Scripps Research scientists have developed an innovative artificial intelligence (AI) model aimed at enhancing clinicians’ ability to screen patients for atrial fibrillation (AFib), a condition characterized by an irregular, rapid heartbeat linked to stroke and heart failure.
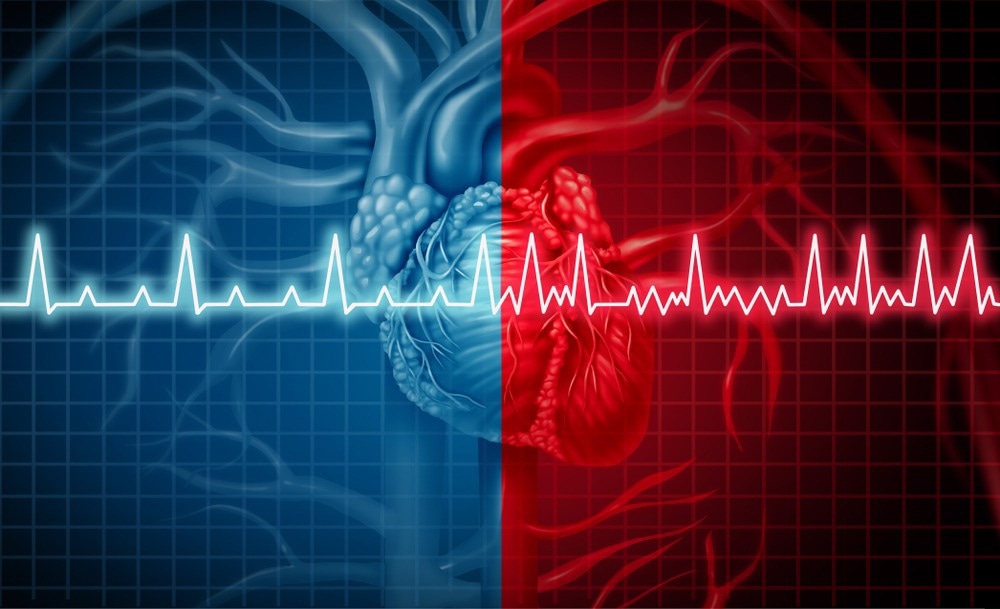
Image Credit: Lightspring/Shutterstock.com
Unlike standard screening tests, this model identifies subtle variations in an individual's normal heartbeat that indicate AFib risk.
The research, detailed in the December 12, 2023 issue of the journal npj Digital Medicine, utilized data from nearly half a million individuals who each wore an electrocardiogram (ECG) patch for a two-week period—a common screening method for AFib and other cardiac issues.
The AI model examined this extensive dataset to identify distinct patterns, beyond AFib itself, that differentiate individuals with AFib from those without. This breakthrough model has the potential to significantly improve the detection of individuals at risk for AFib, thereby helping to prevent the serious complications associated with this cardiac condition, such as stroke and heart failure.
With this new tool, we can better identify patients at high risk of AFib for further tests and interventions. Long term, this can help drive the right resources to the right people and potentially reduce the incidence of stroke and heart failure.
Giorgio Quer Ph.D., Study Senior Author and Director, Artificial Intelligence, Scripps Research Translational Institute
Giorgio Quer is also an Assistant Professor of Digital Medicine at Scripps Research.
The irregular heartbeat associated with AFib can lead to the accumulation of blood in the heart, forming clots that pose a risk of causing strokes. Additionally, AFib is linked to an elevated risk of heart failure or death.
To mitigate these complications in individuals with confirmed AFib, healthcare providers often prescribe anticoagulants—medications designed to prevent blood clot formation—alongside other lifestyle and medical interventions.
Diagnosing AFib can be challenging because some individuals with the condition experience only occasional episodes of irregular heartbeats or exhibit few symptoms. In certain cases, AFib manifests through symptoms like heart palpitations, lightheadedness, shortness of breath, and chest pain.
When patients present these symptoms, cardiologists typically conduct a detailed in-office recording of their heart rhythms using an electrocardiogram (ECG) equipped with 10 electrodes placed on the body, lasting around 10 seconds.
If no immediate irregularities are detected, healthcare professionals advise continued at-home monitoring for one or two weeks using a simpler wearable ECG patch featuring a single electrode. However, even within this two-week period, individuals with sporadic AFib may not have an episode captured by this device.
To address this challenge, Quer, in collaboration with iRhythm Technologies (the creator of the Zio®XT wearable electrocardiogram patch), embarked on a quest to identify alternative patterns in the ECG data of individuals with AFib.
We believe the electrical activity of the heart is slightly different for people who have AFib, but the differences are so subtle that cardiologists can’t look at a printout of heart rhythms and identify these differences.
Giorgio Quer Ph.D., Study Senior Author and Director, Artificial Intelligence, Scripps Research Translational Institute
The group created a machine learning model to scrutinize data gathered by iRhythm from 459,889 individuals who utilized the company's at-home ECG patch for a two-week period.
Extracting a day's worth of data from each ECG without any signs of AFib, the model demonstrated its capability to differentiate between individuals who eventually developed AFib and those who did not.
Even when the researchers incorporated all established AFib risk factors into their manually crafted models—encompassing demographic information and ECG metrics like variability between distinct heartbeats—the machine learning model surpassed them in accuracy when it came to predicting the risk of AFib.
“There was a gap between what we could achieve with any known ECG features and what the model could achieve. It was significantly better,” states Matteo Gadaleta, PhD, a Professional Scientific Collaborator at the Translational Institute and first author of the paper.
The model maintained accuracy across both an elderly population, with a heightened susceptibility to AFib, and individuals under 55 years old, who typically face a lower risk and are often excluded from routine AFib screening.
While the model is not designed for AFib diagnosis, it represents an initial stride toward creating a screening test for individuals at an elevated risk of AFib or those exhibiting symptoms.
This approach would enable them to wear an ECG patch for just one day to determine whether extended testing is warranted. Alternatively, the model could analyze one- or two-week ECG data to identify patients who, despite the absence of AFib during that period, should undergo a follow-up test.
Patients with frequent AFib episodes can be identified easily with an ECG recorded over at least a week. But this AI model could really help with people who have very infrequent AFib episodes yet could still benefit from diagnosis and intervention.
Giorgio Quer PhD, Study Senior Author and Director, Artificial Intelligence, Scripps Research Translational Institute
Quer and his collaborators aspire to orchestrate a prospective study, aiming to incorporate additional data sources—such as electronic medical records—into their models for further enhancement.
In addition to Quer and Gadaleta, contributors to the study encompass Steven R. Steinhubl from Scripps Research, along with Patrick Harrington, Eric Barnhill, Mintu Turakhia, and Evangelos Hytopoulos from iRhythm.
Financial support for this research was provided by the National Institute on Aging (R21AG072349) and the National Center for Advancing Translational Sciences (UL1TR002550) at the NIH.
New AI-powered algorithm could better assess people’s risk of common heart condition
New AI-powered algorithm could better assess people’s risk of common heart conditions. Video Credit: Scripps Research
Journal Reference
Gadaleta, M., et al. (2023) Prediction of atrial fibrillation from at-home single-lead ECG signals without arrhythmias. Npj Digital Medicine. doi.org/10.1038/s41746-023-00966-w
Source: https://www.scripps.edu/