The scientific environment, including many areas of health, is being quickly transformed by machine learning (ML) and artificial intelligence (AI). In this article, we explore how AI is being used to help automate the imaging pipeline.
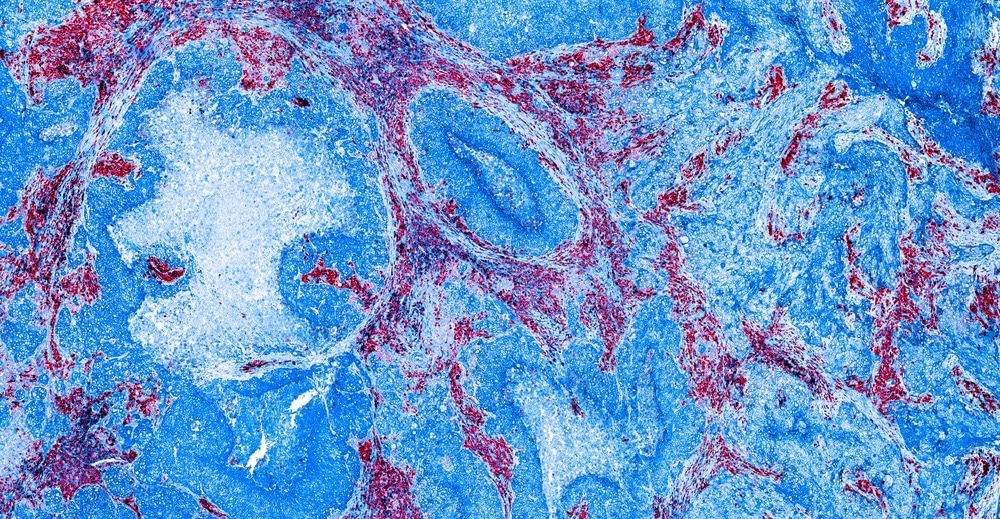
Image Credit: Carl Dupont/Shutterstock.com
While ML is within the larger process of AI in which machines or tools learn from data to generate classifications or predictions, either with or without human supervision, AI refers to the invention of machines or tools that emulate human thought and behavior. High-performance computers have recently emerged, speeding up the advancement in several disciplines.
Digitized fields in medicine, like imaging, are well-suited to becoming early users of ML and AI. The imaging pipeline, which includes acquisition, reconstruction, interpretation, reporting, and the sharing of results, is carried out digitally, making it possible to acquire such data for AI and ML efficiently. In particular, radiologists seem to be more likely to explore and adopt new technologies early on, as cancer imaging is a crucial stage of the process.
Tasks related to the full diagnostic and treatment identification process can be repetitive and exhausting, where readers must sift through extensive studies to identify abnormalities.
There are already a variety of commercially available devices in the field of cancer imaging that are designed to increase productivity, lower error rates, and improve diagnostic accuracy.
However, many technological advancements are being made in isolation and may have trouble finding widespread clinical use.
The inability of clinicians, radiologists, scientists, and other specialists to collaborate to comprehend the clinical and data science landscape, and to identify the requirements, risks, and other challenges for the development, validation and adoption of such tools may have hampered these efforts. Promoting breakthroughs and advancements necessitates the collective cultivation of multidisciplinary ecosystems, including commercial partners, as necessary.
Radiomics
The computer-based examination of specific areas of medical pictures is known as radiomics. The images can be two-dimensional, like 2D X-ray, three-dimensional, like CT, or four-dimensional, like ultrasound. The basic objective of radiomics is to use algorithms that can recognize patterns in images, typically beyond what the human eye can see, and to take advantage of those patterns to provide predictions and support clinical decision-making.
To facilitate pipeline data analysis, several radiomics processing tools are being developed. For example, the Quantitative Image Feature Pipeline, developed at Stanford, contains an expansive library of modeling algorithms that are capable of comprehensively managing the imaging and predictive pipeline, including cloud-based software. It also contains algorithms for generating quantitative image features, which can be used to characterize the imaging phenotype.
When developing ML algorithms and models for cancer imaging, patient images are pre-processed and modified as inputs. Whether they pertain to radiologists-defined features or computationally derived radiomics features, such pre-processing techniques are utilized. A training dataset is typically used to construct ML algorithms, a validation dataset to modify them, and a test dataset to evaluate their performance. The models are then distributed to other entities and trained using local data at each site.
AI in Risk Assessment
Resources are primarily focused on patients who are most at risk to make the best use of cancer imaging tools. For example, many US state governments mandate the evaluation of breast density to determine breast cancer risk. The classification of breast density by a deep learning system demonstrated great accuracy, and such systems will allow consistent density notification to patients during breast cancer screening.
Screening and Detection Using AI
AI research has been quite active in the field of cancer screening. AI algorithms have been tried on illnesses, including lung cancer and breast cancer, that have active screening systems. Studies on breast cancer have shown that AI algorithms can perform as well as expert readers and serve as a second reader for mammographic reviews.
AI for Image Classification
ML systems offer strategies for improving the classification of cancer-related images. The causes and prognoses of malignant brain tumors vary; however, tissue sampling is invasive and might not give a clear picture of the disease because of its variety. According to studies, AI has the capacity to recognize and categorize major intracranial cancers, as well as distinguish them from healthy cells.
Correlation and Treatment
Machine learning and radiomics have been used to anticipate how a disease will respond to treatment and how it will turn out. It is crucial for education, quality improvement, and patient care that radiological data and information from pathology reports are matched. It is possible to mine text-based radiology and pathology reports using natural language processing techniques to locate important findings to cohort-specific populations for additional investigative inspection.
Segmentation and Image Optimization
In many radiomics investigations that involve AI or ML, the outlining of illness, or segmentation, is crucial to extract quantitative tumor measurements, such as tumor sizes, as well as to generate tumor contours for radiotherapy planning.
Imaging optimization is one of the expanding uses of AI and ML in imaging, not just for cancer imaging. For instance, an oncological body inspection in an MRI can take 30 to 60 minutes. It is becoming more common to use AI and ML approaches to speed up image acquisition or reconstruction.
Future Outlook
Most radiologists are supportive of the wide range of clinical applications that machine learning, in particular, cancer imaging, is seeing in the field of AI. The availability of imaging data frequently restricts the creation of new machine learning (ML) techniques; however, there is potential for creating and utilizing real-world, carefully curated imaging data in biobanks and open access repositories to get around these restrictions.
Using open-source tools for algorithm development may improve transparency and cross-center cooperation. AI can increase productivity by precisely identifying infections in X-ray and CT images, allowing for later quantification. Additionally, computer-aided platforms support radiologists in their clinical judgements, such as disease diagnosis, monitoring, and prognosis.
References and Further Reading
Shi F, Wang J, Shi J, Wu Z, Wang Q, Tang Z, He K, Shi Y, Shen D. (2021) Review of Artificial Intelligence Techniques in Imaging Data Acquisition, Segmentation, and Diagnosis for COVID-19. IEEE Rev Biomed Eng. 14, pp. 4-15. https://pubmed.ncbi.nlm.nih.gov/32305937/
Koh, DM., Papanikolaou, N., Bick, U. et al. (2022) Artificial intelligence and machine learning in cancer imaging. Commun Med, 2, p. 133 . https://www.nature.com/articles/s43856-022-00199-0
Thomas, Marie Franziska, Florian Kofler, Lioba Grundl, Tom Finck, Hongwei Li, Claus Zimmer, Björn Menze, and Benedikt Wiestler. (2022) Improving automated glioma segmentation in routine clinical use through artificial intelligence-based replacement of missing sequences with synthetic magnetic resonance imaging scans. Investigative Radiology, 57(3) , pp. 187-193. https://pubmed.ncbi.nlm.nih.gov/34652289/
Caliva, Francesco, Nikan K. Namiri, Maureen Dubreuil, Valentina Pedoia, Eugene Ozhinsky, and Sharmila Majumdar. (2022) Studying osteoarthritis with artificial intelligence applied to magnetic resonance imaging. Nature Reviews Rheumatology, 18(2), pp. 112-121. https://pubmed.ncbi.nlm.nih.gov/34848883/
Disclaimer: The views expressed here are those of the author expressed in their private capacity and do not necessarily represent the views of AZoM.com Limited T/A AZoNetwork the owner and operator of this website. This disclaimer forms part of the Terms and conditions of use of this website.