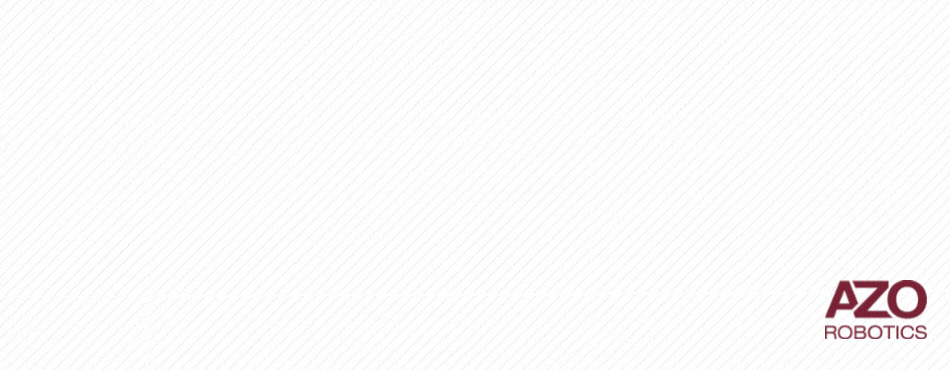
What inspired your research into using artificial intelligence (AI) to help interpret X-Rays?
As a former Radiology deputy editor for MSK, I came to realize the increased interest in AI in MSK imaging, but I never had any experience with AI research or clinical trials.
As an MSK radiologist, I was keen to develop my own knowledge of the use of AI. The opportunity came when a French colleague asked me to help with their AI algorithm which is already quite mature and being used in routine clinical practice in Europe. They wanted to get it approved in the US using US data. This was quite motivating.
I was also motivated by the fact that the algorithm was developed for several body parts. I always thought that the results of this study would be great as part of a study that could be published in a high-impact factor journal.
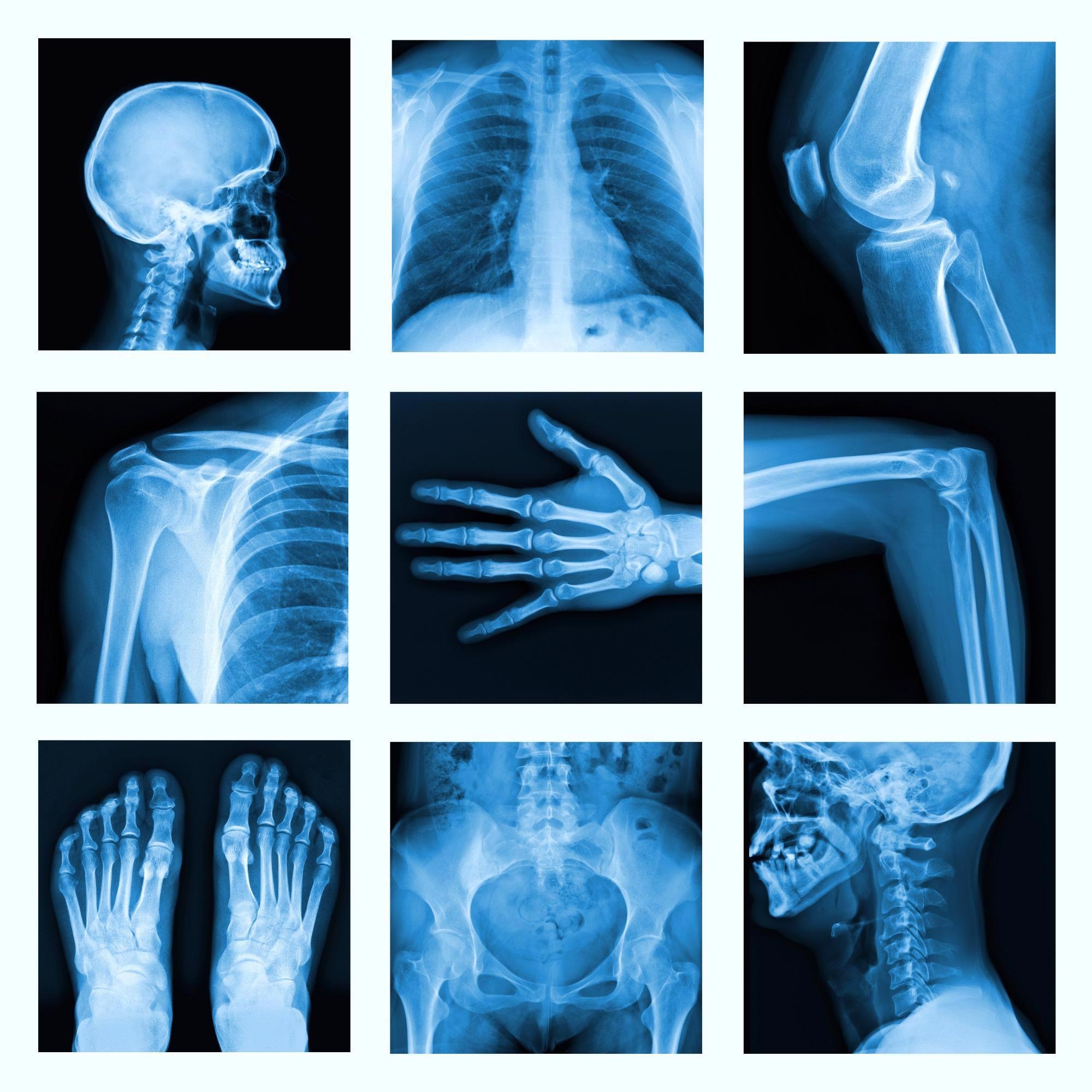
Image Credit: Shutterstock.com/ itsmejust
The aim of this study is particularly relevant in the sense that missed fractures represent a common diagnostic error in Emergency Departments and can lead to several complications for the patients. Also, all fractures are not easy to detect on plain film X-Rays due to multiple factors (workload, fatigue, interruptions, cognitive biases, subtle presentations, etc.).
Currently, the waiting times for emergency room and urgent care clinics can be lengthy, with patients waiting many hours before they can receive treatment. Why are waiting times so long?
There are several bottlenecks in the Emergency room workflow, starting with the high volumes of patients that visit the ER on a daily basis. Imaging is one of them and introduces an additional delay in patient care (from the prescription of the exam to the acquisition of images, to the radiologist’s report).
AI could help in reducing some of these bottlenecks and speed up radiologists’ interpretation of positive cases, and reduce their workload.
Can you give an overview of how your AI algorithm works?
The AI BoneView was developed by GLEAMER. It was trained on accurately annotated X-Ray images of limbs, the pelvis, rib cage and T/L spine. These X-Rays were collected from multiple institutions and acquired on a large variety of systems.
As output, Boneview provides a summary table and replicated images with bounding boxes around regions of interest.
Your AI algorithm can rapidly and automatically detect X-rays that are positive for fractures. How did you teach the algorithm to function in this way?
Teaching the algorithm is the first and most important phase of the process. As with building any AI algorithm, we used a large number of X-Rays with and without fracture, annotated by MSK experts. GLEAMER used 70% of the X-Rays to train the algorithm and the other 20% to test it.
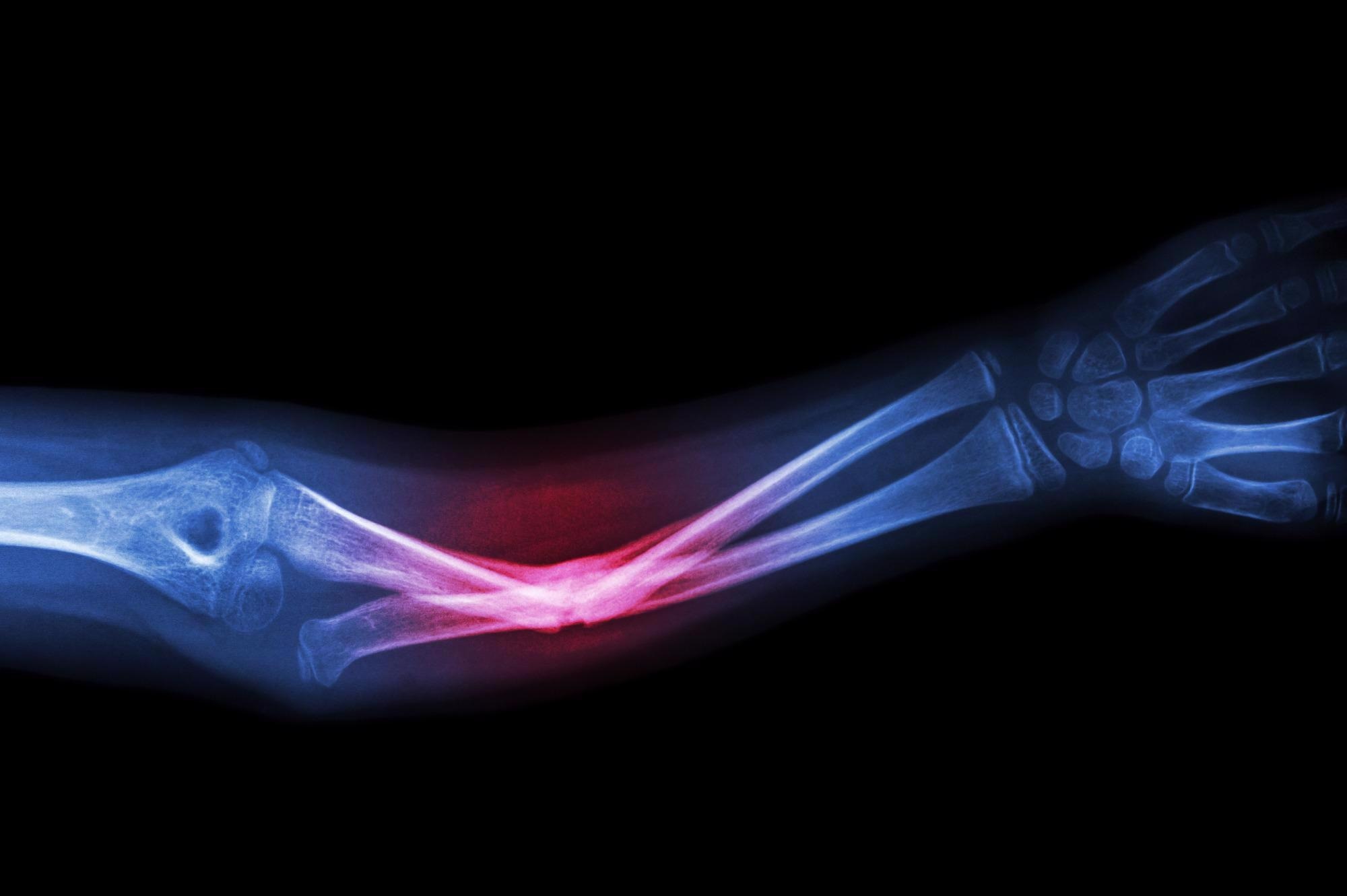
Image Credit: Shutterstock.com/ Puwadol Jaturawutthichai
Finally, once all training was complete, an additional 10% of these images, different from the ones used to train the algorithm, were used to validate it.
Inconsistencies in radiographic diagnoses occur most often at night between the hours of 5 pm and 3 am. Why do you think this is?
The availability of senior readers and MSK subspecialty radiologists is limited at night. Night shifts are mainly covered by junior radiologists or residents in some countries. In others, there will be one senior radiologist at night who has to interpret all specialties.
As such, this radiologist’s focus would be primarily on neuro and body emergencies since they can be life-threatening, while fractures are usually read by residents or other physicians on call, including ER or family medicine physicians.
Moreover, it is well known that fatigue and interruptions increase the probability of errors. The advantage of AI is that it systematically analyzes all X-Rays with the same performance, regardless of the time of the day. Machines do not get tired like their human counterparts.
According to your research, fracture interpretation errors make up to 24% of harmful diagnostic errors seen in the emergency department. How can your AI algorithm be used to reduce errors and inconsistencies in the radiographic diagnosis of fractures?
The AI improves readers’ sensitivity, meaning that it reduces the false-negative rate (i.e. missed fractures) that human error can sometimes provide.
The algorithm can also help to reduce false positives. This can give physicians a better understanding of how to handle patients with fractures that would have been missed. It also avoids physicians wasting crucial time and energy overtreating patients without fractures.
How did you test the efficacy of the algorithm?
To test the efficacy of our algorithm, we conducted a retrospective study that included 480 radiographic examinations of adults over 21 years of age, with indications of trauma and fracture prevalence of 50%.
The radiographs included were of limbs, the pelvis, dorsal spine, lumbar spine and rib cages. The radiographs were obtained from various hospitals and clinics across the United States.
There were 350 fractures in 240 patients and 240 examinations without fractures. The radiographs were analyzed twice (with and without the assistance of Gleamer’s Boneview automatic fracture detection software).
Readers had a 1-month washout period between the two analyses.
There were 24 US board-certified readers from 6 different specialties (4 radiologists, 4 orthopedic surgeons, 4 rheumatologists, 4 emergency physicians, 4 family medicine physicians and 4 emergency physician assistants) with different levels of seniority for radiologists and orthopedic surgeons.
The gold standard was established based on the independent reading of radiographs by two musculoskeletal radiologists. Discrepancies were adjudicated by myself. It was also determined whether the fractures were obvious or not.
The standalone performance of the software on this dataset was evaluated globally, as well as the improvements in the diagnostic performance of the readers in terms of sensitivity, specificity and time-saving. An analysis was also made by the type of reader and by the anatomical location of the fractures.
What were the results of your tests? How did the algorithm compare to expert human readers (subspecialized radiology doctors who are trained to read bone X-rays)?
The results of the study showed a sensitivity increase in the detection of fractures of 10.4%.
With the assistance of the Boneview software, 75.2% of fractures were detected compared to the lower number of 64.8% detected without the assistance of the software (p<0.05).
There was also a 5% increase in specificity in fracture detection when using the software, with specificity increasing from 90.6% without assistance to 95.6% with software assistance (p<0.05).
The reading time of 6.3 seconds per radiograph (p=0.046) was also successfully decreased, with reading time decreasing from 55.5 seconds without assistance to 49.2 seconds with software assistance.
Did you come across any challenges during your research, and if so, how did you overcome them?
Training all 24 readers with various backgrounds on reading with AI was somewhat a unique challenge. On the other hand, the readers were all happy to be part of the study and thought that the use of the AI algorithm was easy, friendly and extremely intuitive. Overall, this research study/trial went well.
Although your research was focused on fracture diagnosis, could this algorithm be applied to other diseases and disorders?
Absolutely. Aside from detecting fractures, GLEAMER can also be enhanced to expand the types of findings the AI can detect: in Europe, the CE-marked version can already highlight dislocations, effusions and bone lesions on top of fractures.
We firmly believe the same technology can be applied to different types of findings to enrich the output of the AI algorithm when assessing trauma X-Rays.
What are the next steps for your research?
The next steps of our research involve validating these new versions of the algorithm on other types of findings and using different body parts. We will then take these validated new versions of the AI algorithm and again use data from across the United States to demonstrate its generalizability. The future is exciting in terms of our research possibilities.
About Dr. Ali Guermazi
Dr. Guermazi is a Professor of Radiology and Medicine, Assistant Dean of Diversity and Inclusion and Director of the Quantitative Imaging Center (QIC) at Boston University School of Medicine. Dr. Guermazi’s interest is musculoskeletal diseases, in particular, the diagnosis and disease progression assessment of osteoarthritis using MRI. His work has focused on identifying structural risk factors for developing and worsening osteoarthritis. Dr. Guermazi had been involved in developing several original and widely accepted radiological methods to assess osteoarthritis disease risk and progression, including the WORMS, BLOKS and MOAKS for the knee, HOAMS for the hip and fixed-flexion radiography for measuring joint space width. Dr. Guermazi has been involved as an MRI reader for the past 22 years in several large U.S. studies, including the Health Aging and Body Composition (Health ABC) study, the Boston Osteoarthritis Knee study (BOKS), the Multi-center Osteoarthritis STudy (MOST), the Framingham study, Osteoarthritis Initiative (OAI), and other large NIH-funded studies, as well as several Pharmaceutical-sponsored clinical trials. He is the author of over 610 peer-reviewed publications and an Investigator on numerous research grants related to MRI reading for osteoarthritis. Dr. Guermazi was Deputy Editor of RADIOLOGY from 2013-2019 and is currently Vice Editor-in-Chief of Skeletal Radiology.
Disclaimer: The views expressed here are those of the interviewee and do not necessarily represent the views of AZoM.com Limited (T/A) AZoNetwork, the owner and operator of this website. This disclaimer forms part of the Terms and Conditions of use of this website.