By Ankit SinghReviewed by Susha Cheriyedath, M.Sc.Dec 8 2024
The combination of hyperspectral imaging (HSI) and artificial intelligence (AI) is enhancing environmental perception in robotics. This integration allows robots to perceive and analyze their surroundings with greater accuracy, supporting advancements in fields such as agriculture, disaster response, mining, and environmental monitoring.
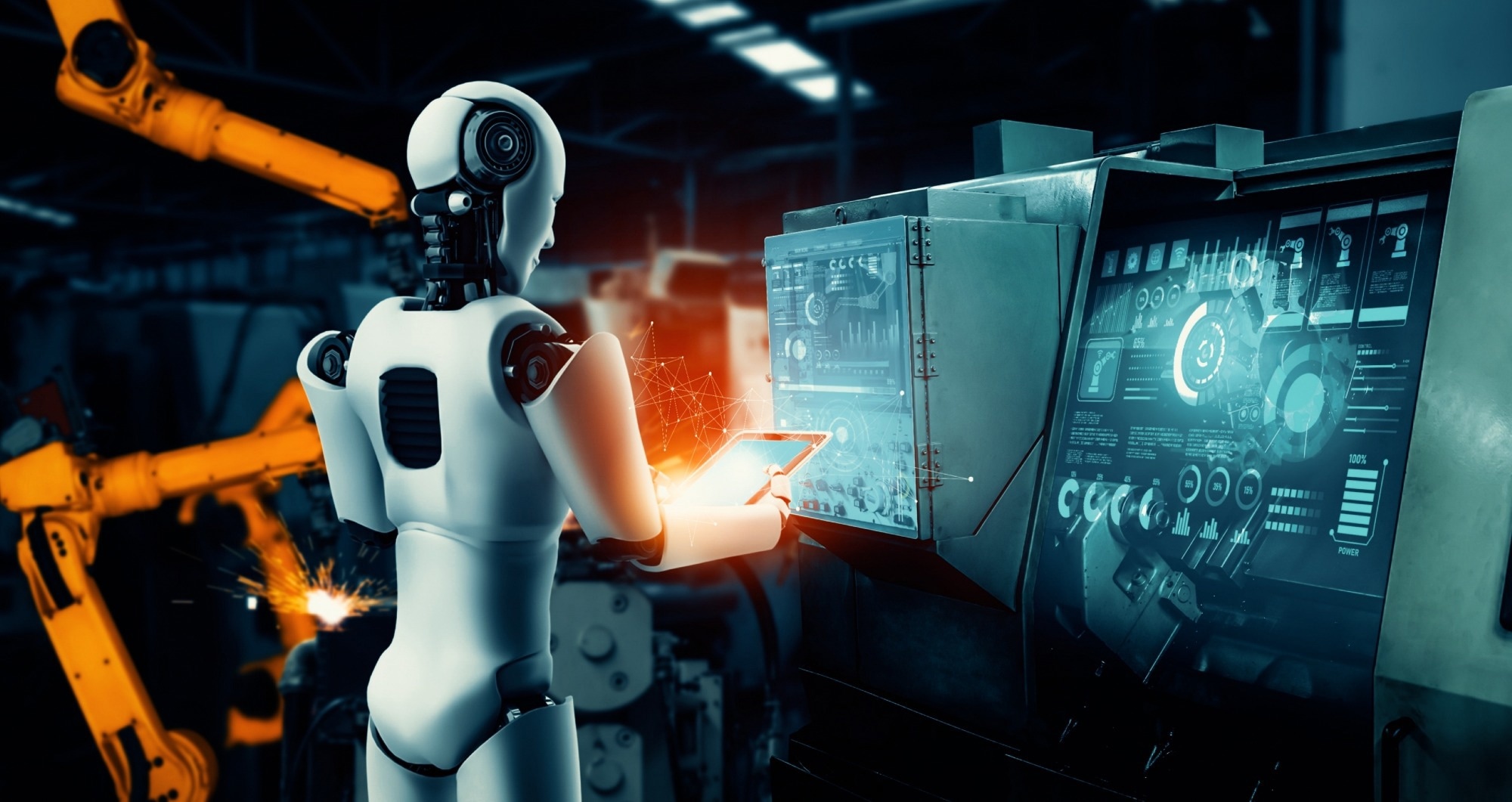
Image Credit: Summit Art Creations/Shutterstock.com
This article explores the technical aspects of how hyperspectral imaging and AI work together to enhance robotic perception and discusses related applications, challenges, and the latest developments in the field.
Understanding Hyperspectral Imaging
HIS involves capturing and analyzing light across a vast range of electromagnetic spectra, far beyond the visible spectrum. Unlike traditional imaging systems that use three color bands (red, green, and blue), HSI employs hundreds of narrow spectral bands. This capability allows the extraction of detailed spectral signatures from objects, making it possible to identify material composition, detect subtle variations, and monitor environmental changes.1
Key Features of HSI
- Spectral Resolution: High resolution in the spectral domain enables differentiation between objects with similar appearances but different compositions.1
- Material Identification: Unique spectral fingerprints allow precise identification of substances such as minerals, pollutants, or vegetation types.1
- Non-Invasive Monitoring: HSI offers real-time, non-invasive data collection, making it ideal for dynamic environments.1
The Role of Artificial Intelligence in Robotics
AI in robotics provides the computational power and algorithms required to interpret complex data streams and enable autonomous decision-making. Neural networks, machine learning, and deep learning models analyze and process environmental inputs to extract meaningful patterns and insights.1
Functions of AI in Environmental Perception
- Pattern Recognition: AI models detect and classify objects based on visual and spectral data.1,2
- Data Fusion: AI combines information from multiple sensors, including HSI, light detection and ranging (LiDAR), and radar, for a comprehensive understanding of the environment.1,2
- Predictive Analytics: Algorithms anticipate environmental changes, allowing robots to adapt proactively.1,2
From Data to Decisions: AI and Hyperspectral Imaging Combined
The synergy of hyperspectral imaging and AI transforms environmental perception by enabling robots to process vast, complex data streams and extract actionable insights.
Improved Spectral Analysis
Hyperspectral imaging provides detailed spectral information by capturing hundreds of narrow spectral bands. AI algorithms, such as convolutional neural networks (CNNs), analyze these data to extract meaningful patterns and identify subtle spectral variations. This capability allows robots to differentiate objects and materials that traditional imaging methods cannot distinguish.1,2
Dimensionality Reduction for Real-Time Processing
Hyperspectral data is inherently high-dimensional, often exceeding the processing capacity of conventional systems. AI employs techniques like principal component analysis (PCA) and autoencoders to reduce the dimensionality while preserving critical information. These methods enable real-time data processing, ensuring robots can respond promptly to dynamic environments.1,2
Enhanced Multimodal Fusion
AI facilitates the seamless integration of hyperspectral imaging data with inputs from other sensors, such as LiDAR and thermal cameras. This fusion creates a more holistic representation of the environment by combining spatial, spectral, and thermal data. Robots leveraging this multimodal approach achieve improved situational awareness and decision-making capabilities.1,2
Adaptive Decision-Making in Dynamic Environments
AI enables robots to dynamically adapt to environmental changes by analyzing hyperspectral inputs in real-time. Reinforcement learning algorithms allow systems to learn optimal actions based on past experiences and spectral data patterns. This adaptability is crucial for robots operating in unpredictable or hazardous settings, ensuring effective task execution.1,2
Real-World Transformations: Hyperspectral AI in Action
The combination of HSI and AI has unlocked diverse applications in fields like agriculture, mining, disaster response, and environmental monitoring. These technologies empower robots to analyze their surroundings with precision, supporting a wide range of tasks in both industrial and ecological domains.1,2
Agriculture
Robots equipped with hyperspectral imaging and AI analyze spectral data to assess crop health by detecting changes in chlorophyll levels and identifying stress indicators. These systems enable precision agriculture by optimizing irrigation, fertilization, and pest control strategies. The use of AI ensures real-time decision-making, helping to maximize crop yields while minimizing resource wastage.1,2
Environmental Monitoring
Robots leveraging HSI and AI monitor air, water, and soil quality by identifying pollutants and tracking their sources. The spectral sensitivity of hyperspectral imaging allows the detection of subtle environmental changes, such as harmful algal blooms or deforestation. AI enhances these capabilities by processing vast datasets to deliver actionable insights for conservation and remediation efforts.1,2
Mining and Resource Exploration
Hyperspectral imaging enables robots to map mineral distributions and evaluate ore quality by identifying spectral signatures unique to different materials. AI-powered systems process these spectral datasets to enhance the precision of resource exploration and extraction activities. This combination improves operational efficiency and reduces environmental impact by minimizing unnecessary excavation.1,2
Disaster Response
HSI and AI allow robots to detect hazardous materials, such as chemical spills, by analyzing their spectral properties. These systems assist in identifying structural damage or unsafe zones in disaster-affected areas. AI-driven models further optimize robotic navigation, enabling effective responses in time-sensitive and high-risk scenarios.1,2
Healthcare and Pharmaceuticals
In healthcare, HSI combined with AI assists in detecting abnormalities in tissues by analyzing spectral variations, providing enhanced accuracy for diagnostic imaging. This technology supports surgeons during operations by highlighting regions of concern, such as cancerous tissues, in real-time. In pharmaceuticals, AI-driven hyperspectral systems ensure quality control by identifying defects or impurities in products with unmatched precision and speed.1,2
Challenges and Limitations
Despite their potential, HSI and AI systems face challenges such as high data complexity, cost of equipment, scalability issues, and real-world adaptability. Addressing these hurdles requires advances in sensor technology, computational efficiency, and robust algorithm design.
- Data Complexity: Hyperspectral data is high-dimensional, requiring sophisticated algorithms and substantial computational power for analysis.1,2
- Cost of Equipment: Hyperspectral sensors and AI systems are expensive, limiting their accessibility for smaller organizations.1,2
- Scalability: Deploying these technologies at scale, such as monitoring vast agricultural fields, requires robust infrastructure and energy-efficient solutions.
- Interoperability: Integrating hyperspectral imaging with other sensor systems and ensuring compatibility can be technically challenging.1,2
- Real-World Generalization: AI models trained in controlled environments may struggle to adapt to unpredictable real-world conditions.1,2
Cutting-Edge Innovations in Hyperspectral AI
The field of hyperspectral imaging and AI is witnessing rapid progress, with research exploring ways to optimize performance and expand applications. A recent study published in Remote Sensing proposed an innovative hyperspectral object detection algorithm that improves detection accuracy by integrating spatial and spectral data.
The method introduces an edge-preserving dimensionality reduction (EPDR) module, which fuses spatial textures with spectral data, and a spatial feature enhancement module (SFEM) using CNN and Visual Mamba for enhanced perception. With linear complexity and superior accuracy compared to state-of-the-art methods, this approach advances real-time hyperspectral object detection capabilities.3
Another breakthrough study published in the Journal of Systems Architecture explored the use of hyperspectral imaging (HSI) cameras for advanced driver assistance systems (ADAS) to improve object detection and segmentation under challenging conditions, such as adverse weather and overlapping objects. By leveraging near-infrared spectral reflectance, the research developed a machine learning-based HSI segmentation system using the HSI-Drive 1.1 dataset.
To address implementation constraints and latency, the system was tested on embedded computing platforms, including graphics processing unit (GPU) system-on-a-chips (SoCs) and Field-Programmable Gate Array (FPGA)-enabled programmable SoCs. Results showed the FPGA-PSoC outperformed GPU-SoC in energy efficiency and processing latency, achieving industry-standard segmentation speeds, marking a significant advancement for reliable ADAS applications.4
Future Prospects and Conclusion
The synergy between hyperspectral imaging and AI holds immense potential for future robotics. Researchers are exploring advancements such as quantum-dot-based hyperspectral sensors and neuromorphic computing for energy-efficient AI processing. Further, collaboration between industry and academia is expected to accelerate the development of affordable, scalable solutions.1,2
Emerging applications in areas like autonomous vehicles, space exploration, and climate change mitigation are set to benefit significantly. For instance, robotic explorers equipped with HSI and AI could analyze extraterrestrial terrains to identify potential resources or signs of life.1,2
In conclusion, combining hyperspectral imaging and AI has transformed environmental perception in robots, enabling them to operate autonomously and effectively in complex, dynamic environments. This fusion not only enhances object recognition, material identification, and environmental mapping but also unlocks new possibilities in critical applications like agriculture, disaster response, and resource exploration. Despite challenges, continuous innovation promises to make these technologies more accessible and impactful, paving the way for a new era in robotics.
References and Further Reading
- Khonina, S. N. et al. (2024). Synergy between Artificial Intelligence and Hyperspectral Imagining—A Review. Technologies, 12(9), 163. DOI:10.3390/technologies12090163. https://www.mdpi.com/2227-7080/12/9/163
- Podlesnykh, I. et al. (2024). Towards the Future of Ubiquitous Hyperspectral Imaging: Innovations in Sensor Configurations and Cost Reduction for Widespread Applicability. Technologies, 12(11), 221. DOI:10.3390/technologies12110221. https://www.mdpi.com/2227-7080/12/11/221
- Li, W. et al. (2023). Hyperspectral Object Detection Based on Spatial–Spectral Fusion and Visual Mamba. Remote Sensing, 16(23), 4482. DOI:10.3390/rs16234482. https://www.mdpi.com/2072-4292/16/23/4482
- Gutiérrez-Zaballa, J. et al. (2023). On-chip hyperspectral image segmentation with fully convolutional networks for scene understanding in autonomous driving. Journal of Systems Architecture, 139, 102878. DOI:10.1016/j.sysarc.2023.102878. https://www.sciencedirect.com/science/article/pii/S1383762123000577
Disclaimer: The views expressed here are those of the author expressed in their private capacity and do not necessarily represent the views of AZoM.com Limited T/A AZoNetwork the owner and operator of this website. This disclaimer forms part of the Terms and conditions of use of this website.