The logistics, supply chain, and warehouse sectors are undergoing significant digital transformation. The large amounts of data associated with this transformation require advanced analytics as a result. In this article, AZoRobotics discusses how autonomous warehouses are managing big data.
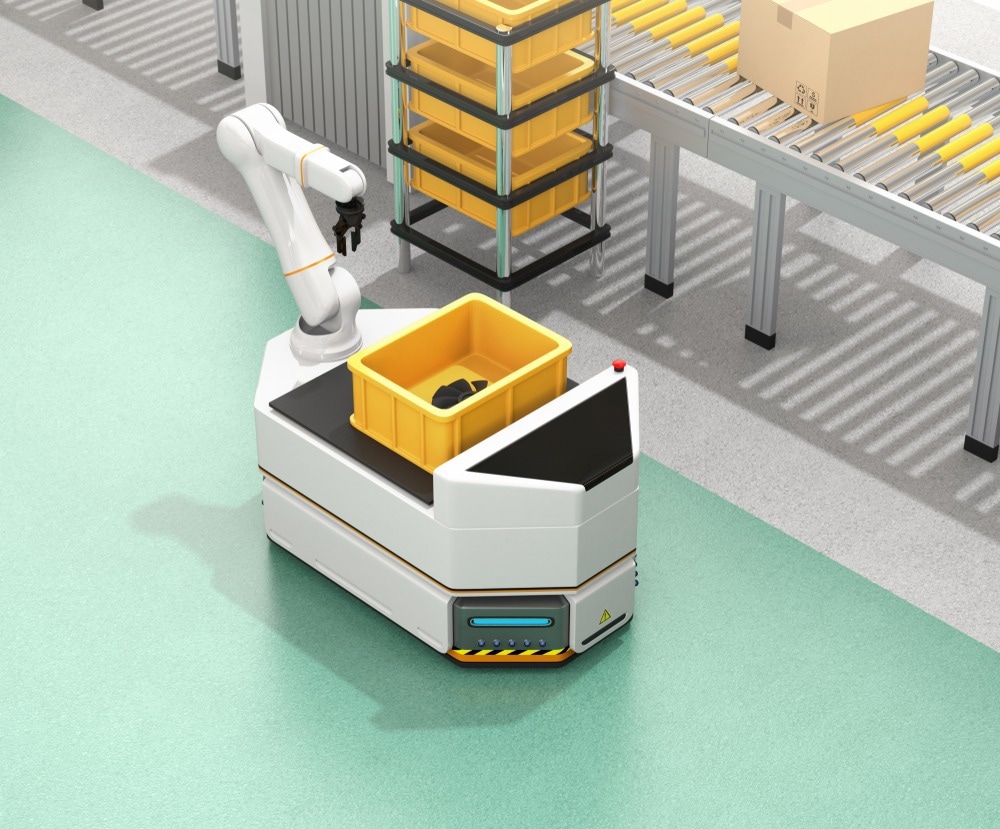
Image Credit: Chesky/Shutterstock.com
The Rise of Big Data Sets
Robots and autonomous vehicles, Industrial Internet of Things (IIoT) networks, and big data analytics are all being brought to bear on the industry. Forward-looking firms can expect to leverage a sizable advantage in warehouse operations by effectively managing all of these large data sets.
Managing big data well – along with industrial automation and smart networks – can result in substantial productivity improvements for autonomous warehouses, supply chains, and logistics companies.
Big data analysis helps businesses to identify subtle or nonintuitive areas for improvement that yield larger than expected performance gains.
Generally speaking, it works by identifying and monitoring identifiers for numerous small details in the logistics chain: from consumer trends to the price of local utilities and fuel to workforce availability.
Adding up many of these small detail identifiers and recording data on them over time can quickly generate large data sets containing lots of useful business planning and management data.
However, managing and analyzing these large data sets is necessary to achieve productivity gains, especially for autonomous warehouses were analyzing data is commonplace in warehouse operations.
What is the Industrial Internet of Things?
The Internet of Things (IoT) refers to a network (or networks) of things or objects that can be linked together via the internet. The term conceptualizes a system of objects (think sensors, lights, speakers, locks) that are all linked by the internet.
When this concept is applied in industrial settings, it is referred to as the Industrial Internet of Things (IIoT).
IIoT networks combine smart technology (devices with data processing chips and internet connectivity built in) with various industrial processes, including autonomous warehouse operations.
How Do Autonomous Warehouses Acquire Data?
Autonomous warehouse operations that deploy IIoT systems quickly generate large amounts of data on their business. RFID data shows where individual inventory items are physically located, sensor data records environmental factors in an autonomous warehouse facility like humidity and temperature, and clocking-in systems keep track of the robot workforce.
In autonomous warehouses, sensors and scanners deployed in key locations like forklifts, conveyor belts, and racking systems record and automatically transmit large amounts of useful operational data.
Connected to a live IIoT network, these help warehouses operate autonomous monitoring and management of through speeds, stock levels, and storage capacity.
Installations for individual autonomous warehouses are unique to the requirements of that warehouse. For example, a cold food or pharmaceutical storage facility will monitor temperature and humidity levels more closely, while distribution centers may invest more in autonomous vehicles, mobile robots and data storage.
Forklift sensors include impact sensors to monitor collisions (a reliable indicator of too much pressure on some aspect of the operation) and load sensors that can detect how far a forklift travels with and without loads throughout the shift (which indicates the effectiveness of route planning).
The key to acquiring big data sets in autonomous warehouses – and any operation – is making the data acquisition process as passive as possible. This is where IIoT concepts come into play. Wireless connections and automated data acquisition throughout the day enable autonomous warehouses to grow big data sets without creating extra responsibilities for staff.
How to Manage and Analyze Big Data
Putting that big data to work requires investment in skilled personnel, either through new recruitment or retraining programs for workers displaced by automation.
Data analysts closely study big data sets captured by autonomous warehouse operations to find ways to improve efficiency and productivity. For example, revising maintenance schedules to avoid equipment failure before it happens or optimizing air conditioning and refrigeration units to maintain correct temperatures with as little energy as possible.
Large autonomous warehouse facilities, complex operations, and international companies operating worldwide create data sets so vast that managing and analyzing them manually is next to impossible.
In these cases, artificial intelligence (AI) technologies like machine learning (ML) and deep neural networks take on the work of big data analytics. Computers can parse digital information much faster and more accurately than humans can, and with enough processing power, they can do it instantly.
Advanced Data Management in Autonomous Warehouses
A key benefit to connecting autonomous warehouse functions up in an IIoT network with algorithmic data management in place is that it lays the groundwork for more automation.
Increasingly, many functions in modern warehouses are being automated with robots. From robots with extremely limited degrees of freedom like conveyor belts and thermostats to fully mobile autonomous forklifts and pickers, industrial automation is transforming warehouse operations.
Big data that has been captured passively and analyzed automatically with AI can inform robot control programs, creating a virtuous cycle of optimization in the autonomous warehouse.
Large data sets are acquired through day-to-day warehouse operations and then transmitted to a central computer (or cloud service). There, algorithms run to find ways to optimize processes in the warehouse. New instructions can then automatically be created for autonomous functions in the warehouse operations to realize the potential optimization.
All of this can happen almost instantly without a human driver, enabling autonomous warehouses to respond to changing circumstances immediately, dynamically reacting to ensure operations are as productive as possible at all times. As well as this, advanced data management of big data can help produce predictive analytics, informing businesses of potential problems before they even occur.
References and Further Reading
Pilkington, B. (2022). How is the Digital Transition Benefiting the Planet? [Online] AZO Materials. Available at: https://www.azom.com/article.aspx?ArticleID=21652
Viswanathan, N. (2018). Using Prescriptive Analytics to Decrease Supply Chain Disruptions. [Online] Supply Chain Management Review. Available at: https://www.scmr.com/article/are_you_using_prescriptive_analytics_to_decrease_supply_chain_disruptions_y
Disclaimer: The views expressed here are those of the author expressed in their private capacity and do not necessarily represent the views of AZoM.com Limited T/A AZoNetwork the owner and operator of this website. This disclaimer forms part of the Terms and conditions of use of this website.