In marine exploration, a new era is dawning as underwater robots, both autonomous and intelligent, redefine our understanding of the ocean's mysteries.
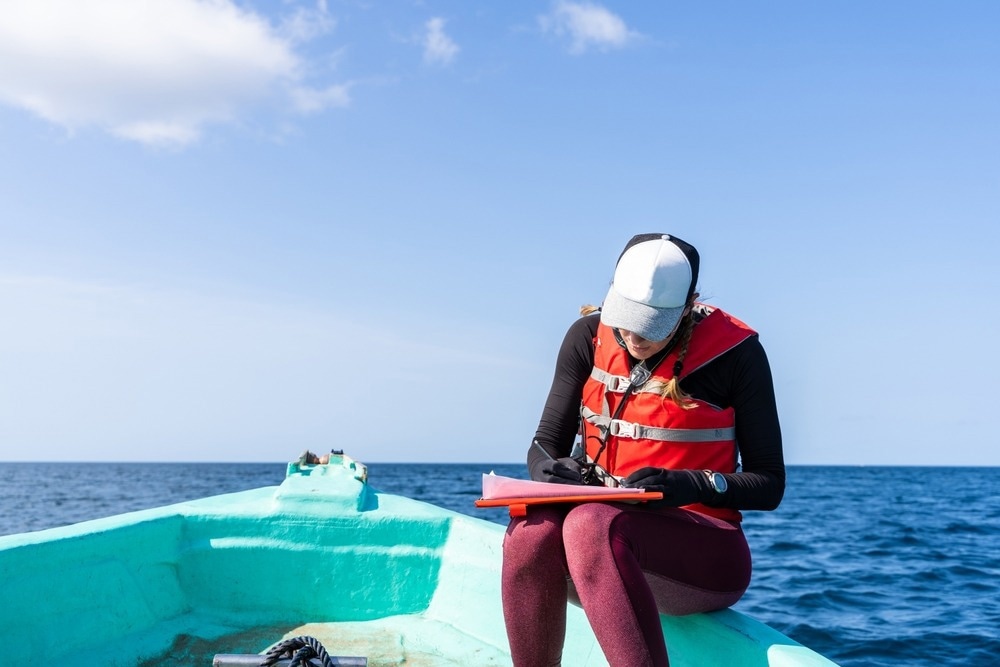
Image Credit: Riderfoot/Shutterstock.com
Underwater Robots Advancing Marine Discoveries
Underwater robots typically refer to autonomous underwater vehicles (AUVs) or remotely operated vehicles (ROVs). Both AUVs and ROVs can be equipped with sensors, cameras, and instruments that enable the exploration and sampling of marine systems. The findings provided by underwater robots have revolutionized our understanding of the ocean by providing unprecedented access to new depths and habitats.
From seafloor mapping to environmental monitoring and the study of marine habitats, underwater robots have provided a wealth of information since their invention in the 1950s. The first AUV was the "Self-Propelled Underwater Research Vehicle" which studied submarine wakes and acoustic transmission underwater. Since then, underwater robots have developed into many forms and fulfill diverse functions thanks to their ‘intelligent’ capacities.
The use of AUVs has been particularly significant in marine research over recent decades. AUVs typically function as profiling floats, buoyancy-driven gliders, or propeller vehicles. The most extensive autonomous ocean network program is the Argo, which has deployed over 3,500 floats around the world.
However, AUVs have been taken a step further with not only autonomous underwater robots but autonomous ships as well. The Mayflower Autonomous Research Ship is a vessel made in the UK that can collect and transmit data from around the world without human operation. Technological innovations have made it possible to combine autonomous robots into autonomous ships that can patrol the oceans and collect data with little human management.
Robots in Marine Research and Conservation
A recent model of an underwater robot, OceanOne, was developed by Stanford scientists and consists of a humanoid AUV designed to mimic human function for sampling coral reefs and benthic systems. With the use of its wrists and fingers, OceanOne can perform lengthy human tasks at depths typically unreachable by humans. Although it is primarily used for sampling ecosystems, the data it collects can directly inform scientists about the susceptibility of the system to human-driven changes.
With the rise of AI technologies, underwater robot projects now seek to harness intelligent underwater robots. Typically, systems will undergo reinforcement learning, so the robots learn to perform actions depending on context. In turn, many studies have been published on how reinforcement learning allows AUVs to navigate underwater (Carlucho et al. 2018), dock and depart autonomously (see Anderlini et al. 2019), locate and track objects in marine systems to perform complex tasks (see Masmitja et al. 2023).
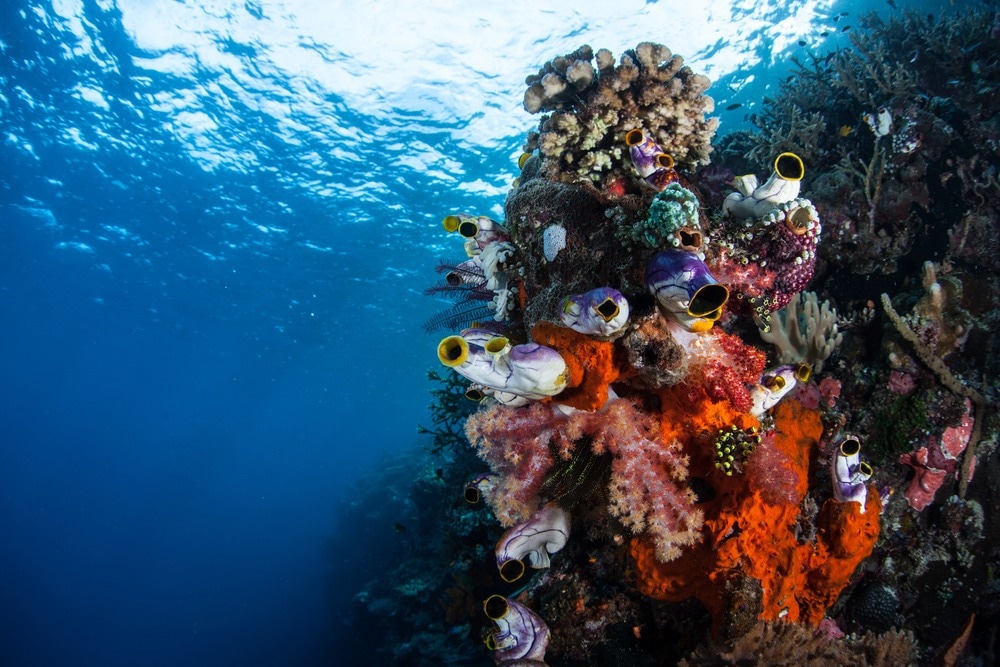
Image Credit: Ethan Daniels/Shutterstock.com
Reinforcement learning is at the base of what underwater robots can achieve. It allows scientists to understand CO2 capture, plankton movements, and coral reef health. With such a broad range of applications, underwater robots are at the forefront of technological innovations in marine research.
Networks of AUVs such as Argo are already deployed worldwide, but individual robots such as OceanOne that operate a range of missions such as planting corals, taking delicate samples, and exploring cave systems are taking marine research to unprecedented levels.
Future Applications and Emerging Limitations of Underwater Robots
With the rising popularity of ROVs and AUVs, underwater robots have been applied to fulfill a range of other functions beyond research in the marine environment. For instance, the BIOSwimmer robot from Boston Engineering has been used to monitor harbors, detect smuggled goods in ships, and can even be used for military operations. Sustainable energy systems have also been integrated into AUVs, with the autonomous Wave Glider from Liquid Robotics using wave energy and solar panels to navigate the oceans and demonstrating what future systems AUVs could incorporate.
Another example of autonomous technology is the Velella Beta, an offshore floating fish farm using the SUBflex system to pivot with the current. This fish farm consists of a 132-cubic-meter pod connected to a vessel floating offshore with the potential to transform modern aquacultural structure. Specifically, the ocean cage system holding fish ensures a safer aquacultural production for humans and local ecosystems and displayed a 98% survival rate of fish during testing.
Robots and autonomous technologies are being increasingly utilized in ocean studies. However, the costs of development and operation limit the high production capacity of complex ROVs. Models such as OceanOne or the BIOSwimmer require extensive investments, thus making ROVs difficult to scale to large operations.
In contrast, AUVs are becoming increasingly less expensive despite their technology becoming increasingly complex. Environmental sensors and buoyancy devices can relay information to satellites rapidly and effectively. Costs of AUV operations are decreasing constantly, while technology now allows real-time data gathering on broad scales.
Underwater robots provide a wealth of information on ocean functioning and are pivotal tools used to better understand valuable ecosystems. With a world experiencing rapid environmental change, underwater robots and particularly AUVs will provide critical information on climatic events and changing ecosystems that can inform broader policies. As a result, underwater robots will likely play an increasingly important role in marine research in the coming decades.
References and Further Reading
Anderlini, E., et al. (2019). Docking control of an autonomous Underwater vehicle using reinforcement learning. Applied Sciences, 9(17), p. 3456. doi.org/10.3390/app9173456
Carlucho, I., et al. (2018). Adaptive low-level control of autonomous underwater vehicles using deep reinforcement learning. Robotics and Autonomous Systems, 107, pp. 71–86. doi.org/10.1016/j.robot.2018.05.016
Cong, Y., et al. (2021). Underwater robot sensing technology: A survey. Fundamental Research, 1(3), pp. 337–345. doi.org/10.1016/j.fmre.2021.03.002
Masmitja, I., et al. (2023). Dynamic robotic tracking of underwater targets using reinforcement learning. Science Robotics, 8(80). doi.org/10.1126/scirobotics.ade7811
Schofield, O., et al. (2018) The robot Ocean Network. American Scientist. Available at: https://www.americanscientist.org/article/the-robot-ocean-network
Zhang, Y., et al. (2021). A system of coordinated autonomous robots for Lagrangian studies of microbes in the oceanic deep chlorophyll maximum. Science Robotics, 6(50). doi.org/10.1126/scirobotics.abb913
Disclaimer: The views expressed here are those of the author expressed in their private capacity and do not necessarily represent the views of AZoM.com Limited T/A AZoNetwork the owner and operator of this website. This disclaimer forms part of the Terms and conditions of use of this website.