Mar 17 2020
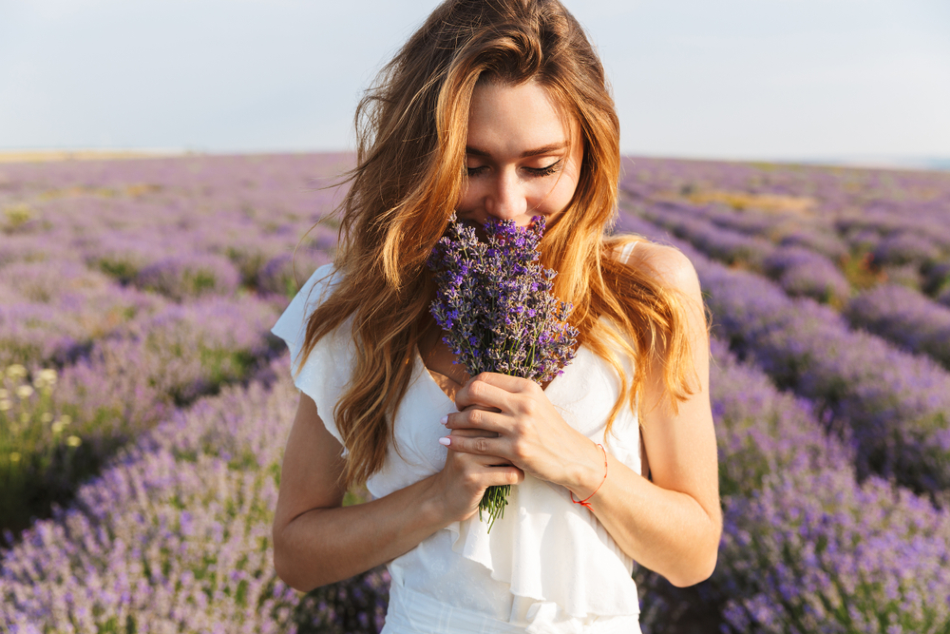
Image Credit: Dean Drobot/Shutterstock.com
When individuals smell an orange, the scent is probably mixed with a number of other smells from products such as soap, flowers, garbage, and car exhaust. Such smells adhere concurrently to an unlimited number of receptors that are present in the olfactory bulb of the human brain, obscuring each other.
Nevertheless, humans can still detect the smell of an orange, even when it is combined with a completely different pattern of other types of scents.
Researchers have long been puzzled by the exact mechanics of the way mammals learn and detect smells. Now, in a new study performed by Cornell University, a few of these functions have been explained with the help of a novel computer algorithm. This algorithm was inspired by the olfactory system present in mammals.
The computer algorithm provides a better understanding of the workings of the brain and, when applied to a computer chip, also learns patterns in a more reliable and rapid way when compared to prevalent machine learning models.
This is a result of over a decade of studying olfactory bulb circuitry in rodents and trying to figure out essentially how it works, with an eye towards things we know animals can do that our machines can’t.
Thomas Cleland, Study Senior Author and Professor of Psychology, Cornell University
The study is titled, “Rapid Learning and Robust Recall in a Neuromorphic Olfactory Circuit,” and published in the Nature Machine Intelligence journal on March 16th, 2020.
“We now know enough to make this work. We’ve built this computational model based on this circuitry, guided heavily by things we know about the biological systems’ connectivity and dynamics,” added Cleland. “Then we say, if this were so, this would work. And the interesting part is that it does work.”
Nabil Imam, Ph.D. ’14, the study’s co-author and a researcher at Intel, and Cleland used the computer algorithm on an Intel computer chip. Named Loihi, the research chip is neuromorphic, which means it was inspired by the way the brain works, and integrates digital circuits that imitate the way neurons learn and interact.
For instance, the Loihi chip is built on several cores that interact through discrete spikes, and the impacts relayed by all these spikes can modify based only on local activity. Such an architecture essentially needs different methods for algorithm design when compared to current computer chips.
With the help of neuromorphic computer chips, machines can potentially learn to detect patterns or carry out specific jobs a thousand times faster than by utilizing the central or graphics processing units of the computer, as done by a majority of the programs.
According to Intel, when certain algorithms are run on the Loihi research chip, this process uses only minimal power—that is, approximately a thousand times less than conventional techniques.
The Loihi research chip offers the ideal platform for the computer algorithm developed by Cleland. This algorithm can accept input patterns from a wide range of sensors, learn numerous patterns sequentially and quickly, and subsequently detect all these meaningful patterns in spite of the powerful sensory interference.
The computer algorithm can effectively detect odors even if their pattern is 80% dissimilar from the pattern learned by the computer originally.
The pattern of the signal has been substantially destroyed, and yet the system is able to recover it.
Thomas Cleland, Study Senior Author and Professor of Psychology, Cornell University
The brain of mammals is highly adept at detecting and recalling smells, with scores of hundreds or even thousands of complex neural networks and olfactory receptors quickly examining the patterns related to odors.
With regards to humans, the brains also retain what individuals have learned even after gaining new knowledge—something that is challenging for artificial intelligence systems but easy for mammals
Specifically, in deep learning methods, everything needs to be presented to the network simultaneously, or else, the latest information can destroy or distort the information learned by the system earlier. According to Cleland, this issue is solved by the brain-inspired computer algorithm.
“When you learn something, it permanently differentiates neurons,” Cleland stated. “When you learn one odor, the interneurons are trained to respond to particular configurations, so you get that segregation at the level of interneurons. So on the machine side, we just enhance that and draw a firm line.”
An earlier misunderstood phenomenon is explained by this concept: why the brain’s olfactory bulb is one of the few regions where new neurons are produced by mammals as soon as they reach adulthood.
The computational model turns into a biological hypothesis for why adult neurogenesis is important. Because it does this thing that otherwise would make the system not work. So in that sense, the model is feeding back into biology. And in this other sense, it’s the basis for a set of devices for artificial olfactory systems that can be constructed commercially.
Thomas Cleland, Study Senior Author and Professor of Psychology, Cornell University
This complexity of the brain-inspired Cleland to apply his neuroscience study around a theoretical method guided by distinct computational models.
“When you start studying a biological process that becomes more intricate and complex than you can just simply intuit, you have to discipline your mind with a computer model,” he added. “You can’t fuzz your way through it. And that led us to a number of new experimental approaches and ideas that we wouldn’t have come up with just by eyeballing it.”
The research was partially supported by the National Institute on Deafness and Other Communication Disorders, part of the National Institutes of Health.