Each day advances are made in the automotive industry as modern automobiles are becoming increasingly technologically sophisticated. With computers built in to monitor emissions, maintain the engine and aid parking, today’s cars offer drivers unfettered access to information to optimize the car-owning experience.
Image Credit: Zewe, A., (2022) Anticipating others’ behavior on the road. [online] MIT News | Massachusetts Institute of Technology. Available at: https://news.mit.edu/2022/machine-learning-anticipating-behavior-cars-0421
However, one of the things really holding scientists back from fully autonomous driving is a vehicle’s ability to accurately predict human behavior.
To safely maneuver through today’s busy city streets, the computer of an automated vehicle would need to deal with numerous hazards such as crossing pedestrians, cyclists and human drivers. However, predicting exactly what a human is going to do next is not an easy task.
As it stands, current artificial intelligence (AI) systems are not fully up to the task to deal with unpredictable human behavior as they are fundamentally focused on a single task or behavior and, as a result, have issues with knowing how to react to sudden changes in direction such as a swerving cyclist or multiple road users at the same time.
Multiagent Behavior Solutions
Now, a team of MIT researchers has developed a solution that is surprisingly simple given the complexity of the issue at hand. The team was able to divide the problem of predicting multiagent behaviors into a series of smaller, more manageable pieces which the AI can solve in real-time.
The system works out the relationship between other road users, for example, calculating which driver or cyclist has the right of way in a given situation, and from this relationship can predict potential maneuvers offering a multiagent behavior solution.
During the research, which will be presented at the upcoming Computer Vision and Pattern Recognition Conference, the team established that this model demonstrated greater accuracy than other machine-learning programs.
The technique is also innovative in that it uses less memory than existing methods and even outperformed Waymo’s recently published model.
This is a very intuitive idea, but no one has fully explored it before, and it works quite well. The simplicity is definitely a plus.
Xin “Cyrus” Huang, Co-Lead Author
Huang added that the MIT model is also being compared with other state-of-the-art models in the field.
Including the one from Waymo, the leading company in this area, and our model achieves top performance on this challenging benchmark. This has a lot of potential for the future.
Xin “Cyrus” Huang, Co-Lead Author
M2I
The system, known as M2I, is able to take the information of multiple agents and determine the previous behaviors and relationships between these agents and consider who has the right of way (the passer) while the other is classified as the yielder. From there, the computer focuses on predicting the passer’s behavior as It behaves independently.
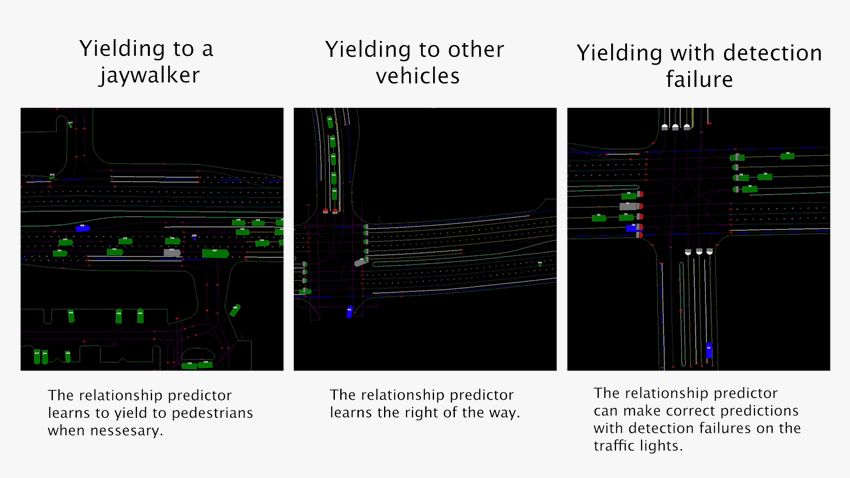
Image Credit: Zewe, A., (2022) Anticipating others’ behavior on the road. [online] MIT News | Massachusetts Institute of Technology. Available at: https://news.mit.edu/2022/machine-learning-anticipating-behavior-cars-0421
Once the M2I system has determined the possible maneuvers, it then uses an additional prediction model to predict the behaviors of the yielder. From the information gathered on the passer and the yielder, the computer then works out a series of ‘most-likely’ scenarios and selects six of those which are most likely to occur.
This model is then projected for a period of 8 seconds in advance, and the automated pilot system of the car is able to make accurate decisions based on an accurate behavioral model. During the trials, the M2I system slowed down to allow a pedestrian to cross the road and allow several cars to pass before making a turn.
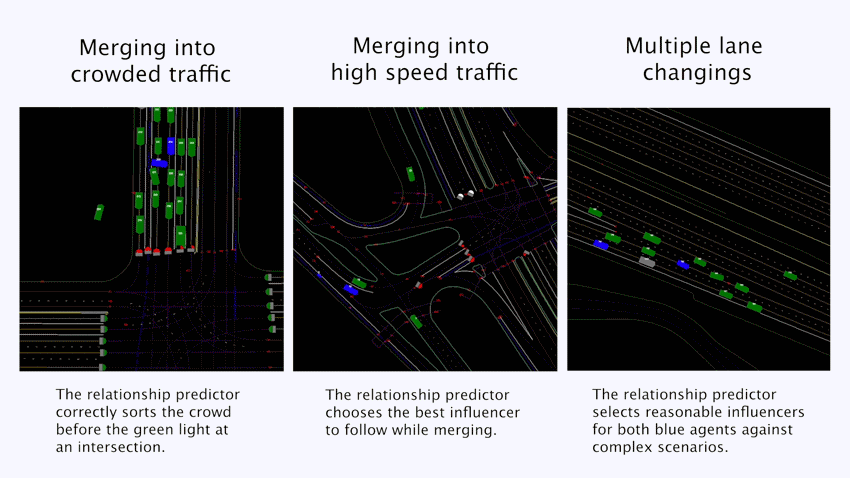
Image Credit: Zewe, A., (2022) Anticipating others’ behavior on the road. [online] MIT News | Massachusetts Institute of Technology. Available at: https://news.mit.edu/2022/machine-learning-anticipating-behavior-cars-0421
While currently focused on the relationship between two agents, the system could be tweaked to take into account many road users and then apply the same principles to make even more accurate decisions.
Training Self-Driving Cars
In order to train their system, the MIT team used a dataset containing millions of real traffic scenarios, which include multiple road users such as heavy traffic, cyclists, pedestrians, etc. The dataset is part of Waymo’s Open Motion Dataset and was captured using Lidar technology.
Rather than just building a more complex model to solve this problem, we took an approach that is more like how a human thinks when they reason about interactions with others. A human does not reason about all hundreds of combinations of future behaviors. We make decisions quite fast.
Xin “Cyrus” Huang, Co-Lead Author
The researchers plan to finetune the M2I system by addressing current limitations such as accounting for several participants and agents at the same time rather than the relationship between just two road users. This could make for better, safer self-driving vehicles when placed in real-world scenarios.
By simulating real-life interactions between multiple road users that could also be used to train future planning algorithms for autonomous self-driving cars, the system could also help create vast amounts of driving data to continually enhance performance in the long-term and, in turn, deliver a new generation of autonomous vehicles.
References and Further Reading
Zewe, A., (2022) Anticipating others’ behavior on the road. [online] MIT News | Massachusetts Institute of Technology. Available at: https://news.mit.edu/2022/machine-learning-anticipating-behavior-cars-0421
Disclaimer: The views expressed here are those of the author expressed in their private capacity and do not necessarily represent the views of AZoM.com Limited T/A AZoNetwork the owner and operator of this website. This disclaimer forms part of the Terms and conditions of use of this website.