By Ankit SinghReviewed by Susha Cheriyedath, M.Sc.Jun 23 2024
An aneurysm refers to an abnormal bulge that develops on the wall of blood vessels and can become potentially fatal if not treated on time. Current diagnostic tools such as magnetic resonance imaging (MRI) and computer tomography (CT) can effectively diagnose cases of aneurysms.
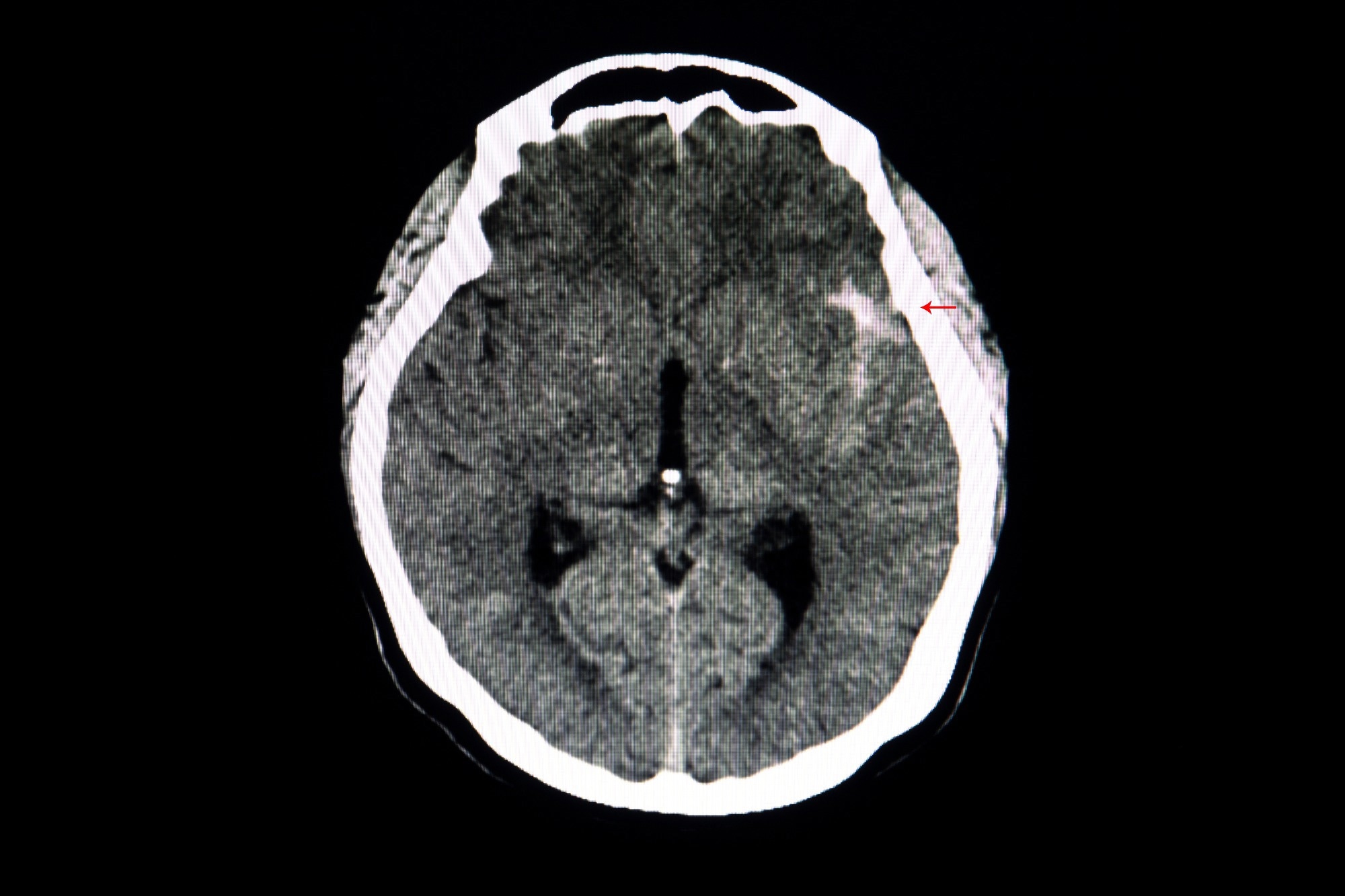
Image Credit: Tomatheart/Shutterstock.com
Yet, these methods can be time-consuming and complex, requiring experienced and highly skilled healthcare professionals. Deep learning, a class of artificial intelligence (AI), has emerged as a game-changer by automating the diagnosis process, leading to improved accuracy and efficiency.
Evolution of Deep Learning Technology
Deep learning originated in the mid-20th century as artificial neural networks and progressed from basic structures to back-propagation algorithms that emerged in the 1980s and 1990s. The actual rise occurred in the early 2000s with the arrival of GPUs and higher computational capabilities that made it possible to train deeper networks.1
Today, the onset of convolutional neural networks (CNNs) has proved to be crucial in the detection of aneurysms. These models excel at classifying pathological images in medical scans with high probabilities, surpassing traditional methods and transforming medical diagnostics.1
Principle of Deep Learning Algorithms
The main pillars of deep learning algorithms are CNNs, which are composed of interconnected nodes or neurons. These neurons contain multiple layers that are especially helpful in feature extraction and pattern identification processes. The key CNN layers are as follows.
- Convolutional Layers: These layers apply convolution operations to the input images using filters to detect local patterns such as edges, textures, and shapes. This process helps in capturing spatial hierarchies and features relevant to the required task.2
- Pooling Layers: Pooling layers reduce the spatial dimensions of the feature maps generated by convolutional layers. It decreases the computational load and overfitting. Common pooling operations include max pooling and average pooling.2
- Fully Connected Layers: These layers connect every neuron in one layer to every neuron in the next layer. This network builds complex relationships between the spatial features of the medical images and makes final predictions.2
In the context of medical diagnosis, CNNs can be trained using thousands of images. This process empowers the networks to recognize and identify anomalies such as aneurysms. The training of a deep learning model involves the manipulation of the weights and biases of the neurons through backpropagation. This technique allows the model to minimize the error between its predictions and the actual outcomes. This repetitive process continues until the model reaches the desired level of accuracy.2
Applications of Deep Learning in Aneurysm Diagnostics
Deep learning algorithms have completely transformed the diagnosis and treatment landscape of aneurysms. By leveraging advanced neural network architectures, these technologies provide unprecedented accuracy, efficiency, and support in various stages of medical care. This section explores these diverse applications in detail.
Enhancing Diagnostic Accuracy
Deep learning methods have shown immense promise in improving diagnostic accuracy. A study published in Neuroinformatics demonstrated that a deep learning model trained on thousands of annotated images could match the diagnostic accuracy of experienced radiologists in identifying intracranial aneurysms on CT angiography images.3 Additionally, ensemble learning techniques, which use multiple deep learning models simultaneously, have proven more sensitive and specific in detecting aneurysms than individual models.4
Reducing Diagnostic Time
The introduction of deep learning has dramatically reduced diagnostic time. Traditional methods, reliant on manual inspection of medical images, are time-consuming and prone to human error. In contrast, deep learning algorithms can analyze and process images quickly.
A study published in Cardiovascular Engineering and Technology reported a high-speed, accurate deep-learning model that processed large batches of CT angiography images for aneurysms within minutes, significantly faster than manual review by radiologists. This speed is particularly advantageous in emergencies, where quick diagnosis is crucial.5
Assisting in Preoperative Planning
Deep learning plays a crucial role in preoperative planning for aneurysm treatment. Accurate identification and understanding of aneurysms are vital for deciding on the appropriate surgical or endovascular treatment. Sophisticated algorithms can generate detailed 3D representations of aneurysms, assisting surgeons in preparing for accurate and minimally invasive procedures.
A study published in Neurosurgical Focus developed a deep learning-based system that produced 3D representations of cerebral aneurysms using CT and MRI images, improving visualization and understanding of aneurysm shapes and enriching healthcare workers' education by offering comprehensive anatomical models for analysis.6
Personalized Patient Monitoring
Beyond diagnostics and preoperative planning, deep learning is instrumental in personalized patient monitoring. Continuous monitoring of patients with known aneurysms is essential to detect any changes in size or morphology that might indicate a heightened risk of rupture. Deep learning models can analyze sequential medical images over time to monitor aneurysm progression.
A recent study in SPIE demonstrated the use of recurrent neural networks (RNNs), a type of deep learning model capable of handling sequential data, to monitor the growth of intracranial aneurysms in patients over time. This method enables the detection of patients who might necessitate more frequent monitoring or early intervention, leading to enhanced patient outcomes.7
Recent Breakthroughs
The field of deep learning in aneurysm diagnosis is making rapid progress with continuous research, and advancements focused on addressing current constraints and enhancing clinical uses.
A recent Scientific Reports study introduced an automated workflow for accurate measurement of abdominal aortic aneurysms using semantic segmentation with active learning (AL) and computer-aided design. Utilizing models like UNEt Transformers (UNETR) and 3D U-Net, the workflow significantly improved accuracy and reduced segmentation time for key clinical landmarks.8
For 96 patients, the 3D U-Net model achieved the highest dice similarity coefficient and significantly reduced manual segmentation time, demonstrating the workflow's efficiency and potential to streamline endovascular aneurysm repair procedures.8
Another intriguing development in the field is transfer learning, which entails adjusting pre-existing deep-learning models with specialized medical datasets. This approach reduces the need for large annotated datasets and speeds up the training process. A recent JVSCIT study showed how transfer learning can enhance the performance of aneurysm detection models, especially when there is limited data available.9
Explainable AI (XAI) methods are also gaining traction when it comes to tackling the fundamental "black box" nature of deep learning models, aiming to render the decision-making process of AI models more transparent and understandable. A recent study in World Neurosurgery introduced an XAI framework that provides visual explanations for the predictions made by aneurysm detection models, helping clinicians understand the reasoning behind the AI's decisions and increasing trust in the technology.10
Challenges in Implementing Deep Learning for Aneurysm Diagnostics
The practical use of deep learning for aneurysm diagnostics faces several challenges and must be addressed to ensure effective integration into clinical practice. Understanding these challenges is crucial for advancing and accepting this technology in medicine. One of the main challenges is the need for large, high-quality datasets. Training deep learning models requires a lot of data, and accurately labeling medical images can be difficult and expensive.
Another big challenge is understanding deep learning models. While these models can be very accurate, it can be hard to understand why they make certain predictions. This "black box" nature of deep learning makes it difficult for medical professionals and regulatory bodies to trust the models.
Additionally, integrating deep learning systems into clinical workflows needs thorough validation and standardization. It is important to ensure that these models work well across different patient populations and imaging techniques for them to be widely used. Dealing with issues related to data privacy and security is also crucial due to the sensitive nature of medical information.
Future Prospects and Conclusions
The future of using deep learning to automate the diagnosis of aneurysms looks promising. Current research is working on addressing challenges by creating models that are easier to understand, building large sets of labeled data, and improving how these technologies are used in medical practice. There is also potential for making the models stronger and more adaptable through advancements in federated learning.
In conclusion, deep learning could change the current landscape of aneurysm diagnosis. It can make diagnoses more accurate and quicker, help with surgery planning, and allow for personalized patient monitoring. There are still challenges, but as deep learning technology advances and researchers, doctors, and regulators work together, it could be used more widely and improve patient outcomes. Deep learning is expected to play a bigger role in automating aneurysm diagnosis, which could lead to big changes in medical imaging and patient care.
References and Further Reading
- Kaul, V., Enslin, S., & Gross, S. A. (2020). History of artificial intelligence in medicine. Gastrointestinal Endoscopy, 92(4), 807–812. https://doi.org/10.1016/j.gie.2020.06.040
- Wang, X., Zhao, Y., & Pourpanah, F. (2020). Recent advances in deep learning. International Journal of Machine Learning and Cybernetics, 11(4), 747–750. https://doi.org/10.1007/s13042-020-01096-5
- Di Noto, T. et al. (2022). Towards Automated Brain Aneurysm Detection in TOF-MRA: Open Data, Weak Labels, and Anatomical Knowledge. Neuroinformatics. https://doi.org/10.1007/s12021-022-09597-0
- Peng, Y. et al. (2024). Deep learning and machine learning predictive models for neurological function after interventional embolization of intracranial aneurysms. Frontiers in Neurology, 15. https://doi.org/10.3389/fneur.2024.1321923
- Brutti, F., Fantazzini, A., Finotello, A. et al. Deep Learning to Automatically Segment and Analyze Abdominal Aortic Aneurysm from Computed Tomography Angiography. Cardiovasc Eng Tech 13, 535–547 (2022). https://doi.org/10.1007/s13239-021-00594-z
- Patel, T. R. et al. (2023). Evaluating a 3D deep learning pipeline for cerebral vessel and intracranial aneurysm segmentation from computed tomography angiography–digital subtraction angiography image pairs. Neurosurgical Focus, 54(6), E13. https://doi.org/10.3171/2023.3.focus2374
- Mohammad, M. et al. (2020). Predicting treatment outcome of intracranial aneurysms using angiographic parametric imaging and recurrent neural networks. Proc. SPIE. https://doi.org/10.1117/12.2548635
- Kim, T., On, S., Gwon, J.G. et al. Computed tomography-based automated measurement of abdominal aortic aneurysm using semantic segmentation with active learning. Sci Rep 14, 8924 (2024). https://doi.org/10.1038/s41598-024-59735-8
- Camara, J. R. et al. (2022). Development of a Convolutional Neural Network to Detect Abdominal Aortic Aneurysms. Journal of Vascular Surgery Cases, Innovations and Techniques. https://doi.org/10.1016/j.jvscit.2022.04.003
- Jing, L. et al. (2024). Determination of significant 3D hemodynamic features for post-embolization recanalization in cerebral aneurysms through explainable artificial intelligence. World Neurosurgery. https://doi.org/10.1016/j.wneu.2024.01.076
Disclaimer: The views expressed here are those of the author expressed in their private capacity and do not necessarily represent the views of AZoM.com Limited T/A AZoNetwork the owner and operator of this website. This disclaimer forms part of the Terms and conditions of use of this website.