Vision transformers (ViTs) are strong artificial intelligence (AI) technologies that have the potential to determine or classify objects in images.
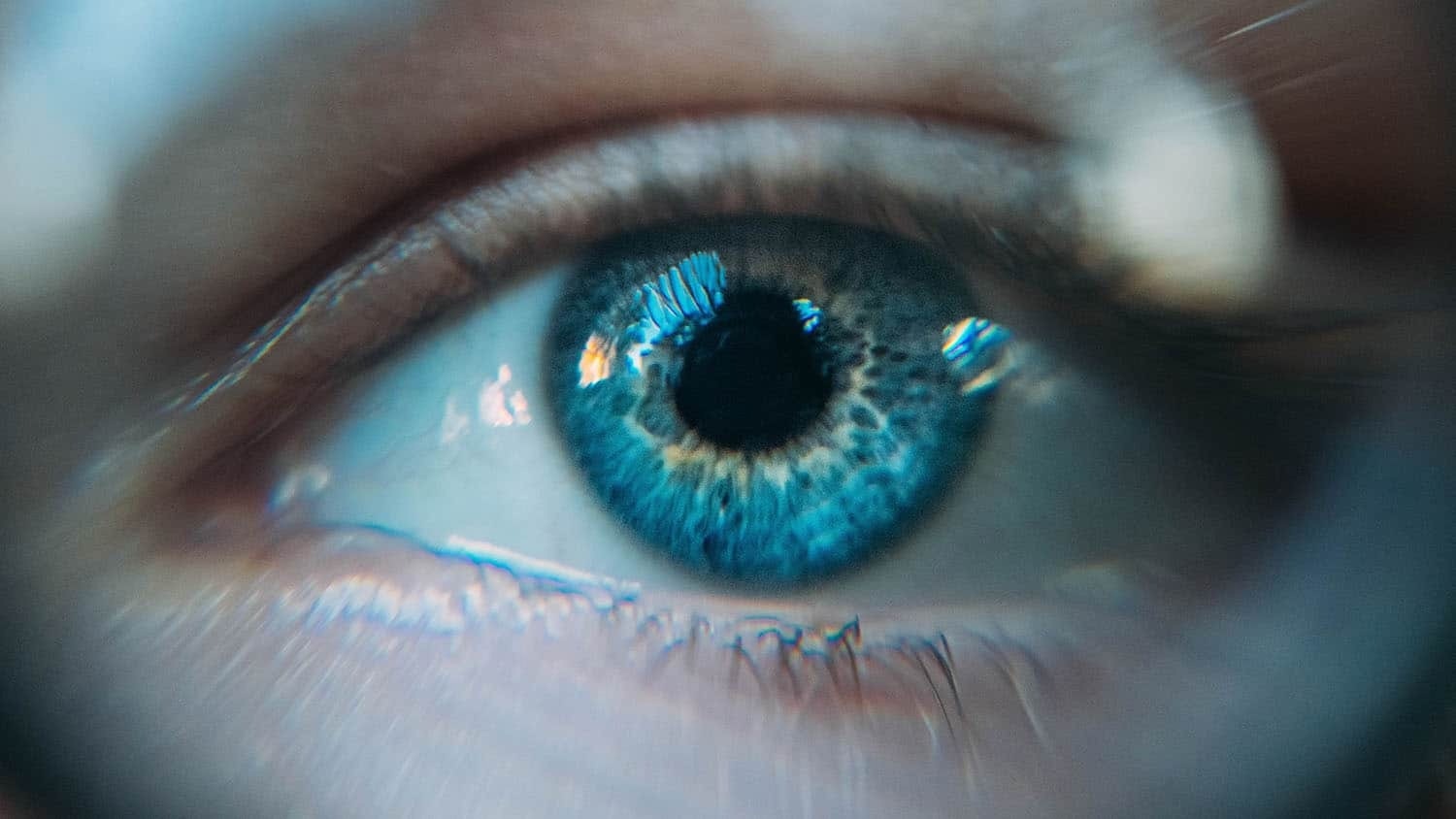
Image Credit: Ion Fet.
But there are considerable difficulties connected to both decision-making transparency and computing power needs. Scientists have come up with a new methodology that fulfills both challenges while also enhancing the potential of ViTs to determine, categorize, and segment objects in images.
Transformers are the most powerful AI models that are available to date. For instance, ChatGPT is an AI that makes use of transformer architecture, but the inputs utilized to train it are language. ViTs are known as transformer-based AI that is trained with the help of visual inputs. For example, ViTs can be utilized to detect and classify objects in an image, like determining all of the cars or all of the pedestrians in an image.
But ViTs encounter two challenges.
Transformer models are highly complicated. Transformer models need a significant amount of computational power and utilize a large amount of memory relative to the amount of data being plugged into the AI. This is especially tricky for ViTs since images consist of so much data.
Second, it is hard for users to comprehend precisely how ViTs make decisions. For instance, users may have trained a ViT to determine dogs in an image. However, it is yet to be clearly understood how the ViT is identifying what is a dog and what is not.
Based on the application, comprehending the ViT’s decision-making process, also called model interpretability, could be highly significant.
The new ViT methodology, known as “Patch-to-Cluster attention” (PaCa), helps fulfill both difficulties.
We address the challenge related to computational and memory demands by using clustering techniques, which allow the transformer architecture to better identify and focus on objects in an image.
Tianfu Wu, Study Corresponding Author and Associate Professor, Electrical and Computer Engineering, North Carolina State University
Wu added, “Clustering is when the AI lumps sections of the image together, based on similarities it finds in the image data. This significantly reduces computational demands on the system. Before clustering, computational demands for a ViT are quadratic. For example, if the system breaks an image down into 100 smaller units, it would need to compare all 100 units to each other—which would be 10,000 complex functions.
Wu continued, “By clustering, we’re able to make this a linear process, where each smaller unit only needs to be compared to a predetermined number of clusters. Let’s say you tell the system to establish 10 clusters; that would only be 1,000 complex functions.”
“Clustering also allows us to address model interpretability, because we can look at how it created the clusters in the first place. What features did it decide were important when lumping these sections of data together? And because the AI is only creating a small number of clusters, we can look at those pretty easily,” added Wu
The scientists performed extensive testing of PaCa, thereby making a comparison to two state-of-the-art ViTs known as PVT and SWin.
We found that PaCa outperformed SWin and PVT in every way. PaCa was better at classifying objects in images, better at identifying objects in images, and better at segmentation—essentially outlining the boundaries of objects in images.
Tianfu Wu, Study Corresponding Author and Associate Professor, Electrical and Computer Engineering, North Carolina State University
Wu added, “It was also more efficient, meaning that it was able to perform those tasks more quickly than the other ViTs. The next step for us is to scale up PaCa by training on larger, foundational data sets.”
The first author of the study is Ryan Grainger, a Ph.D. Student at NC State. The study was co-authored by Thomas Paniagua, a Ph.D. Student at NC State; Xi Song, an Independent Researcher; and Naresh Cuntoor and Mun Wai Lee of BlueHalo.
The study was done with financial support from the Office of the Director of National Intelligence, under contract number 2021-21040700003; the US Army Research Office, under grants W911NF1810295 and W911NF2210010; and the National Science Foundation, under grants 1909644, 1822477, 2024688 and 2013451.