Scientists were capable of simulating human behaviors with a probabilistic finite automaton—a renowned model of restricted computational power. They programmed the automatons to battle against each other in a wildlife poaching game, as either a rhino poacher or a ranger trying to prevent him.
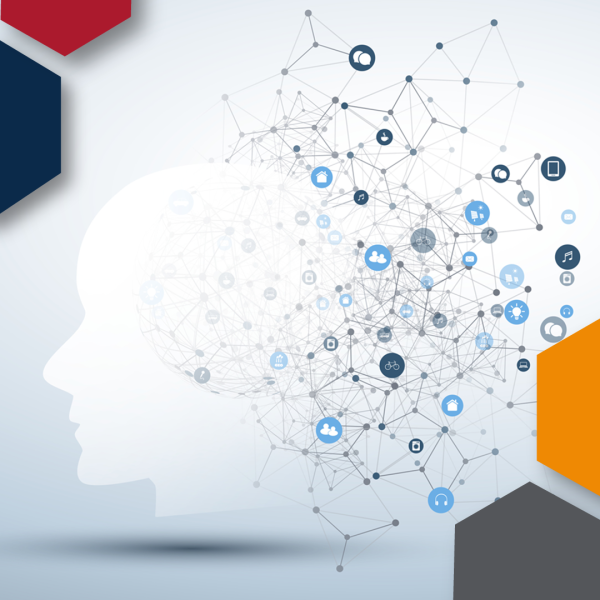
Image Credit: Cornell University
When the automatons could bear everything in mind, they settled into an optimal game strategy. However, when scientists restricted their memories, they took few decision-making shortcuts—the same kinds as actual humans playing the game.
The study outcomes indicate that human decisions might not be so irrational after all—taking the computational limitations into account.
This new work assists in the concept of bounded rationality.
Sometimes we do silly things or make systemic mistakes, not because we’re irrational but because we have limited resources. Oftentimes, we cannot remember everything that happened in the past or we don't have enough time to make a fully rational decision.
Xinming Liu, Study First Author, Cornell University
Liu presented the work, “Strategic Play By Resource-Bounded Agents in Security Games,” in May at the 2023 International Conference on Autonomous Agents and Multiagent Systems. The senior author is Joseph Halpern, Professor of Computer Science at the Cornell Ann S. Bowers College of Computing and Information Science.
As far as the poaching game is concerned, there are a limited number of sites, each with a different probability of including a rhino. In every round, the ranger and poacher selected a site to visit, thereby making their decisions depending on data from earlier rounds of the game. The poacher gets points by catching a rhino; the ranger gets points by catching the poacher.
If the ranger and poacher could recollect every move in the game, they soon settled into Nash equilibrium—a rational, unvarying pair of strategies.
However, if the automatons have highly restricted memory—so they cannot remember where precisely they saw that rhino 10, 100, or 1,000 rounds back—they begin to make irrational human-like decisions.
One human behavior the automatons reflected was probability matching. This happens when a person is guessing the outcomes of a coin toss when the coin has been weighted to heads three out of four times.
Rather than always guessing heads, which would provide a 75% success rate, several people would guess heads three-quarters of the time, which would reduce their success rate to around 63%.
In the game, this implies that the poacher made more visits to sites where they most frequently encountered rhinos in the past and fewer visits to sites that infrequently had a rhino. For the automatons, this strategy was not perfect, but it yielded decent outcomes.
One more irrational human behavior that resulted in good game performance was overweighting considerable outcomes—a phenomenon in which significant or traumatic incidents loom particularly large in the memory. For instance, a person may drive slowly down a stretch of road where they obtained a speeding ticket several years ago.
When the scientists programmed the poachers to overweight earlier encounters with the ranger, it paid off in the game. They ended up avoiding sites where the rangers were most likely to be.
To see how such outcomes match up to actual humans, Liu recruited nearly 100 people to play as the poacher on an online platform.
While some humans tended to select the same site every time or picked randomly to end the game and receive payment, others chose sites purely depending on probability matching. A third group supposed the ranger was probability-matching and visited sites accordingly to evade the ranger.
The parallels present in gameplay between the humans and automatons displayed that the model has the potential to recreate a minimum of two human behaviors, which, rather than being irrational, enhanced their performance.
Another way to interpret it is to say that you're doing the best you can given your computational limitations. And that strikes me as pretty damn rational.
Joseph Halpern, Professor of Computer Science, Ann S. Bowers College of Computing and Information Science, Cornell University