Drones, also known as unmanned aerial vehicles (UAVs) or small unmanned aircraft (SUAs) have seen rapid technological advancement in recent decades. This has resulted in an ever-growing array of applications such as aerial surveillance, rescue operations, border patrol tasks, and precision farming
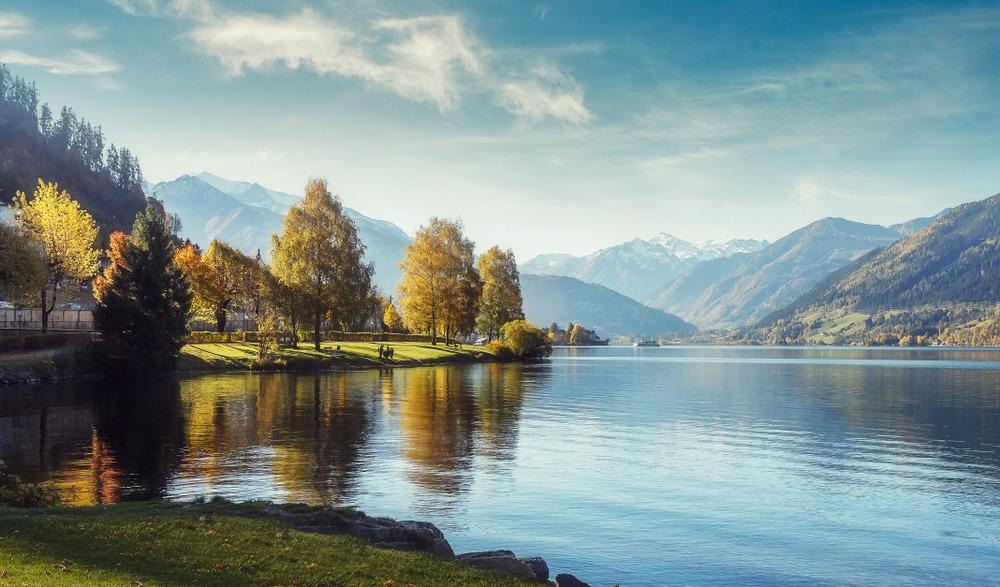
Image Credit: Yevhenii Chulovskyi/Shutterstock.com
Drones are also being used more frequently in the fields of resource and environmental observation as they can track quick changes in environmental variables, including plant growth, hydrological processes, and destruction in the aftermath of catastrophic weather events like hurricanes.
Drones provide scientists with in-depth information and verification of vegetation change, flow measurements, and at-risk areas for floods in aquatic ecosystems, as well as data from unreachable ranges of freshwater and coastal systems in a cost-effective manner.
However, such progress in water quality assessment is extremely desirable, particularly for large-scale routine monitoring programs.
The goal of a new study published in the journal Science of The Total Environment was to see if a drone-deployed payload could: i) accurately collect physiochemical data and water samples, ii) be of sufficient volume to meet the needs of large-scale water quality management programs like the WFD, and iii) produce similar water chemistry and physiochemical outcomes to traditional boat water sampling.
Methodology
From September to November 2019, field trials were held at Ballquirke Lake and Loughs Fee, Inagh, Conn, Derg, and Mask in the west of Ireland.
Three water samples were taken at each of the 12 locations using each of the two collection techniques, conventional boat-based water sampling and drone-based water sampling, for a total of 72 water samples to compare water chemistry parameters between the two sampling techniques and to examine the variability associated with each sampling techniques. Table 1 reveals the information.
Table 1. Key characteristics of lakes sampled during field trials. Source: Graham, et al., 2022
|
No. of sampling locations |
Co-ordinates for sampling locations |
Lake surface area (ha) |
Maximum depth (m) |
Altitude (m) |
Water framework directive alkalinity statusa |
Water framework directive typology classa |
WFD statusb |
Lough Fee |
1 |
53.59122
–9.8381 |
174 |
23 |
60 |
– |
– |
– |
Lough Inagh |
1 |
53.5162
–9.73816 |
310 |
24 |
21 |
– |
– |
– |
Lough Conn |
2 |
53.9898
–9.25791
53.09365
–9.29682 |
4704 |
33.8 |
20 |
High |
12 |
Moderate |
Ballyquirke lake |
2 |
53.32469
–9.15257
53.32603
–9.1543 |
73.6 |
12.2 |
15 |
Moderate |
6 |
Bad |
Lough Derg |
3 |
52.90733
–8.50461
52.92032
–8.45241
52.91859
–8.45476 |
13,000 |
34 |
40 |
High |
12 |
Moderate |
Lough Mask |
3 |
53.56779
–9.41073
53.56526
–9.41522
53.64387
–9.36527 |
8218 |
17 |
58 |
High |
12 |
Good |
a Data taken from Inland Fisheries Ireland National Research Survey Programme Fish Stock Assessments 2015 & 2016 (Kelly et al., 2016; Kelly et al., 2017, Kelly et al., 2017; McLoone et al., 2017).
b Data taken from the EPA Maps portal (EPA, 2019).
To eliminate any biosecurity hazards, all sampling equipment was cleaned with distilled water before each consecutive sample collection, and all field equipment was cleaned with Virkon Aquatic.
The data from the last 90 seconds of the 4-minute period were kept for the physico-chemical parameters to guarantee that the probes had enough time to adapt from capturing data while in flight.
The coefficient of variation was determined from the three samples at each sampling location for each parameter and sampling mode to see if the variability and clarity differed between boat and drone acquired data.
The non-parametric Wilcoxon signed-rank tests were used to analyze data that were non-normally generated and showed heterogeneous variability, even after suitable treatment.
Results and Discussion
Drone water sampling collected 2 L of water from each of the 36 sampling flights. No significant differences between the conventional boat and drone water sampling techniques for alkalinity, hardness, true color, silica, TON, TP, Chl-a, and permeability (Figure 1) were revealed by the paired sample t-tests.
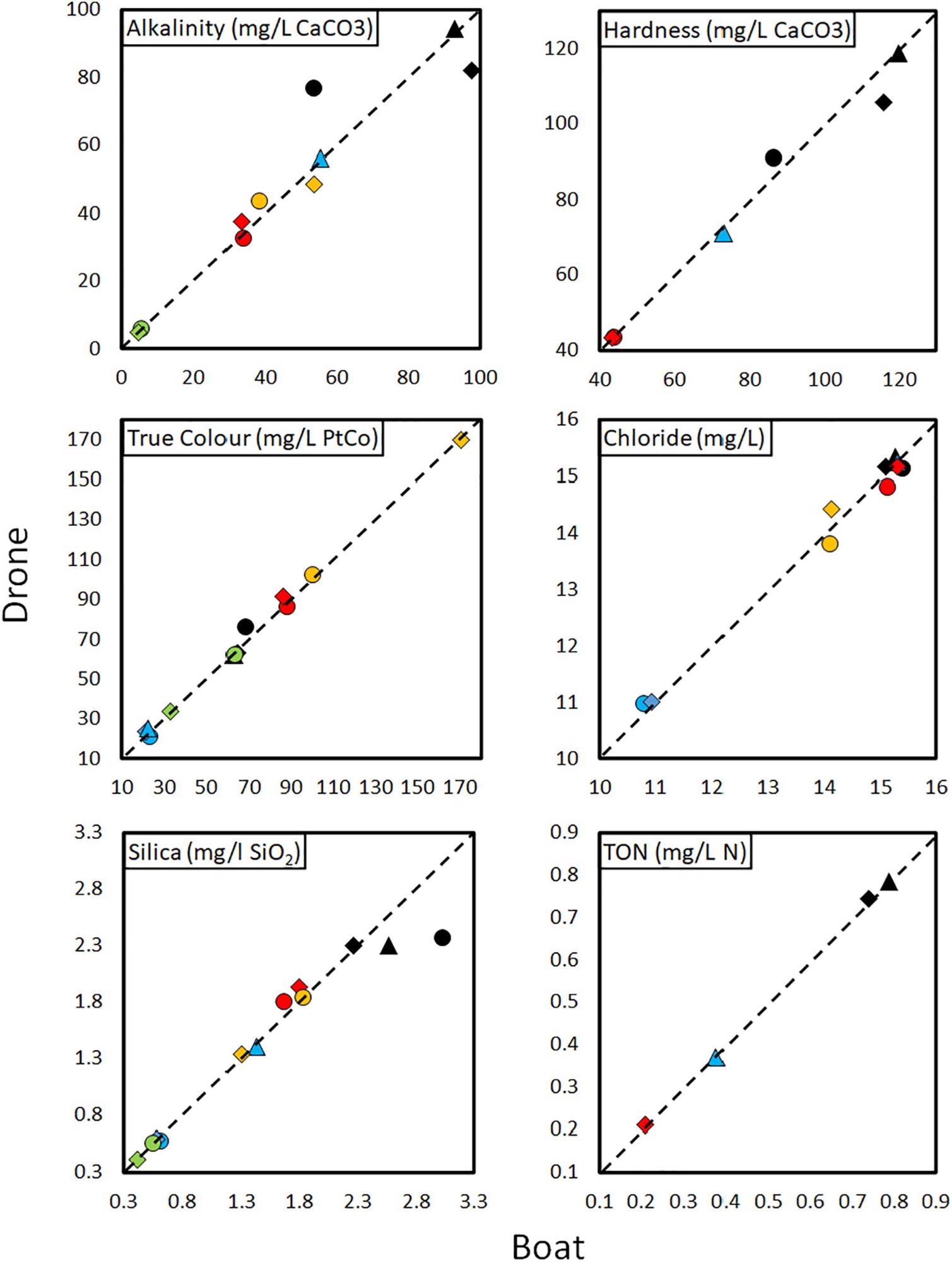
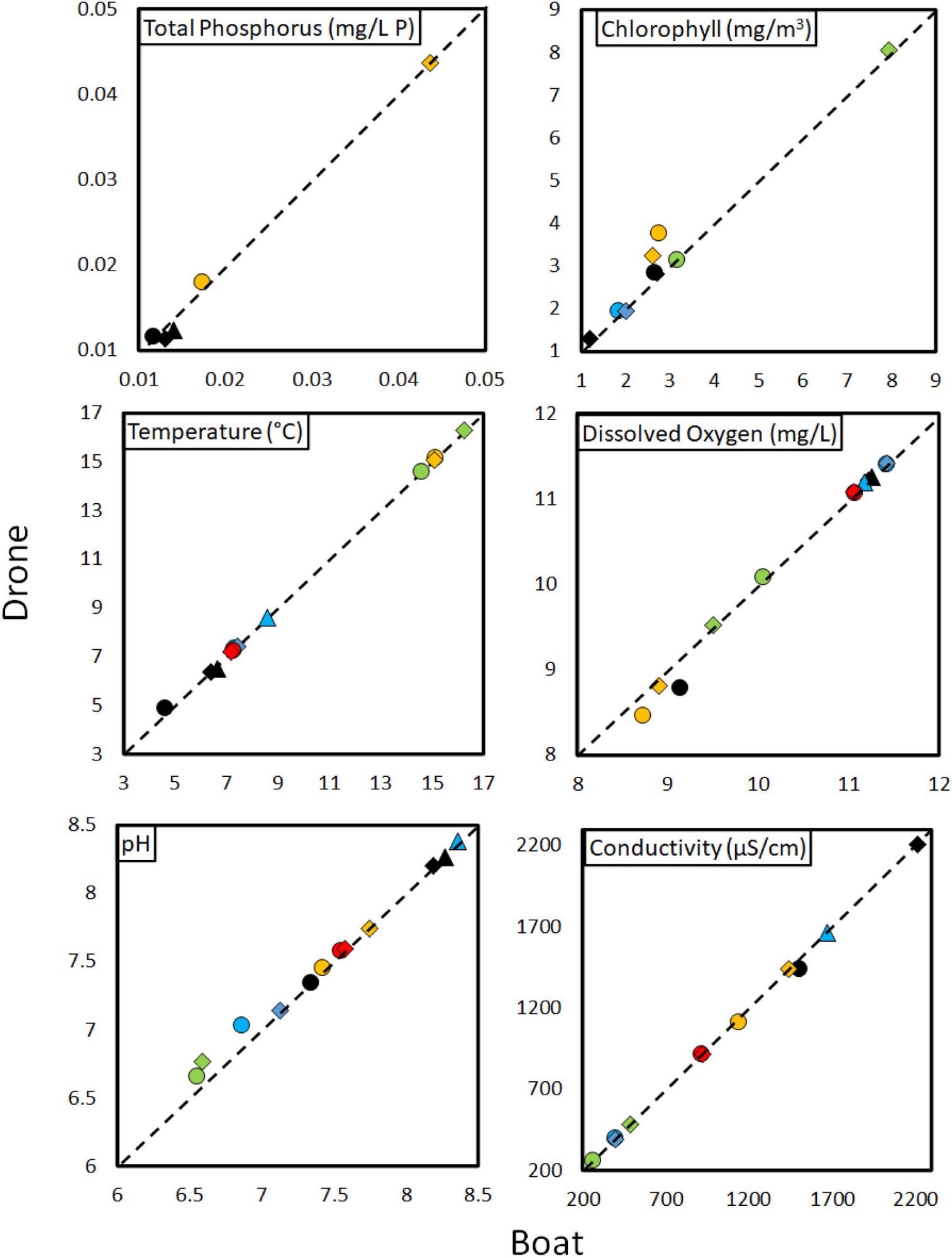
Figure 1. Comparison of water chemistry variables collected using traditional boat (x-axis) and drone (y-axis) water sampling. Image Credit: Graham, et al., 2022.

A statistical analysis of variability discovered no statistically significant difference in total variability between the information recorded by drone and boat water sampling techniques. Furthermore, the accuracy of each variable revealed only one substantial difference, for hardness, with greater variability in boat collected samples than in drone collected samples (Table 2).
Table 2. Results of paired t-test and Wilcoxon-signed rank tests used to assess precision, using the coefficient of variation (CV%), between water chemistry variables at each sampling location between paired samples collected via traditional boat and drone water sampling methodologies. Significant differences are highlighted in bold. Source: Graham, et al., 2022
Variable |
Test statistic |
No. of pairs |
p value |
Average CV% |
Boat |
Drone |
True coloura |
–1.54 |
12 |
0.15 |
2.9 |
4.3 |
Hardness |
2.87 |
6 |
0.043 |
7.3 |
3.9 |
Silicaa |
–0.89 |
12 |
0.4 |
7.6 |
8.7 |
TONa |
–0.19 |
4 |
0.86 |
1.3 |
1.6 |
Chloride |
–0.15 |
10 |
0.89 |
0.9 |
0.9 |
Alkalinityb |
–0.77 |
10 |
0.44 |
6.6 |
9.4 |
Chlorophyll-a |
–1.25 |
8 |
0.25 |
6.4 |
8.9 |
TPb |
–0.41 |
5 |
0.69 |
6.9 |
5.9 |
pHb |
–1.81 |
12 |
0.07 |
1.1 |
0.6 |
Temperatureb |
–1.73 |
12 |
0.08 |
0.6 |
0.4 |
Conductivitya |
0.08 |
12 |
0.94 |
0.6 |
0.6 |
DO |
0.2 |
12 |
0.84 |
0.5 |
0.5 |
TON = Total oxidised nitrogen, TP = Total phosphorous, DO = dissolved oxygen.
a Square root transformed.
b Wilcoxon signed rank test.
This study revealed the significant ability of drones to effectively and consistently collect large volumes of water in a manner appropriate for large water monitoring programs such as the EU WFD, National Aquatic Resource Surveys in the United States, and the United Nations Global Environment Monitoring System for Freshwater.
While no substantial variations in any of the water chemistry variables calculated in the laboratory were found between samples collected by boat and drone, there was a statistically significant difference in pH captured on the data logging EXO Sonde.
While many researchers hope to advance drone technology for water sampling, there is a remarkable lack of statistical comparisons of water chemistry parameters between conventional boats and drone-assisted water sampling in the literature. This is likely due to limited sample size and reproduction.
It is crucial that adequate volumes of water can be gathered by tailored payloads deployed by drones, and that these amounts of water can be captured reliably for drone technology to be applied meaningfully in large-scale water monitoring projects. Doing so will help to realize their full potential to collect water samples cleaner and more effectively.
Early studies in this field, which considerably developed the use of drone technology in water sampling, had varying degrees of success in catching the quantity of water for which they were constructed.
Conclusion
In conclusion, this study shows that drone technology can be used to collect high precision, exact water physiochemical data and large volume samples from aquatic ecosystems reliably and safely, meeting many of the requirements of large-scale water monitoring programs.
This research systematically overcame many important technological limitations in the implementation of drone technology, including the relatively small volume of water retrieved by drones for evaluations, the low rate of success in collecting the desired volume of water, and, most importantly, the clear discrepancies in water chemistry results of previous studies between drone and conventional boat water sampling techniques.
As a result, drone technology could be used to gather water samples from lakes in a more dependable, faster, and cost-effective manner than traditional techniques such as using boats, which is safer for professionals and reduces biosecurity risk.
More research is needed to determine whether drone technology can be used to collect water samples for evaluations of priority substances, typically gathered in specialized glass vessels. As well as this, the capacity and cost-benefit analyses of using drone technology to gather large volume water samples in remote areas that are unreachable using conventional boat sampling must be assessed.
Journal Reference:
Graham, C. T., O’Connor, I., Broderick, L., Broderick, M., Jensen, O., Lally, H. T. (2022) Drones can reliably, accurately and with high levels of precision, collect large volume water samples and physio-chemical data from lakes. Science of The Total Environment, 824, p. 153875. Available Online: https://www.sciencedirect.com/science/article/pii/S0048969722009676.
References and Further Reading
- Bandini, F., et al. (2017) Measuring water level in rivers and lakes from lightweight unmanned aerial vehicles. Journal of Hydrology, 548, pp. 237–250. doi.org/10.1016/j.jhydrol.2017.02.038.
- Banerjee, B.P., et al. (2018) Development of a UAV-mounted system for remotely collecting mine water samples. International Journal of Mining, Reclamation and Environment, 34(6), pp. 1–12. doi.org/10.1080/17480930.2018.1549526.
- Beloev, I H (2016) A review on current and emerging application possibilities for unmanned aerial vehicles. Acta Technologica Agriculturae, 3, pp. 70–76.doi.org/10.1515/ata-2016-0015.
- Benson, J., et al. (2019) Microorganisms collected from the surface of freshwater lakes using a drone water sampling system (DOWSE). Water, 11(1), p. 157. doi.org/10.3390/w11010157.
- Butcher, P.A., et al. (2021) The drone revolution of shark science: a review. Drones, 5(1), p. 8. doi.org/10.3390/drones5010008.
- Castendyk, D., et al. (2018) Experiences with autonomous sampling of pit lakes in North America using drone aircraft and drone boats. C. Wolkersdorfer, L. Sartz, A. Weber, J. Burgess, G. Tremblay (Eds.), In: Mine Water – Risk to Opportunity, Tshwane University of Technology, Pretoria, South Africa, pp. 1036-1042. Available at: https://imwa.info/docs/imwa_2018/IMWA2018_Castendyk_1036.pdf
- Castendyk, D.N., et al. (2019) Using aerial drones to select sample depths in pit lakes. Mine Closure, pp. 1113-1126. doi.org/10.36487/ACG_rep/1915_89_Castendyk.
- Castendyk, D., et al. (2020) A validated method for pit lake water sampling using aerial drones and sampling devices. Mine Water and the Environment, 39, pp. 440–454. doi.org/10.1007/s10230-020-00673-y.
- Chabot, D & Bird, D M (2016) Wildlife research and management methods in the 21st century: where do unmanned aircraft fit in? Journal of Unmanned Vehicle Systems, 3(4), pp. 137–155. doi.org/10.1139/juvs-2015-0021.
- Christiansen, F., et al. (2018) Maternal body size and condition determine calf growth rates in southern right whales. Inter-Research Science Publisher - Marine Ecology Progress Series, 592, pp. 267–281. doi.org/10.3354/meps12522.
- Chung, M., et al. (2015) Obtaining the thermal structure of lakes from the air. Water, 7(11), pp. 6467–6482. doi.org/10.3390/w7116467.
- Detweiler, C., et al. (2015) Bringing unmanned aerial systems closer to the environment. Environmental Practice, 17(3), pp. 188–200. doi.org/10.1017/S1466046615000174.
- Doi, H., et al, (2017) Water sampling for environmental DNA surveys by using an unmanned aerial vehicle. Limnology and Oceanography Methods, 15(11), pp. 939–944. doi.org/10.1002/lom3.10214.
- EC (European Commission) Environment (2016) Introduction to the new EU Water Framework Directive. Available at: https://ec.europa.eu/info/index_en
- EC (European Commission) Environment (2017) Our oceans, seas and coasts. Available at: http://ec.europa.eu/environment/marine/eu-coast-and-marine-policy/marine-strategy-framework-directive/index_en.htm
- Geoghegan, J. L., et al. (2018) Virological sampling of inaccessible wildlife with drones. Viruses, 10(6), p. 300. doi.org/10.3390/v10060300
- Greenwood, F., et al. (2020) Flying into the hurricane: a case study of UAV use in damage assessment during the 2017 hurricanes in Texas and Florida. PLoS ONE, 15(2), p. e0227808. doi.org/10.1371/journal.pone.0227808.
- Hogan, S. D., et al. (2017) Unmanned aerial systems for agriculture and natural resources. California Agriculture, 71(1), pp. 5–14. doi.org/10.3733/ca.2017a0002.
- Kelly, F. L., et al. (2016) Fish Stock Survey of Lough Mask, June 2015. In: National Research Survey Programme, Inland Fisheries Ireland, 3044 Lake Drive, Citywest Business Campus, Dublin 24, 2016. Available at: http://wfdfish.ie/wp-content/uploads/2016/09/Mask_2015.pdf.
- Kelly, F. L., et al. (2017) Fish Stock Survey of Lough Derg, June 2016. In: National Research Survey Programme, Inland Fisheries Ireland, 3044 Lake Drive, Citywest Business Campus, Dublin 24, 2017. Available at: http://wfdfish.ie/wp-content/uploads/2017/10/Derg_2016-1.pdf.
- Kelly, F. L., et al. (2017) Fish Stock Survey of Lough Conn, August 2016. In: National Research Survey Programme, Inland Fisheries Ireland, 3044 Lake Drive, Citywest Business Campus, Dublin 24, 2017.
- Koparan, C & Koc, A B (2016) Unmanned aerial vehicle (UAV) assisted water sampling. In: 2016 American Society of Agricultural and Biological Engineers (ASABE), Annual International Meeting, Orlando, Florida, 2016, doi.org/10.13031/AIM.20162461157.
- Koparan, C., et al.(2018a) Evaluation of a UAV-assisted autonomous water sampling. Water, 10(5), p. 655. doi.org/10.3390/w10050655.
- Koparan, C., et al.(2018b) In situ water quality measurements using an unmanned aerial vehicle (UAV) system. Water, 10(3), p. 264. doi.org/10.3390/w10030264.
- Koparan, C., et al. (2019) Autonomous in situ measurements of noncontaminant water quality indicators and sample collection with a UAV. Water, 11(3), p. 604. doi.org/10.3390/w11030604.
- Koparan. C., et al. (2020) Adaptive water sampling device for aerial robots. Drones, 4(1), p. 5. doi.org/10.3390/drones4010005.
- Lally, H. T., et al. (2019) Can drones be used to conduct water sampling in aquatic environments? A review. Science of The Total Environment, 670, pp. 569-575. doi.org/10.1016/j.scitotenv.2019.03.252.
- Lally, H. T., et al. (2020) Assessing the Potential of Drones to Take Water Samples and Physicochemical Data from Open Lakes. In: EPA Research Report. EPA Research Programme 2014-2020, 2020. Available at: http://research.thea.ie/handle/20.500.12065/3459.
- Librán-Embid, F., et al. (2020) Unmanned aerial vehicles for biodiversity-friendly agricultural landscapes-a systematic review. Science of The Total Environment, 732, p. 139204. doi.org/10.1016/j.scitotenv.2020.139204.
- Manfreda,S., et al.(2018) On the use of unmanned aerial systems for environmental monitoring. Remote Sensing, 10(4), p. 641. doi.org/10.3390/rs10040641.
- McLoone, P., et al. (2017) Fish Stock Survey of Ballyquirke Lough, September 2016. In: National Research Survey Programme, Coarse Fish and Pike, Inland Fisheries Ireland, 3044 Lake Drive, Citywest Business Campus, Dublin 24, 2017.
- Niethammer, U., et al. (2012) UAV-based remote sensing of the super sauze landslide: evaluation and results. Engineering Geology, 128, pp. 2–11. doi.org/10.1016/j.enggeo.2011.03.012.
- Ore, J. P., et al. (2013) Autonomous aerial water sampling. Proceedings of the 9th International Conference in Field and Service Robots (FSR), Brisbane, Australia, 5, pp. 137–151.
- Ore, J. P., et al. (2015) Autonomous aerial water sampling. Journal of Field Robotics, 32(8) (2015), pp. 1095–1113. doi.org/10.1002/rob.21591.
- Pajares, G (2015) Overview and current status of remote sensing applications based on unmanned aerial vehicles (UAVs). Photogrammetric Engineering & Remote Sensing, 81(4), pp. 281–330. doi.org/10.14358/PERS.81.4.281.
- Rhee, D. S., et al. (2018) Applications of unmanned aerial vehicles in fluvial remote sensing: an overview of recent achievements. KSCE Journal of Civil Engineering, 22(2), pp. 588–602. doi.org/10.1007/s12205-017-1862-5.
- Samseemoung, G., et al. (2012) An application of low altitude remote sensing (LARS) platform for monitoring crop growth and weed infestation in a soybean plantation. Precision Agriculture, 13, pp. 611–627. doi.org/10.1007/s11119-012-9271-8.
- Schofield, G., et al. (2019) Drones for research on sea turtles and other marine vertebrates—a review. Biological Conservation, 238, p. 108214. doi.org/10.1016/j.biocon.2019.108214.
- Song, K., et al. (2017) Using unmanned aerial vehicles to sample aqautic ecosystems. Limnology and Oceanography Methods, 15(12), pp. 1021–1030. doi.org/10.1002/lom3.10222.
- Stone, H., et al. (2017) The use of emerging technology in post-disaster reconnaissance missions. In: EEFIT Report, Institution of Structural Engineers, London, UK (2017). Available at: https://www.istructe.org/IStructE/media/Public/Resources/report-eefit-grant-emerging-technology-reconnaissance-20170131.pdf
- The, S. K., et al. (2008) Experiments in integrating autonomous uninhabited aerial vehicles (UAVs) and wireless sensor networks. In: Proceedings 2008 Australasian Conference on Robotics and Automation (ACRA 08), Canberra, 2008.
- Terada, A., et al. (2018) Water sampling using a drone at Yugama crater lake, Kusatsu-Shirane volcano, Japan. Earth, Planets and Space, 70(64), pp. 1–9. doi.org/10.1186/s40623-018-0835-3.
- Tierney, D., et al. (2015) Lakes. In: Water Quality in Ireland 2010-2012, Environmental Protection Agency, P.O. Box 3000, Johnstown Castle Estate, County Wexford, Ireland, Y35 W821, 2015.
- Toro, F G & Tsourdos, A (2018) UAV Sensors for Environmental Monitoring. Printed Edition of the Special Issue Published in Sensors. 978-3-03842-754-4.
- Tyler, S., et al. (2018) Perspectives on the application of unmanned aircraft for freshwater fisheries census. Fisheries, 43(11), pp. 510-516 doi.org/10.1002/fsh.10167.
- UN (United Nations) Environment (2019) Monitoring water quality. Available at: https://www.unep.org/
- US (United States) Environmental Protection Agency (EPA) (2018) National aquatic resource surveys. Available at:https://www.epa.gov/national-aquatic-resource-surveys
- Van der Wal, T., et al. (2013) Fieldcopter: unmanned aerial systems for crop monitoring services. Precision Agriculture, 13, pp. 169-175.
- Vergouw, B., et al. (2016) Drone technology: types, payloads, applications, frequency spectrum issues and future developments. The Future of Drone Use. Information Technology and Law Series, 27, pp. 21-45. doi.org/10.1007/978-94-6265-132-6_2.
- Watts, A. C.,et al. (2012) Unmanned aircraft systems in remote sensing and scientific research: classification and considerations of use. Remote Sensing, 4(6), pp. 1671-1692. doi.org/10.3390/rs4061671.
- Wich, S A & Koh, L P (2018) Conservation Drones: Mapping and Monitoring Biodiversity. Oxford University Press, New York. 2018.
- Zhang, C & Kovacs, J M (2012) The application of small unmanned aerial systems for precision agriculture: a review. Precision Agriculture, 13, pp. 693-712. doi.org/10.1007/s11119-012-9274-5.