Scientists require a deep understanding of previous studies to identify the best gene/protein targets and the most promising drugs to test to find novel therapies for genetic diseases. However, the amount of biomedical literature is expanding rapidly and frequently contains contradicting data, making it more and more time-intensive for researchers to undertake an exhaustive study.
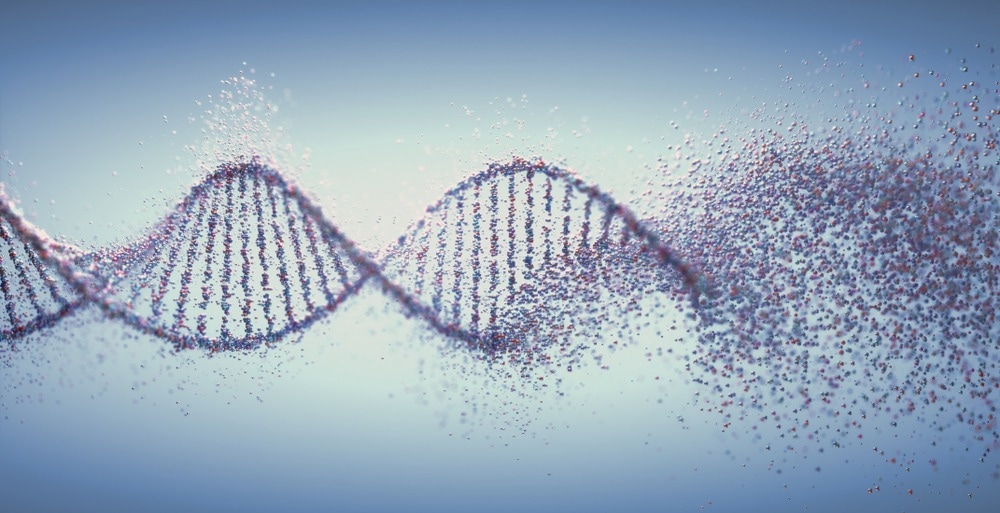
Image Credit: ktsdesign/Shutterstock.com
Cole Deisseroth, a graduate student in the MD./Ph.D. program who works with Dr. Huda Zoghbi and Dr. Zhandong Liu at the Jan and Duncan Neurological Research Institute (Duncan NRI) at the Texas Children's Hospital and Baylor College of Medicine, oversaw a study to create an NLP tool called PARsing ModifiErS via Article aNnotations (PARMESAN) to address this problem.
This new tool can search for current information, compile it into a single knowledge base, and even forecast possible medications that might repair certain protein imbalances. A description of the instrument and its capabilities was just published in the American Journal of Human Genetics.
PARMESAN offers a wonderful opportunity for scientists to speed up the pace of their research and thus, accelerate drug discovery and development.
Dr. Huda Zoghbi, Distinguished Service Professor, Baylor College
This AI-powered application searches public biomedical literature sources (PubMed and PubMed Central) for descriptions of gene-gene and drug-gene regulatory connections and ranks them. What distinguishes PARMESAN in particular is its ability to use curated information to forecast previously unknown associations.
The unique feature of PARMESAN is that it not only identifies existing gene-gene or drug-gene interactions based on the available literature but also predicts putative novel drug-gene relationships by assigning an evidence-based score to each prediction.
Dr. Zhandong Liu, Chief, Computation Sciences, Texas Children’s Hospital
PARMESAN's artificial intelligence algorithms examine studies that detail the contributions of numerous participants in a multistep genetic process. Then, for each reported interaction, it provides a weighted numerical score. Interactions that are consistently and often reported in the literature obtain higher values, whereas interactions that are either weakly supported or appear to be contradicted between studies receive lower scores.
PARMESAN presently predicts over 18,000 target genes, and benchmarking tests indicate that the highest-scoring predictions are more than 95% correct.
By pinpointing the most promising gene and drug interactions, this tool will allow researchers to identify the most promising drugs at a faster rate and with greater accuracy.
Cole Deisseroth, Medical Scientist Training Program (MD/PhD), Baylor College
Won-Seok Lee, Jiyoen Kim, Hyun-Hwan Jeong, Ryan S. Dhindsa, and Julia Wang. They were affiliated with one or more of the following institutions: Baylor College of Medicine, the Jan and Dan Duncan Neurological Research Institute at Texas Children’s Hospital, the University of Pennsylvania, and Howard Hughes Medical Institute.
The study was supported by the National Institutes of Health (NIH), the Medical Scientist Training Program of Baylor College of Medicine, the Robert and Janice McNair Foundation M.D./Ph.D. Student Scholar Program, the Howard Hughes Medical Institute International Student Research Fellowship, the BrightFocus Foundation, the JPB Foundation, the CHDI Foundation, the Huffington Foundation, and the Ting Tsung and Wei Fong Chao Foundation were the others involved in the study.
Journal Reference
Deisseroth, C. A., et al. (2023) Literature-based predictions of Mendelian disease therapies. American Journal of Human Genetics. doi:10.1016/j.ajhg.2023.08.018
Source: https://www.texaschildrens.org/