Agronomy is a branch of agriculture that deals with crop production and soil management. Typically, agronomists are involved in the large-scale production of plants, focusing on several factors, such as yield, phytopathogens, plant disease, cultivation, weed management, soils, and the impact of climate change on plant growth. At present, digital technology has enabled the acceleration of agricultural practices, and this approach is known as computational agronomy.
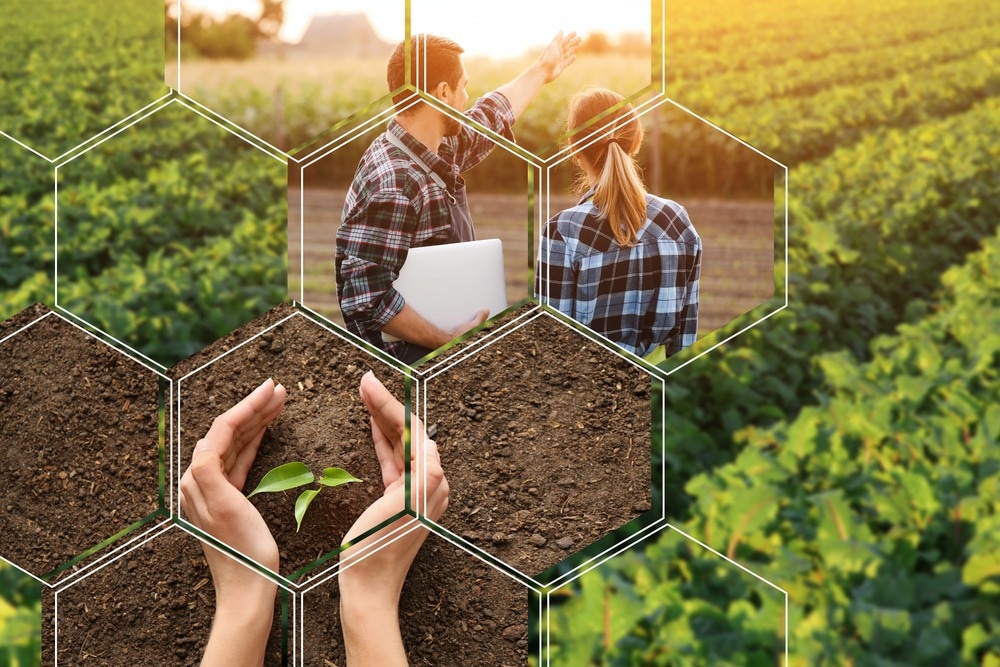
Image Credit: Pixel-Shot/Shutterstock.com
Challenges of Modern Agriculture
Necessary changes in agricultural practices are required to keep up with the ever-increasing demands of rising global populations.
For instance, it is essential to select crops based on available information and develop an optimal method to grow them. Modern agriculture has focussed on cultivating high-yielding crops whose growing parameters have been standardized.
Intensive cultivation of only a few varieties of high-yielding crops makes the agriculture system vulnerable to pests, diseases, and climate change. This process leads to soil nutrient depletion and reduction in soil microbe diversity, which ultimately affects future plant growth. Therefore, in the long term, these modern agricultural practices could lead to the cultivation of less nutritious food products.
How Could Computational Agronomy Help?
Scientists envisioned how technology could be used to uncover the genetic diversity of edible plant species that exist globally. This process will help agronomists to identify specific plant species or crop varieties that can be resilient and grow freely under climate change pressure.
If technologies assist agronomists to understand the optimal growing parameters of each and every plant and how they interact with the environment, a significant reduction in the use of water, chemical fertilizers, pesticides, and weed killer could be achieved.
The first phase of agriculture digitalization involves automating the execution of human decisions based on algorithms and sensors. Currently, computational agronomy is still in its infancy; however, many companies are coming forward to advance the same.
The Moonshot Project of Alphabet’s X
Although many farmers are already using sensors and GPS, this information does not fully represent agricultural complexities. Alphabet’s X lab has currently focussed on the “moonshot” project that combines artificial intelligence and computer resources for farming practices.
Mineral is a new sibling of Google that aims to use the latest technologies to feed the growing population. Mineral believes that to feed the global population, farmers have to adopt the latest technologies or “computational agronomy”.
Researchers have developed and tested several software and hardware prototypes based on artificial intelligence, robotics, simulation, sensors, and many more relevant components. The main focus of these applications is to monitor and project information on the nutritional need of a plant. In addition, the latest technologies could be used to uncover the genetic and environmental factors that enhance crop yields.
Mineral has recently developed prototype plant buggies that can slowly drive across the farmland. These buggies use cameras to collect data about the plant, including its height, leaf area, and fruit size, which can be combined with other data sets, such as soil and weather. Machine learning has been used to understand how plants grow and interact with their environment.
The above-mentioned solar-powered plant buggy was tested against crops, such as berries, oats, oilseeds, lettuce, melons, and barley, from germination to harvest. This system can detect specific requirements of individual plants, such that farmers can now use this technique and spray pesticides on specific plants instead of the entire field.
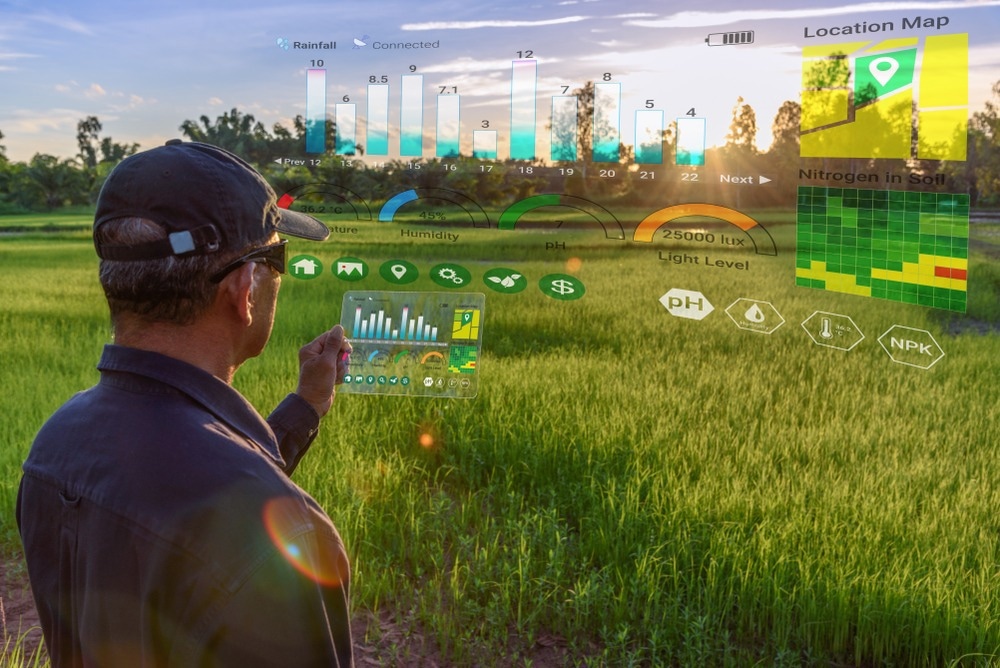
Image Credit: William Potter/Shutterstock.com
Computational Agronomy Improves the Taste of Edible Plants
Researchers at MIT’s Media Lab have combined botany, chemistry, and machine-learning algorithms to create basil plants with enhanced flavor. Notably, no genetic modifications were involved in this process. Here, computer algorithms were used to determine the optimal growing conditions of basil plants to maximize the synthesis of flavoring molecules, such as volatile compounds.
In addition to flavor enhancement, this technique can detect plants that can adapt and grow under different conditions. Herein, scientists focussed on designing an open-source technology by combining data acquisition, sensing, and machine learning, and applied it in agricultural research.
Application of computational agronomy in basil plants to enhance its flavors revealed that exposing the plant to light for 24 hours a day generated maximum flavor. Conventional agricultural techniques would have never been able to decipher this insight.
To validate the computational agronomy data, experiments were conducted in a warehouse at the MIT-Bates Laboratory, Massachusetts. The plants (OpenAg) were grown in shipping containers such that the environmental conditions, including humidity, temperature, and light could be controlled.
Once the plants were fully grown, scientists evaluated the taste of the basil leaves based on the levels of volatile compounds found in them. The volatile compounds were detected and quantified using gas chromatography and mass spectrometry techniques. This type of agriculture is also known as vertical or urban farming.
Researchers have planned to make OpenAg hardware, software, and data freely available so that their technology can benefit other researchers and farmers.
References and Future Reading
Britannica, T. Editors of Encyclopaedia (2021). Agronomy. Encyclopedia Britannica. Available at: https://www.britannica.com/science/agronomy
Stone, L. (2020) Alphabet’s X launches ‘computational agriculture’ business Mineral. Available at: https://aibusiness.com/verticals/alphabet-s-x-launches-computational-agriculture-business-mineral
Johnson, J.A. et al. (2019) Flavor-cyber-agriculture: Optimization of plant metabolites in an open-source control environment through surrogate modeling. PLoS ONE, 14(4). https://journals.plos.org/plosone/article?id=10.1371/journal.pone.0213918
Grant, E. (2019) Entering the era of computational agriculture. Available at: https://blog.x.company/entering-the-era-of-computational-agriculture-9f8417f21be0
do Prado, H. A., Barreto Luiz, A., and Filho, H. (Eds.). (2011). Computational Methods for Agricultural Research: Advances and Applications. IGI Global. https://doi.org/10.4018/978-1-61692-871-1
Disclaimer: The views expressed here are those of the author expressed in their private capacity and do not necessarily represent the views of AZoM.com Limited T/A AZoNetwork the owner and operator of this website. This disclaimer forms part of the Terms and conditions of use of this website.