Climate change is significantly impacting crop yields, undermining food security and economic stability and exacerbating the negative environmental impact of the agricultural industry, which further feeds climate change.
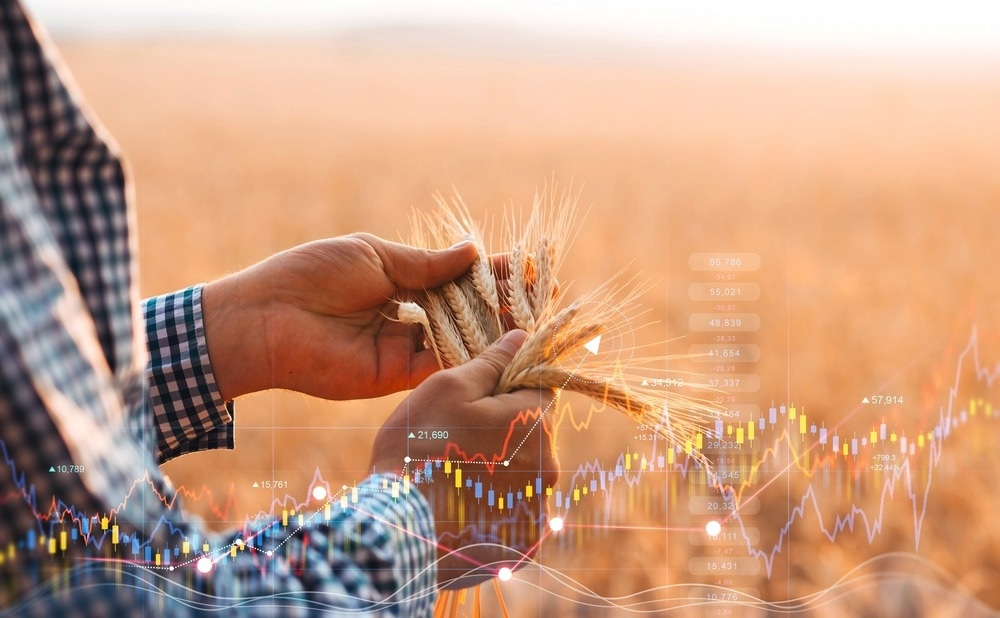
Image Credit: Miha Creative/Shutterstock.com
Artificial intelligence (AI) has been developed so that it can help farmers predict crop yield, mitigating threats to food security and economic stability and helping to make farming processes more sustainable.
How Does Climate Change Affect Crop Yields?
Climate change affects crop yields in numerous ways. Rising global temperatures can reduce crop yields, hinder crop development and cause crop failure. Rice, wheat, and maize are particularly vulnerable to rising temperatures.
Traditional growing seasons are also being altered by climate change, which can make crops more vulnerable to diseases and pests due to altered growing seasons. Changes in rain patterns can lead to crop failure due to drought and excessive rain (which can cause root rot and nutrient leaching).
Why Is It Important to Predict Crop Yields?
By predicting crop yields, some of the negative effects of climate change on crops can be mitigated. Food security can be increased by using predictions to understand how much food may be produced in a specific region so that shortages can be planned to ensure people have access to enough food.
Predicting crop yields also helps to bolster economic stability. In many parts of the world, agriculture is a significant part of the economy. By predicting crop yields, farmers are able to make more accurate financial plans, which helps to stabilize food prices.
The environmental impact of agriculture can also be lessened by predicting crop yields. If a farmer knows when they are likely to have a low yield, they can choose not to plant as much and therefore waste less resources (such as water) nurturing crops that are destined to fail.
Using AI to Predict Crop Yields
In recent years, AI has emerged as a promising tool for predicting crop yields. It is able to deliver highly accurate, data-driven approaches to agricultural forecasting.
AI algorithms are being leveraged to analyze large datasets relating to numerous factors that impact crop yield, such as soil quality, temperature, historical crop yields, and more. These algorithms can accurately identify patterns and relationships to establish reliable predictive models that help farmers understand the likely outcomes of a particular crop yield.
AI is also being used to process and analyze data gathered from satellites along with remote sensing data to help monitor crop growth and health, as well as environmental conditions that impact this. This technology enables the early detection of problems that could impact the overall crop yield.
Disease and pest detection has also been enhanced with the help of AI. Farmers can better identify diseases and pests from images taken on smartphones or by drones. The early detection of pests and diseases gives farmers ample time to minimize the damage they cause to their crops.
Estimating Rice Yields With New AI Technology
Rice is one of the most widely eaten staple foods across the globe, accounting for roughly 20% of the world’s daily calorie intake. It is the primary source of nutrition for billions of people. Furthermore, as the world’s population continues to grow, rice will be relied on to feed even more mouths. Therefore, ensuring an adequate supply of rice is vital for future global food security.
To explore how AI could help improve food security, with a focus on rice crops, researchers from Japan combined digital photos taken at the harvest time of the rice crop along with convolutional neural networks (CNNs) (a type of AI) to estimate rice yield.
The researchers’ CNN model was developed to approximate the grain yield for each individual photo it was shown. A visual-occlusion technique was used to understand how different regions within the rice canopy images contributed to the overall additive effect. The process involved masking parts of the images and looking at how the model responded by altering its yield estimation. The insights obtained from this process allowed the scientists to understand how the CNN model interpreted various features of the images, which contributed to its accuracy in determining yield-contributing elements.
The model performed well, successfully explaining between 68%-69% of yield variation. The study results identified the importance of clusters of flowers, known as panicles, in yield estimation via occlusion-based visualization.
Overall, the model was reliable and had good accuracy. The study highlights the importance of CNN-based models for monitoring rice yields. This kind of technology could be leveraged on a large scale to monitor rice production at a regional level, thus supporting food security at a regional level.
Conclusions
This AI model for predicting rice yield is one of many exciting ways that AI is being leveraged to predict crop yields. The widespread adoption of these techniques will be crucial in enhancing food security and supporting economic stability. In the coming years, we will likely see further developments in this field.
Resources and Further Reading
Morales, A. & Villalobos, F.J. (2023). Using machine learning for crop yield prediction in the past or the future. Frontiers in Plant Science, 14. doi.org/10.3389/fpls.2023.1128388.
Tanaka, Y. et al., (2023). Deep learning enables instant and versatile estimation of rice yield using ground-based RGB images. Plant Phenomics, 5. doi.org/10.34133/plantphenomics.0073.
van Klompenburg, T., et al. (2020). Crop yield prediction using Machine Learning: A Systematic Literature Review. Computers and Electronics in Agriculture, 177, p. 105709. doi.org/10.1016/j.compag.2020.105709.
Disclaimer: The views expressed here are those of the author expressed in their private capacity and do not necessarily represent the views of AZoM.com Limited T/A AZoNetwork the owner and operator of this website. This disclaimer forms part of the Terms and conditions of use of this website.