Across the globe, coronary heart disease is the primary cause of adult death. The coronary angiography procedure offers the standard diagnostic assessment for almost all related clinical decision-making, ranging from medications to coronary bypass surgery.
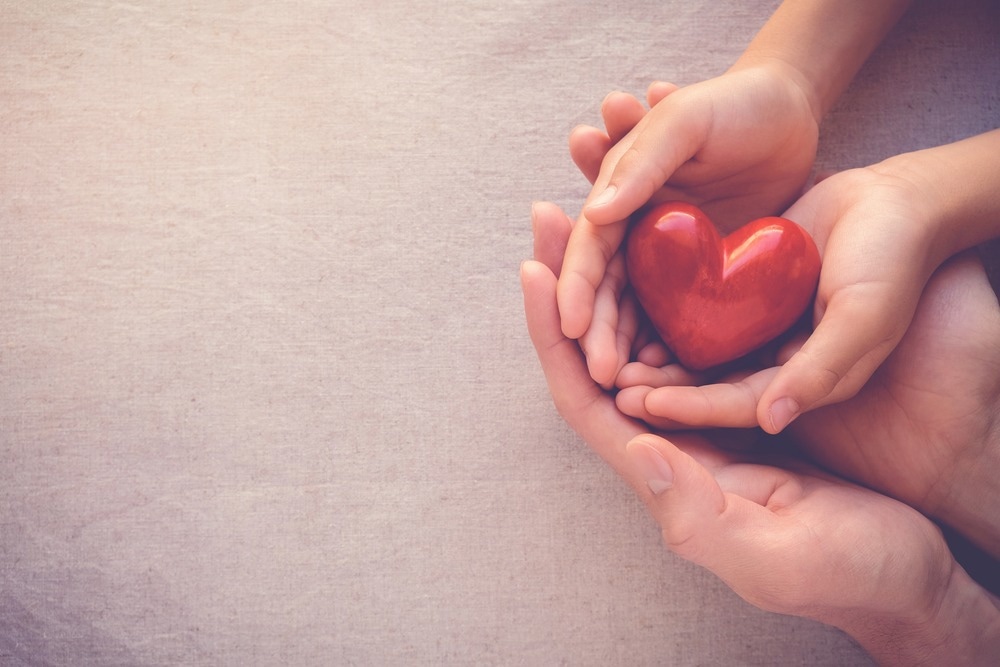
Image Credit: SewCream/Shutterstock.com
In several cases, measuring left ventricular ejection fraction (LVEF) at the time of coronary angiography is vital to improving clinical decision-making and treatment decisions, particularly when angiography is executed for possibly serious acute coronary syndromes (ACS).
Since the left ventricle is known to be the pumping center of the heart, quantifying the ejection fraction in the chamber offers vital information regarding the percentage of blood leaving the heart whenever it is subjected to contraction.
Quantifying LVEF during angiography requires an extra-invasive procedure known as left ventriculography. In this context, a catheter has been inserted into the left ventricle, and contrast dye is injected, which carries extra threats and increases the contrast exposure.
In a new study performed, senior author and University of California San Francisco Medical Center (UCSF) cardiologist Geoff Tison, MD, MPH, and first author Robert Avram, MD, of the Montreal Heart Institute, set out to identify if deep neural networks (DNNs), a category of AI algorithm, could be utilized to anticipate cardiac pump (contractile) function from standard angiogram videos.
A DNN known as CathEF was developed and tested to evaluate LVEF from coronary angiograms of the heart’s left side.
The study was reported in the JAMA Cardiology Journal on May 10th, 2023.
CathEF offers a novel approach that leverages data that is routinely collected during every angiogram to provide information that is not currently available to clinicians during angiography, effectively expanding the utility of medical data with AI and provides real-time LVEF information that informs clinical decision-making.
Geoff Tison, Associate Professor, Medicine and Cardiology, University of California San Francisco Medical Center
A cross-sectional study was performed by the scientists, which consisted of 4,042 adult angiograms paired with corresponding transthoracic echocardiograms (TTEs) ranging from 3,679 UCSF patients and trained a video-based neural network to evaluate the reduced LVEF (below or equal to 40%) and to anticipate (continuous) LVEF percentage from standard angiogram videos of the left coronary artery.
The study outcomes displayed that CathEF precisely anticipated LVEF, with powerful correlations to echocardiographic LVEF measurements and the standard noninvasive clinical approach. The model was validated externally in real-world angiograms from the Ottawa Heart Institute.
The algorithm executed well across various patient demographics and clinical conditions, such as acute coronary syndromes and altering levels of renal function—patient populations that might be less well-fitted to get the standard left ventriculogram procedure.
This study presents a novel method for assessing LVEF, an important measure of heart function, during any routine coronary angiography without requiring additional procedures or increasing cost. LVEF is essential for making decisions during the procedure and for managing patient care.
Robert Avram, Interventional Cardiologist and Former Research Fellow, University of California San Francisco Medical Center
Even though the algorithm was made to train on a large dataset of angiograms from UCSF and further individually verified in a dataset from the Ottawa Heart Institute, the investigators are undertaking additional research to test this algorithm at the point of care and identify its effect on the clinical workflow in patients who are suffering from heart attacks.
As a result, a multi-center prospective validation study in patients with ACS is in progress to compare the performance of CathEF and the left ventriculogram with TTEs executed within seven days of ACS.
This work demonstrates that AI technology has the potential to reduce the need for invasive testing and improve the diagnostic capabilities of cardiologists, ultimately improving patient outcomes and quality of life.
Geoff Tison, Associate Professor, Medicine and Cardiology, University of California San Francisco Medical Center
The study’s additional authors from UCSF include Joshua P. Barrios PhD, Sean Abreau MS, and Jeffrey E. Olgin MD.
This study was financially supported by US NIH grants K23HL135274 and U2CEB021881.
Journal Reference
Avram, R., et al. (2023) Automated Assessment of Cardiac Systolic Function From Coronary Angiograms With Video-Based Artificial Intelligence Algorithms. JAMA Cardiology. doi.org/10.1001/jamacardio.2023.0968.