In this article, we explore the use of artificial intelligence in Raman spectroscopy.
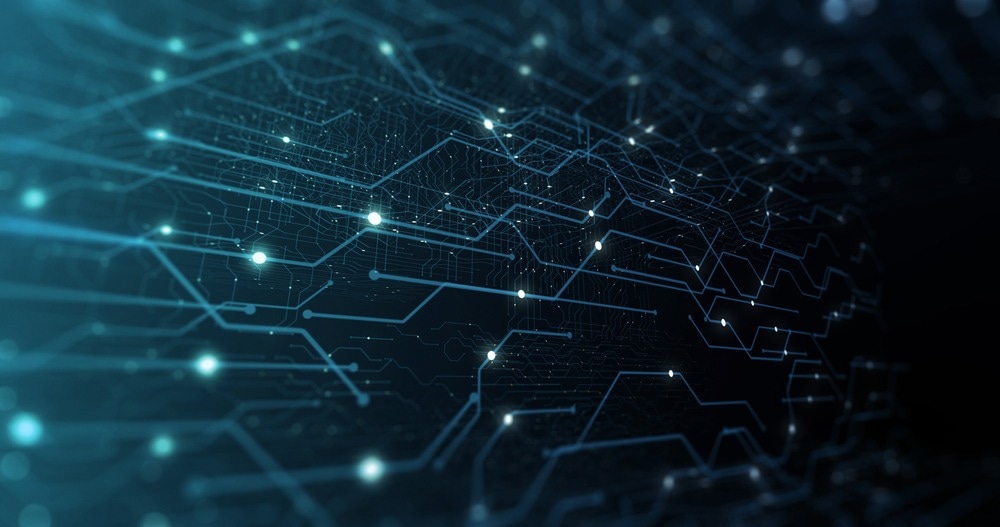
Image Credit: Quardia/Shutterstock.com
One of the main applications of artificial intelligence (AI) in Raman spectroscopy has been for image recognition for medical diagnosis.1 One of the key advantages of Raman spectroscopy is that it is a label-free technique and can be integrated into point-of-care devices for advanced bedside diagnostics for patients as well as live, non-invasive monitoring.
Raman spectroscopy works for chemical identification by measuring the vibrational frequencies of the bonds in the target molecule. As the frequencies of the bonds are determined by both the particular atoms involved in the bond and the local environment, analysis of the various spectral line positions and intensities can be used to identify a target chemical.
Combining Raman spectroscopy with imaging applications has proved a useful tool for tumor identification and profiling.2 One of the key advantages of Raman spectroscopy and microscopy is that the tumor analysis does not require a biopsy sample to be taken and evaluated in the histopathology laboratory.
While histopathology is still considered the ‘gold standard’ in medical diagnostics, for live situations such as surgery or where very quick diagnostics are needed, Raman spectroscopy offers a powerful alternative, as well as often removing the need for invasive biopsy sampling.3
One of the complexities associated with using Raman spectroscopy for tumor identification and evaluation of malignancy is that the spectral information can often be very rich, with many spectral features overlapping from different chemical species. In addition, where imaging modalities have been used, there can be large volumes of data to analyze.2
Artificial intelligence approaches can help unlock the rapid diagnostic power of spectroscopies such as Raman spectroscopy by identifying frequencies associated with chemical species in malignant tumors.4 Artificial intelligence can help with the rapid analysis of the image and spectroscopic data for diagnostics.
Tumor Identification
The key advantage of methods like laser Raman spectroscopy over traditional histopathology approaches is the speed with which a diagnosis can be made. A recent study used laser Raman spectroscopy in combination with two machine learning algorithms to provide real-time data analysis to identify whether a patient was suffering from breast cancer.4
Breast cancer is the most common cancer for women worldwide and nearly one in five patients will undergo re-excision surgery following diagnosis. Early intervention is key to improving patient outcomes.
One of the advantages of using artificial intelligence and neural networks for the spectroscopic analysis is that the team demonstrated that there are Raman markers not just for the presence of a tumor itself, but more specifically, the DNA/RNA components, lipids and protein components. Overall, losses in the DNA/RNA signal were one of the best indicators of tumor presence, with the neural networks able to rapidly generate several models for classification.
Portable Raman Measurements
Raman spectroscopies compatibility with point-of-care devices brings new possibilities in where and how patients can be screened. Some recent work has been making use of a robotic Raman device and convolutional neural networks (CNNs) to develop an instrument for community-level screening of endometrial cancer.5
While there were some challenges in the availability of certain types of data for training the model for particular high-grade tumor types, the team was able to find a number of spectral features associated with the presence of endometrial cancers and obtain decent accuracy in diagnosis.5
In particular, as the device was designed to be portable and the noise-content of the images would be larger than small handheld devices, the team needed to demonstrate that the CNNs could successfully still identify tumors in nosier and non-ideal datasets. This is an important development for performing Raman measurements on tumor regions that are difficult to access, where image contrast may not be as good.
Other Applications
Another application of Raman spectroscopy in combination with artificial intelligence has been to investigate the profile of compounds in urine samples as an early diagnostic for prostate cancer. 6
Current analysis methods tend to flag a large number of false positives leading to unnecessary biopsies. However, by using artificial intelligence to help identify biomarkers that indicate the presence of a tumor, researchers could help provide a non-invasive, more accurate alternative.
Urine is chemically complex in terms of the number of species, making spectral analysis somewhat challenging. By using a deep learning algorithm that could both identify and correlate the presence of particular biomarkers with each other, the team could then create a tumor diagnostic model, focusing on the presence of amino acid and tumor metabolites. The team suggested the strongest correlation with the presence of tumors was with the amino acid concentrations and that this was one of the most valuable types of analysis to include in diagnostic models.
Improvement in fiber optic bundles for obtaining better quality images and the opportunities to train machine learning algorithms on larger datasets are important steps in obtaining higher quality images and improved accuracy for automated diagnosis.
From early results, though, the ability to quickly apply trained models for data analysis seems like a very promising route to improving diagnostic speeds and helping patients get the early interventions they need.
References and Further Reading
Qi, Y., Liu, Y., & Luo, J. (2023). Recent application of Raman spectroscopy in tumor diagnosis : from conventional methods to artificial intelligence fusion. PhotoniX. https://doi.org/10.1186/s43074-023-00098-0
Lazaro-pacheco, D., Shaaban, A. M., & Rehman, S. (2020). Raman spectroscopy of breast cancer. Applied Spectroscopy Reviews, 55(6), 439–475. https://doi.org/10.1080/05704928.2019.1601105#
Rubin, G., Vedsted, P., & Emery, J. (2011). Improving cancer outcomes: Better access to diagnostics in primary care could be critical. British Journal of General Practice, 61(586), 317–318. https://doi.org/10.3399/bjgp11X572283
Kothari, R., Jones, V., Mena, D., Reyes, V. B., Shon, Y., Smith, J. P., Schmolze, D., Cha, P. D., Lai, L., Fong, Y., & Lombardi, M. C. S. (2021). Raman spectroscopy and artificial intelligence to predict the Bayesian probability of breast cancer. Scientific Reports, 1–17. https://doi.org/10.1038/s41598-021-85758-6
Nambudiri, M. N. K., Rajanbabu, A., Nair, I. R., Anandita, Nair, S. V., Koyakutty, M., & Madathil, G. C. (2022). Development of a portable Raman device with artificial intelligence method for the detection and staging of endometrial cancer. Translational Biophotonics, 5, e202200014. https://doi.org/10.1002/tbio.202200014
Chen, S., Zhang, H., Yang, X., Shao, X., Li, T., Chen, N., Chen, Z., Xue, W., Pan, J., & Liu, S. (2021). Raman Spectroscopy Reveals Abnormal Changes in the Urine Composition of Prostate Cancer : An Application of an Intelligent Diagnostic Model with a Deep Learning Algorithm. Advanced Intelligent Systems, 3, 20200090. https://doi.org/10.1002/aisy.202000090
Disclaimer: The views expressed here are those of the author expressed in their private capacity and do not necessarily represent the views of AZoM.com Limited T/A AZoNetwork the owner and operator of this website. This disclaimer forms part of the Terms and conditions of use of this website.