An innovative method of artificial intelligence (AI) allows for the reconstruction of a huge field of data, such as total ocean temperature, from a small number of field-deployable sensors utilizing low-powered “edge” computing, with extensive applications in industry, science, and medicine.
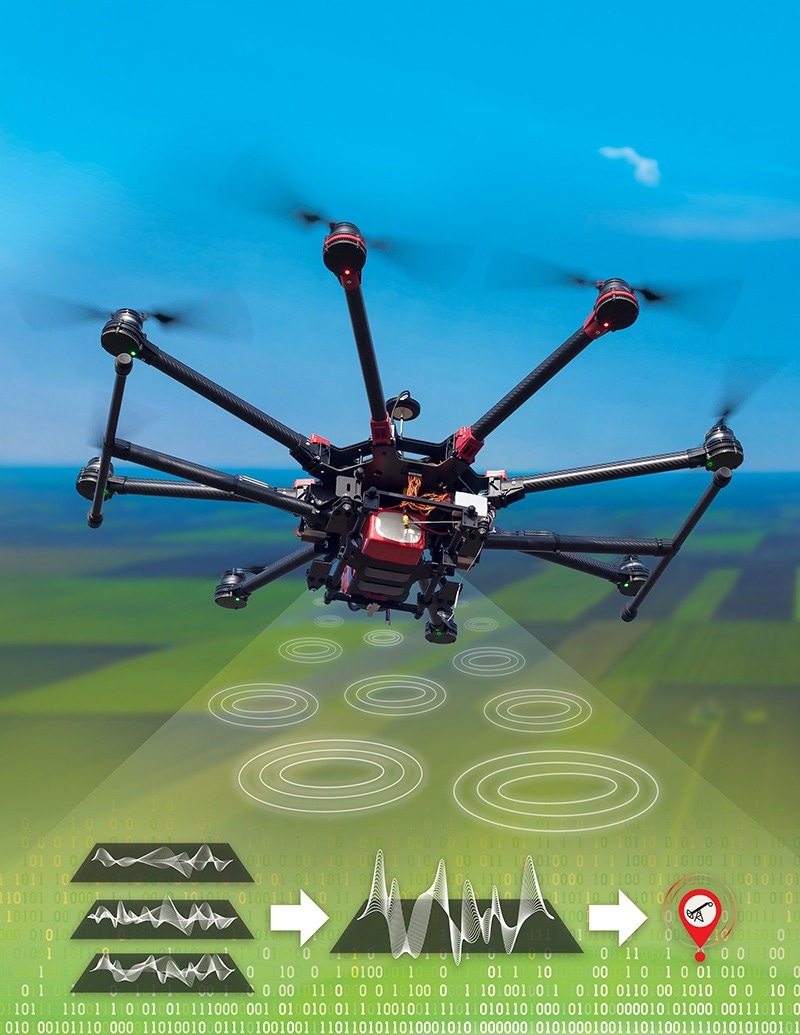
An innovation in using natural language models brings artificial intelligence to field deployable sensors, including drones. Los Alamos National Laboratory is exploring the AI technology for locating and characterizing orphaned oil and gas wells that emit climate-warming methane. Image Credit: Los Alamos National Laboratory
We developed a neural network that allows us to represent a large system in a very compact way. That compactness means it requires fewer computing resources compared to state-of-the-art convolutional neural network architectures, making it well-suited to field deployment on drones, sensor arrays and other edge-computing applications that put computation closer to its end use.
Javier Santos, Researcher, Los Alamos National Laboratory
Novel AI Approach Boosts Computing Efficiency
Santos is the first author of a study published in Nature Machine Intelligence by a team of Los Alamos researchers on the revolutionary AI technique known as Senseiver. The research, which is based on a Google AI model called Perceiver IO, combines natural-language model techniques such as ChatGPT to the problem of reconstructing knowledge about a large area — such as the ocean — from just a few measurements.
The researchers understood that due to the model’s efficiency, it would be widely applicable.
Using fewer parameters and less memory requires fewer central processing unit cycles on the computer, so it runs faster on smaller computers.
Dan O’Malley, Study Co-Author and Researcher, Los Alamos National Laboratory
Santos and his Los Alamos colleagues validated the model in a first for the published literature by showing its performance on complex three-dimensional fluids datasets and real-world sets of sparse data, or information gathered from sensors that cover only a small portion of the field of interest.
The group used a sea-surface temperature dataset from the National Oceanic and Atmospheric Administration to apply the model to and show off the practical uses of the Senseiver. The model successfully combined several readings from satellites and ship sensors that were collected over several decades. The model forecasted temperatures over the whole ocean based on these sparse point measurements, which gives global climate models valuable data.
Bringing AI to Drones and Sensor Networks
The Senseiver is a good fit for several of Los Alamos’s research initiatives and fields of interest.
Los Alamos has a wide range of remote sensing capabilities, but it’s not easy to use AI because models are too big and don’t fit on devices in the field, which leads us to edge computing. Our work brings the benefits of AI to drones, networks of field-based sensors and other applications currently beyond the reach of cutting-edge AI technology.
Hari Viswanathan, Study Co-Author and Fellow, Environmental Scientist, Los Alamos National Laboratory
The AI model will be especially helpful for the Lab's orphaned well identification and characterization tasks. The Lab is in charge of the federal program called Consortium Advancing Technology for Assessment of Lost Oil & Gas Wells (CATALOG), which is supported by the Department of Energy.
Its duties include identifying and evaluating wells that are illegally orphaned and quantifying the methane emissions they produce. Viswanathan is CATALOG’s lead scientist.
Large-scale, useful applications like self-driving cars, remote asset modeling in oil and gas, patient monitoring in healthcare, cloud gaming, content delivery, and contamination tracing can all benefit from the approach’s enhanced capabilities.
The Laboratory Directed Research and Development program at Los Alamos National Laboratory, the DOE Office of Science, Basic Energy Sciences, Fossil Energy and Carbon Management, Undocumented Orphan Wells program, and the Department of Energy’s Office of Science, Office of Basic Energy Sciences, Geoscience Research, provided funding for this work.
Journal Reference
Santos, J. E., et al. (2023) Development of the Senseiver for efficient field reconstruction from sparse observations. Nature Machine Intelligence. doi:10.1038/s42256-023-00746-x