Data science is a key part of efforts to tackle humanitarian and development challenges worldwide. The increasingly plentiful nature of all manner of data sources is combined with increasingly powerful algorithms to bring artificial intelligence (AI) and machine learning (ML) to bear on some of the world’s most pressing concerns.
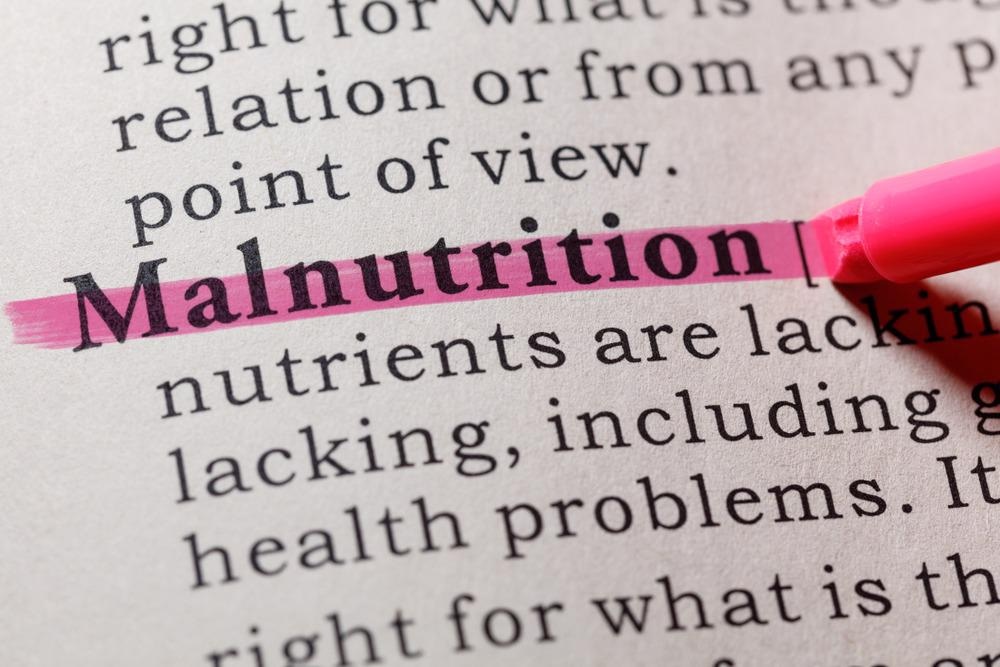
Image Credit: Feng Yu/Shutterstock.com
ML is helping agencies target poverty and malnutrition, monitor these issues, and provide an early warning system to enable preventative measures to be put into place.
Using ML to Treat Child Malnourishment in some of São Paolo’s Poorest Neighborhoods
Researchers from the Universities of Notre Dame (U.S.) and a nonprofit in São Paolo (Brazil) are collaborating on an ML project that will help to combat child nutrition in São Paolo. The project combines the social sciences and psychology with cutting-edge technology.
Brazil’s dietary changes are reflective of its economic growth, as more heavily processed foods containing more sugar and additives have gained more share of the food market from people with more available money and less time.
And, with 85% of Brazil’s population living in cities and massive inequality and areas of deep poverty, many people in the country lack access to food. This has caused children’s growth to be stunted in the poorest areas.
It is not safe for children to go outside and play in many neighborhoods in Brazilian cities due to the extremely high crime rate in those areas. Even if the streets were safe, there is little access to recreation space and exercise facilities.
In São Paolo, a nonprofit treating child malnourishment – the Centre for Nutritional Recovery and Education (CREN) – is operating in the poorest neighborhoods.
CREN is collaborating with the University of Notre Dame’s Interdisciplinary Center for Network Science & Applications (iCeNSA) to use ML to help treat some of the children suffering from malnourishment. Using a psychologically informed approach, the team hopes to spread attitudes and beliefs through local networks of children and families, so that over time they will create lasting, sustainable change.
The partnership is developing an assessment system to evaluate children’s nutritional and wellness risk profiles using ML-based algorithms. The system also provides personalized treatment plans, which developers anticipate may be replicated worldwide.
Using ML, the researchers are personalizing nutrition and children’s health with a practice that is informed by the local context. Their method helps care providers contextualize the socio-ecological model – the web of social and ecological determinants of health – around a child they are treating for malnutrition.
The method sends children’s electronic medical records data to a secure server. There, ML-based modeling and analysis are applied to provide information about children’s environment, lifestyle, family, and the wider community.
The program then creates a risk profile that care providers can use to make holistic treatment plans taking into account children’s physical, psychological, and socio-economic needs.
Combining psychology with AI and ML to tackle social problems is based on the rationale that malnutrition is often caused by social and psychological factors unique to individuals and by lack of access to healthy food.
Complex factors like depression, parental engagement, and family stress also contribute to child malnutrition. ML can work on patient data to identify more accurately how these factors are linked and how they can be managed.
The analytical tools applied to datasets in this project have shown a three-dimensional picture of child malnutrition. All relevant factors are shown to interact with each other and the root problem in dynamic and complex ways.
This perspective also helps to highlight underlying problems that seem unrelated to hunger but need to be addressed before attempting to improve nutrition.
The scientists behind this project hope to demonstrate an almost causal relationship between psychological markers and child malnutrition outcomes.
They are also developing a digital application to help workers collect patient data and intend to expand the project to another nonprofit in Brazil before introducing it globally.
iMapNut: Improving and Analyzing Data on Nutrition
The University of Cambridge’s Department of Archaeology works with ML to improve data integration on children’s nutrition for researchers and policymakers. Their innovative project, iMapNut, aims to develop capacity in WASH (water, sanitation and hygiene) data collection.
The project uses diverse methods like surveys, health care facilities, crowdsourced mobile app data, satellite imagery, and weather data to gather data, which is analyzed with advanced tools such as ML and geospatial mapping techniques.
Neural networks, cluster algorithms, and predictive regression algorithms are applied to data to create site-specific predictive algorithms on the risks of child malnutrition.
iMapNut also integrates socio-economic data with food security information and other factors.
Improving Crop Yield with ML
Technology firm Omdena recently piloted an ML-based system to predict crop yields in Senegal. The pilot used satellite images from Google Earth and applied deep learning algorithms to predict yields.
From Farm to Fork: Cutting Down on Food Waste with Machine Learning
References and Further Reading
Department of Archaeology (2022) iMapNut: Machine Learning to Map and Address Causal Factors of Child Malnutrition in Low- and- Middle- Income Countries. University of Cambridge. [online] Available at: https://www.arch.cam.ac.uk/research/projects/current-projects/imapnut-machine-learning-map-and-address-causal-factors-child.
Rowland, A. (2021). Using ‘Machine Learning’ to Combat Child Malnutrition. University of Notre Dame. [online] Available at: https://kellogg.nd.edu/news/using-%E2%80%98machine-learning%E2%80%99-combat-child-malnutrition.
Masson-Forsythe, M. (2021). Crop Yield Prediction Using Deep Neural Networks and LSTM. Omdena. [online] Available at: https://omdena.com/blog/deep-learning-yield-prediction/.
Disclaimer: The views expressed here are those of the author expressed in their private capacity and do not necessarily represent the views of AZoM.com Limited T/A AZoNetwork the owner and operator of this website. This disclaimer forms part of the Terms and conditions of use of this website.