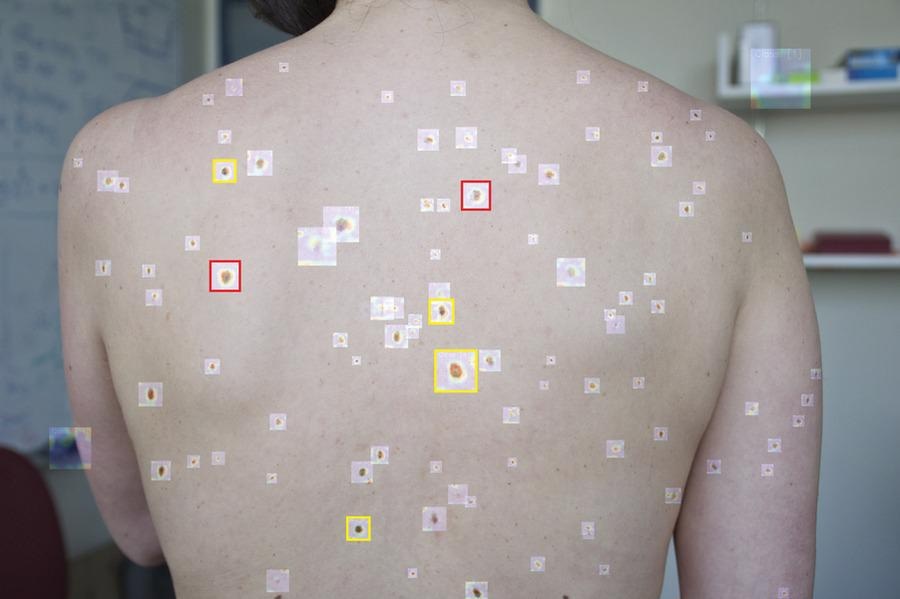
Image Credit: MIT
A newly developed system could use neural networks to spot 'ugly duckling' pre-cancerous lesions on a patient's skin, potentially detecting cancer more efficiently.
Melanoma is a type of malignant tumor that is responsible for 70% of all skin cancer deaths. Of the 96,480 people diagnosed with melanoma in the US during 2019, reports indicate that 7230 passed away, making it the deadliest form of skin cancer.
The key to preventing melanoma-related deaths is early identification. If doctors can spot the early cancer indicators of suspicious pigmented lesions (SPLs) quickly in primary-care settings, not only could this yield improved melanoma prognosis, but it could also result in a 20-fold reduction in treatment costs.
The problem is that currently, widespread screening isn't feasible. Returning to the US as an example, there are currently around 12 thousand practicing dermatologists. This means that each of them would have to see almost 30,000 patients per year to screen the entire population for SPLs.
Computer-aided diagnosis (CAD) systems currently exist that can analyze skin lesions to hunt for SPLs. Unfortunately, so far, CADs have failed to make a significant positive impact on melanoma diagnosis.
However, this could be about to change thanks to researchers from Wyss Institute for Biologically Inspired Engineering at Harvard University and the Massachusetts Institute of Technology (MIT).
The team, led by Luis R. Soenksen, a postdoc and a medical device expert, has developed an artificial intelligence (AI) system that uses deep convolutional neural networks (DCNNs) and wide-field photography to analyze SPLs.
Early detection of SPLs can save lives; however, the current capacity of medical systems to provide comprehensive skin screenings at scale is still lacking.
Luis R. Soenksen, Medical Device Expert
A study documenting the researchers' findings and the system they developed is documented in a recent edition of the journal Science Translational Medicine.
Using AI to Spot the 'Ugly Duckling'
One of the main reasons CAD systems have thus far failed to make a significant impact on the diagnosis of SPLs is thanks in large part to the fact they have been unable to replicate the system used to considerable effect by dermatologists. CAD systems' human counterparts compare lesions on a patient's body and analyze which could be cancerous — a so-called 'ugly duckling' criteria.
The team's new system can match this spotting method by analyzing photographs, ranking and distinguishing suspicious, potentially pre-cancerous skin lesions, which can turn into the deadly skin malignancy melanoma if not caught and removed early.
To test the system, the team applied it to almost 40 thousand images collected from 113 patients. The images were taken by commonly available commercial cameras, with the system able to use cameras common to smartphones, therefore eliminating the need for cumbersome medical imaging systems.
The system could spot SPLs and distinguish them from nonsuspicious lesions with a sensitivity and specificity of around 90%.
In a separate test, the results produced by the system were also compared to the assessment of three professional dermatologists with a good concordance between the human and machine-produced assessments.
Catching SPLs Early to Mitigate Skin Cancer Deaths
The ultimate aim of the team's AI is to produce a rapid and accurate SPL assessment, thus catching and treating melanomas early.
One of the most exciting factors is that getting rid of large cameras and complex medical equipment means that SPLs can be spotted in the GP's office or even in the patient's own home.
Our research suggests that systems leveraging computer vision and deep neural networks, quantifying such common signs, can achieve comparable accuracy to expert dermatologists. We hope our research revitalizes the desire to deliver more efficient dermatological screenings in primary care settings to drive adequate referrals.
Luis R. Soenksen, Medical Device Expert
The team will now aim to make the system work with a wider range of cameras, in different light settings, and with images taken by different photographers.
References
1. Soenksen. L. R., Kassis. T., Conover. S. T., et al, [2021], 'Using deep learning for dermatologist-level detection of suspicious pigmented skin lesions from wide-field images,' Science Translational Medicine, [https://stm.sciencemag.org/lookup/doi/10.1126/scitranslmed.abb3652]
Disclaimer: The views expressed here are those of the author expressed in their private capacity and do not necessarily represent the views of AZoM.com Limited T/A AZoNetwork the owner and operator of this website. This disclaimer forms part of the Terms and conditions of use of this website.