A Digital Twin is a virtual representation of a physical product, process, or service that enables real-time data flow between the virtual and physical spaces. They are seen as one of the driving technologies behind the ongoing digital transformation of traditional manufacturing and industrial practices within Industry 4.0.
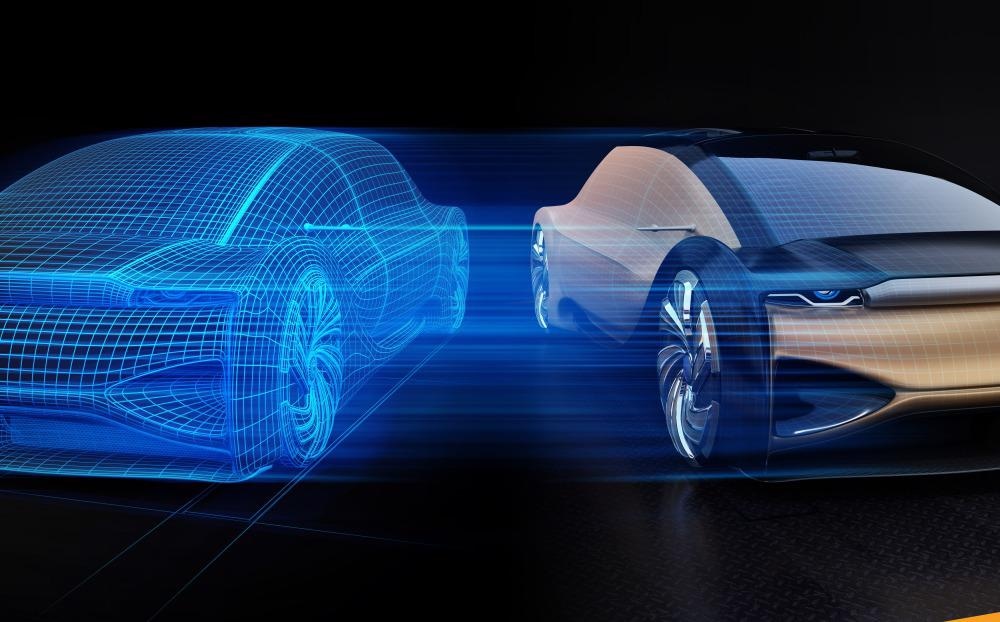
Image Credit: Chesky/Shutterstock.com
The Digital Twin concept is commonly attributed to Dr. Michael Grieves, who introduced the idea in 2002 through a proposed model as part of his product lifecycle management (PLM) research at the University of Michigan.
The model, which had numerous names over subsequent years, was finally called a Digital Twin in 2010 by John Vickers of NASA. However, its underlying concepts remained unchanged: the physical entity, the virtual entity, and the bidirectional data connection.
Despite the established concept of Digital Twins, their widespread application and rapid progression have only recently been made viable due to the advances in Internet of Things (IoT), machine learning, and artificial intelligence (AI). This is likely to continue, with Accenture and McKinsey both listing Digital Twins as a top-five technology trend for 2021 and Forbes citing it as one of the defining technologies of the next decade.
Defining a Digital Twin
The diverse range of Digital Twin research and potential applications has resulted in a variety and vagueness to its definition. Wright and Davidson (2020) foresee this as conceivably contributing to the ‘plateau of productivity’ for the technology falling well below its true potential. It is, therefore, worthwhile to look for a rigorous definition, one that is applicable irrespective of the industry and application, and consider some distinctions that are often overlooked.
An example of such a definition is that proposed by Singh et al. (2021):
A Digital Twin is a dynamic and self-evolving virtual model or simulation of a real-life subject or object (part, machine, process, human, etc.) representing the exact state of its physical twin at any given point of time via exchanging the real-time data as well as keeping the historical data.
Importance should be placed upon the dynamic and self-evolving nature of the virtual model, as this forms the distinction of a Digital Twin from validated models that have been used to great effect for decades. Similarly, the requirement for the physical subject or object to exist should also be noted, as this differs from simple models.
Although this may appear self-evident, overlooking this distinction has occasionally resulted in the misuse of Digital Twins early in design stages (before prototyping), having a detrimental effect on the design process.
Value of a Digital Twin
The reason for the enthusiasm surrounding Digital Twins is due to the extensive advantages they offer in reducing errors, uncertainties, inefficiencies, and expenses in any process, system or object. To give an idea of how such value can be added, a few value-adding examples can be considered.
Monitoring
Digital Twins can detect abnormalities and identify malfunctions in a process or system with far greater accuracy and speed than traditional methods. This is scalable to ‘plant-wide’ applications comprising of hundreds of thousands of components with no significant reduction in performance.
Predicting
Progressing from monitoring, Digital Twins can predict future problems and paths of fault propagation to provide accurate references for maintenance planning and logistical support. Similarly, the maintenance action itself can be simulated plant-wide, and an optimized maintenance strategy is proposed.
Managing
Through complete information transparency, a Digital Twin can continuously optimize the process or system. For example, asset management strategies can be improved to give resource utilization rates previously prevented by the lengthy and costly traditional methods.
Other benefits include waste reduction, remote access of Digital Twin to its physical counterpart, enabling safer control for hazardous working environments, and enhanced internal monitoring and control network security.
Applications of Digital Twins
Use cases for Digital Twins are plentiful. Starting in aerospace and manufacturing sectors, they can now be found in agriculture, healthcare, construction, and robotics sectors.
For example, Xu et al. (2021) developed a Digital Twin-based industrial cloud robotics (DTICR) framework for the control of industrial robots (IRs).
The virtual space of the Digital Twin bridged the gap between the physical IRs and the cloud robotics services, enabling an excellent sensing control of a robotic system through seamlessly synchronizing virtual and physical IRs with a bidirectional data connection. The DTICR framework proved flexible and scalable, promising its suitability for the increasingly complex and varied uses of robotics.
Angin et al. (2020) showed that adopting a Digital Twin approach within the agriculture sector enables constant monitoring of soil parameters, automated detection of crop diseases, and prescription fertilizer treatment plans. The study shows that this approach within agriculture is critical in achieving the high-yield crop production required in the future.
Why digital twins will be the backbone of industry in the future
Video Credit: Siemens/YouTube.com
Remaining Challenges
Realizing an immense technology like Digital Twinning understandably has its challenges, and as the technology is still considered in its infancy, many of these challenges remain.
The process of developing the high-fidelity computer models with appropriate simulation processes, the embedding of sensors with high-capacity IT infrastructure, and facilitating the required data processing and storage, all represent costly and time-consuming investments. So much so that West and Blackburn (2017) predicted the cost of fully implementing a Digital Twin into the Next Generation Air Dominance aircraft for the U.S Air Force, as between $1 and $2 trillion and taking approximately 250 years.
The concept of data ownership brings another, more contentious challenge into view. As Digital Twins often aim to capture all environmental parameters through their connected sensors exhaustively, it is highly likely that such parameters will relate to people’s lives and their intellectual property in some fashion. This poses a major challenge in how this information and data should be shared, and who indeed the true owner is.
The Future of Digital Twins
Digital Twins unquestionably have the potential to profoundly impact how we manufacture products, grow crops, treat diseases, ultimately, how we live our lives. Yet vast amounts of future research and development are required before their potential is realized.
Directions of future research were suggested by Jones et al. (2020) from their comprehensive literature review. These included undertaking validation studies to give a tangible understanding of the perceived benefits, potentially justifying the significant investment and building much-needed trust in the technology.
Teng et al. (2021) suggested that research attention should focus on data communication and infrastructure problems instead of making data-driven models. Researchers state that this is imperative given the rise in enabling technologies such as 5G developments, IoT standardization, AI, and blockchain 3.0, which will all accelerate the development and feasibility of Digital Twins.
Interview: Assembling the Foundations of Soft Robot Systems with Worm Blobs.
References and Further Reading
Angin, P., Anisi, M., Goksel, F., Gursoy, C. and Buyukgulcu, A., (2020). AgriLoRa: A Digital Twin Framework for Smart Agriculture. Journal of Wireless Mobile Networks, Ubiquitous Computing, and Dependable Applications (JoWUA), 11(4), pp.77-96. Available at: https://doi.org/10.22667/JOWUA.2020.12.31.077
Jiang, Y., Yin, S., Li, K., Luo, H. and Kaynak, O., (2021) Industrial applications of digital twins. Philosophical Transactions of the Royal Society A: Mathematical, Physical and Engineering Sciences, 379(2207), p.202-360. Available at: https://doi.org/10.1098/rsta.2020.0360
Jones, D., Snider, C., Nassehi, A., Yon, J. and Hicks, B., (2020) Characterising the Digital Twin: A systematic literature review. CIRP Journal of Manufacturing Science and Technology, 29, pp.36-52. Available at: https://doi.org/10.1016/j.cirpj.2020.02.002
Marr, B., (2021) These 25 Technology Trends Will Define The Next Decade. [online] Forbes. Available at: https://www.forbes.com/sites/bernardmarr/2020/04/20/these-25-technology-trends-will-define-the-next-decade/?sh=39fc580029e3
Singh, M., Fuenmayor, E., Hinchy, E., Qiao, Y., Murray, N. and Devine, D., (2021) Digital Twin: Origin to Future. Applied System Innovation, 4(2), p.36. Available at: https://doi.org/10.3390/asi4020036
Teng, S., Touš, M., Leong, W., How, B., Lam, H. and Máša, V., (2021) Recent advances on industrial data-driven energy savings: Digital twins and infrastructures. Renewable and Sustainable Energy Reviews, 135, p.110-208. Available at: https://doi.org/10.1016/j.rser.2020.110208
West, T. and Blackburn, M., (2017) Is Digital Thread/Digital Twin Affordable? A Systemic Assessment of the Cost of DoD’s Latest Manhattan Project. Procedia Computer Science, 114, pp.47-56. Available at: https://doi.org/10.1016/j.procs.2017.09.003
Wright, L. and Davidson, S., (2020) How to tell the difference between a model and a digital twin. Advanced Modeling and Simulation in Engineering Sciences, 7(1). Available at: https://doi.org/10.1186/s40323-020-00147-4
Xu, W., Cui, J., Li, L., Yao, B., Tian, S. and Zhou, Z., (2021) Digital twin-based industrial cloud robotics: Framework, control approach and implementation. Journal of Manufacturing Systems, 58, pp.196-209. Available at: https://doi.org/10.1016/j.jmsy.2020.07.013
Disclaimer: The views expressed here are those of the author expressed in their private capacity and do not necessarily represent the views of AZoM.com Limited T/A AZoNetwork the owner and operator of this website. This disclaimer forms part of the Terms and conditions of use of this website.