverse environments. The method finds an application in the updating and/or construction of internal maps: performing various tasks (such as floor cleaning done by the ROOMBA robot) with the help of limited sensor information.
Genetic algorithms are used to acquire a single competence known as phototaxis, the movement of an organism in response to light. This competence results in allowing the physical robot to perform a task and modify their response via communication. In the simulate-and-transfer mechanism, a controller is developed on the simulator with the help of a genetic algorithm. The resulting solution is then shifted to the robot to enable it to push a box towards a light source. These same algorithms are also called physically embedded genetic algorithms as, essentially, they are embedded in the actual robot – physically instead of virtually, being executed by software personnel.
Basic Principle - Working Examples of Learning Robots Using Genetic Algorithms - The ZORC Project
The major goal of the ZORC project was to train a physical robot to walk. During the training period, the robot is taught to control its servo-motors and process sensor inputs. These complicated processes are accomplished through the implementation of a software system developed using the Genetic Programming paradigm.
Through the Genetic Programming system, a virtual machine-code program can be included with the most basic commands, but also other simple operators such as +,-,*,/ and conditional operators.
ZORC - humanoid robot learning to walk using Genetic Programming
The virtual programs were executed in a physics simulator that controls a ZORC 3D model, after being interpreted. Fitness Function is used to evaluate the program behaviors to determine the effect of programs on the movement and control of the robot.
When these programs are successfully executed in the physical robot, they are executed in the real robot, too. This means that the interpreter stops controlling the simulation of the robot but not the sensors and servos of the actual robot. Eventually, a robust walking algorithm is accomplished and stored in the robot.
Robot Learning based on Cellular Neural Network (CNN) and Genetic Algorithm. Neuroevolution techniqu
Future Work
A large number of robots have to take part in this experimental stage to conclude whether this new distributed learning algorithm can benefit larger robotic colonies. However, the optimal colony size must also be taken into account. Today, experimental results show that the robots acquire either the capability to choose between different behaviors or sensor-motor competences. These experiments need to be further extrapolated to allow the robots the acquisition of both management and behavioral strategies. It is also necessary to analyze and predict whether these experiments can be scaled up by the expansion of the robots’ state space.
This article was updated on 24th February, 2020.